CATN: Cross-Domain Recommendation for Cold-Start Users via Aspect Transfer Network
SIGIR '20: The 43rd International ACM SIGIR conference on research and development in Information Retrieval Virtual Event China July, 2020(2020)
摘要
In a large recommender system, the products (or items) could be in many different categories or domains. Given two relevant domains (e.g., Book and Movie), users may have interactions with items in one domain but not in the other domain. To the latter, these users are considered as cold-start users. How to effectively transfer users' preferences based on their interactions from one domain to the other relevant domain, is the key issue in cross-domain recommendation. Inspired by the advances made in review-based recommendation, we propose to model user preference transfer at aspect-level derived from reviews. To this end, we propose a cross-domain recommendation framework via aspect transfer network for cold-start users (named CATN). CATN is devised to extract multiple aspects for each user and each item from their review documents, and learn aspect correlations across domains with an attention mechanism. In addition, we further exploit auxiliary reviews from like-minded users to enhance a user's aspect representations. Then, an end-to-end optimization framework is utilized to strengthen the robustness of our model. On real-world datasets, the proposed CATN outperforms SOTA models significantly in terms of rating prediction accuracy. Further analysis shows that our model is able to reveal user aspect connections across domains at a fine level of granularity, making the recommendation explainable.
更多查看译文
关键词
Cold-Start Recommender Systems, Aspect-based Recommendation, Deep Learning
AI 理解论文
溯源树
样例
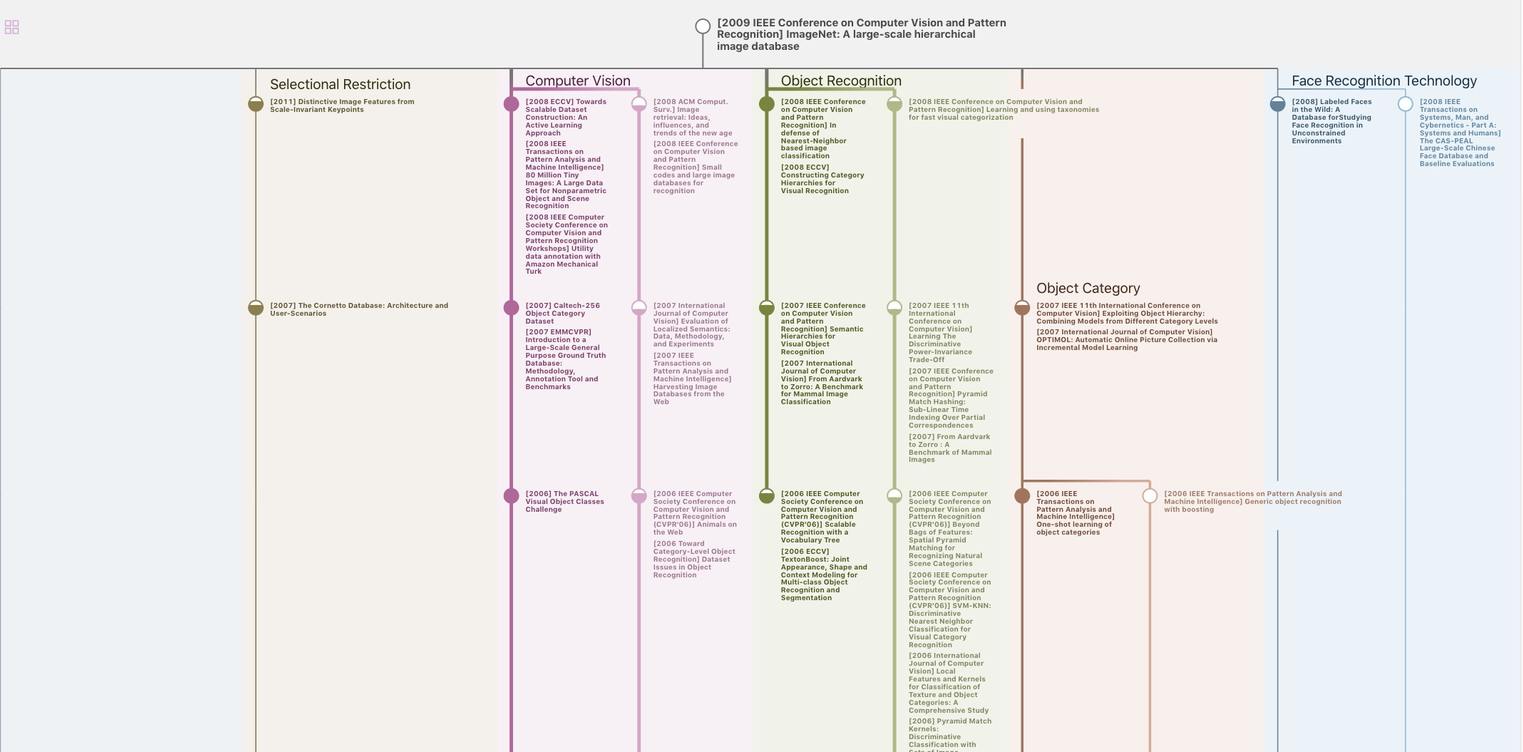
生成溯源树,研究论文发展脉络
Chat Paper
正在生成论文摘要