Sequential Recommendation with Self-Attentive Multi-Adversarial Network
SIGIR '20: The 43rd International ACM SIGIR conference on research and development in Information Retrieval Virtual Event China July, 2020(2020)
摘要
Recently, deep learning has made significant progress in the task of sequential recommendation. Existing neural sequential recommenders typically adopt a generative way trained with Maximum Likelihood Estimation (MLE). When context information (called factor) is involved, it is difficult to analyze when and how each individual factor would affect the final recommendation performance.
For this purpose, we take a new perspective and introduce adversarial learning to sequential recommendation. In this paper, we present a Multi-Factor Generative Adversarial Network (MFGAN) for explicitly modeling the effect of context information on sequential recommendation. Specifically, our proposed MFGAN has two kinds of modules: a Transformer-based generator taking user behavior sequences as input to recommend the possible next items, and multiple factor-specific discriminators to evaluate the generated sub-sequence from the perspectives of different factors. To learn the parameters, we adopt the classic policy gradient method, and utilize the reward signal of discriminators for guiding the learning of the generator. Our framework is flexible to incorporate multiple kinds of factor information, and is able to trace how each factor contributes to the recommendation decision over time. Extensive experiments conducted on three real-world datasets demonstrate the superiority of our proposed model over the state-of-the-art methods, in terms of effectiveness and interpretability.
更多查看译文
关键词
Sequential Recommendation, Adversarial Training, Self-Attentive Mechanism
AI 理解论文
溯源树
样例
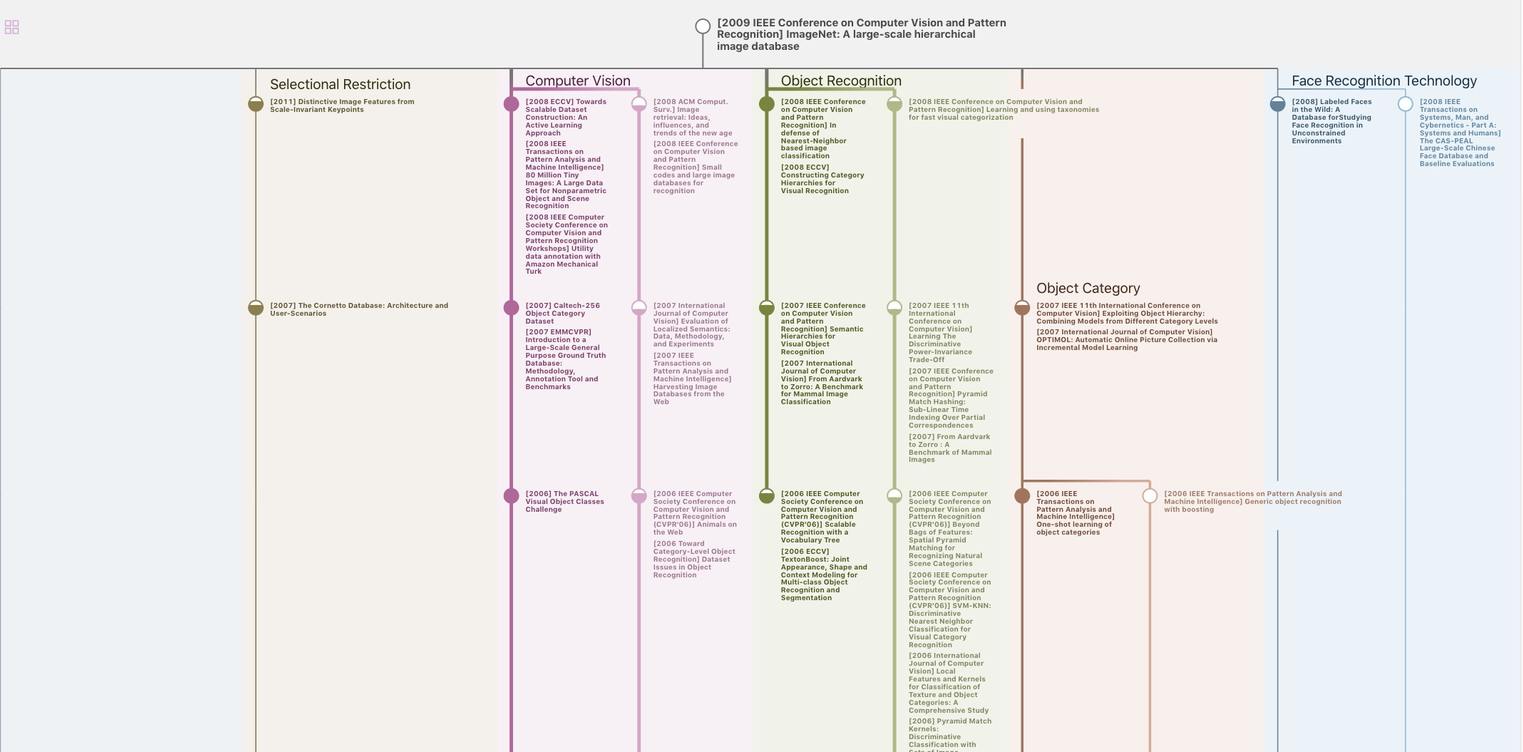
生成溯源树,研究论文发展脉络
Chat Paper
正在生成论文摘要