Crafting and Detecting Adversarial Web Requests
2019 IEEE International Conference on Smart Cloud (SmartCloud)(2019)
摘要
Deep learning (DL) techniques have provided state-of-the-art results for many machine learning tasks. In response to the increasing demand for web security, many researchers have been focusing on applying DL to detect web attacks. However, these works just pay attention to the detection accuracy, not the robustness of the detection model itself. In this paper, we proved that it is possible to generate adversarial web requests by modifying only a few characters of them, which can lead the existing DL based model to wrong predictions. The attackers may take this vulnerability to trigger false positive alarms or even disable the whole detection model. As the defensive measure, we propose to use a combined method of kernel density estimation and model uncertainty estimation to detect these adversaries. Through experiment, we report a ROC-AUC of over 95% of detecting these adversarial web requests.
更多查看译文
关键词
Web Attack,Adversarial Attack,Deep Neural Network,Model Uncertainty,Density Estimation
AI 理解论文
溯源树
样例
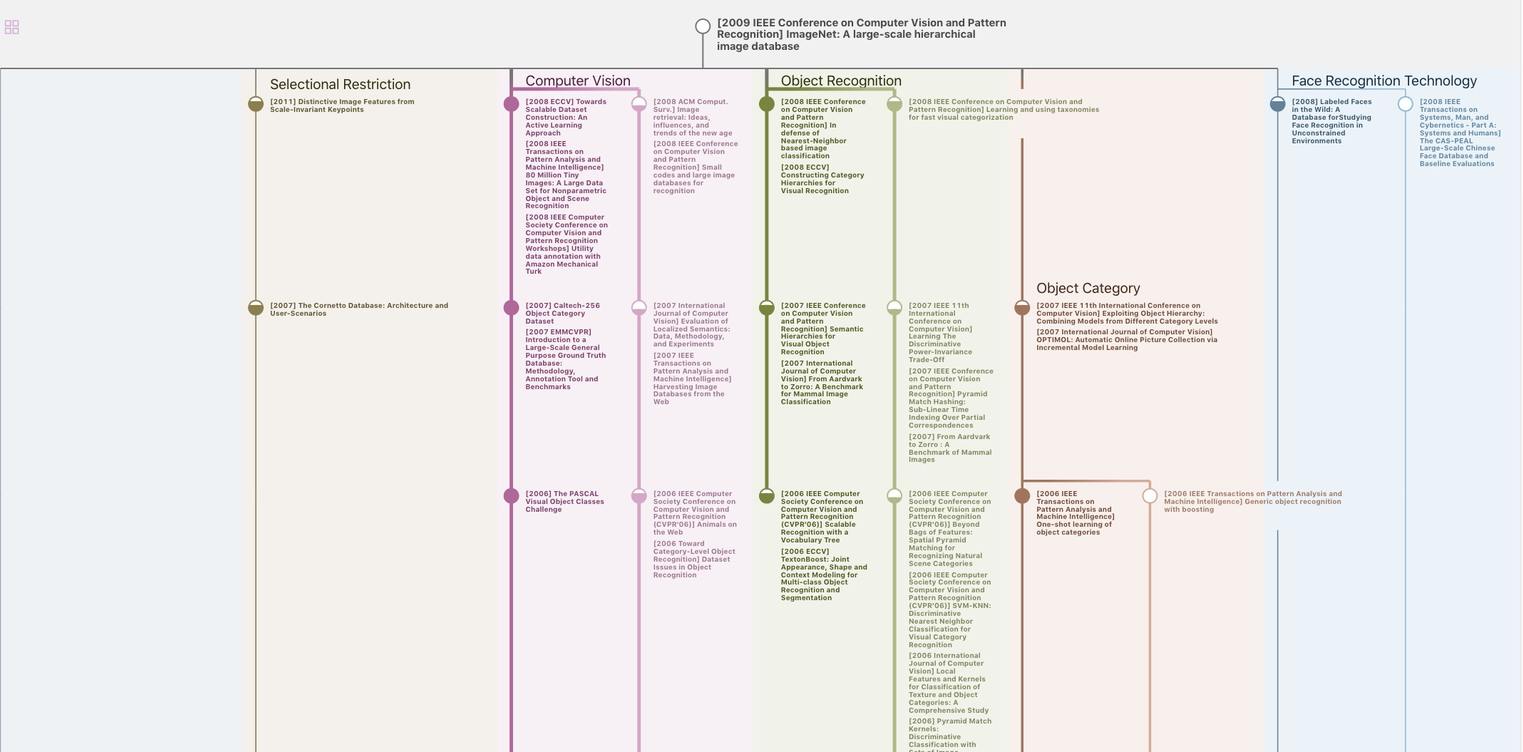
生成溯源树,研究论文发展脉络
Chat Paper
正在生成论文摘要