Learned Resolution Scaling Powered Gaming-as-a-Service at Scale
IEEE Transactions on Multimedia(2021)
摘要
Built on the explosive advancement of cloud and telecommunication technologies, Gaming-as-a-Service (GaaS) or cloud gaming system is expected to revolutionize the traditional multi-billion video game market in the near future. This wave is analogous to the rise of live-video-streaming-based-Netflix to replace conventional DVD rental business for movies and TVs. In practice, a successful GaaS platform need to operate in a transparent mode without requiring substantial efforts from both content providers and end users, and offer the pristine quality of experience (QoE) at an affordable cost. Our analysis suggests that GaaS provisioning cost can be reduced significantly by enforcing the game video rendering and streaming at a lower resolution (so as to increase the user concurrency in the cloud and reduce the streaming bandwidth over the network). However, streaming video at a lower resolution may deteriorate the QoE. To maintain the client QoE at the level using the default-native resolution for streaming or even enhance it, we introduce the learned resolution scaling (LRS), which leverages the computational capabilities at clients/edges to restore/improve the reconstructed image/video quality via stacked deep neural networks (DNN). We integrate this LRS into a commercialized GaaS platform - AnyGame, to study its efficiency and complexity quantitatively. Extensive real-life experiments have shown that LRS-powered AnyGame offers the state-of-the-art performance, and the lower operational cost, paving the road for a potential success of GaaS over the Internet. Additionally, we dive into proposed LRS via ablation studies to further demonstrate its consistent performance, including the discussions on trade-off between efficiency and complexity, alternative training sets, etc.
更多查看译文
关键词
Gaming-as-a-Service,compressed video strea-ming,learned resolution scaling
AI 理解论文
溯源树
样例
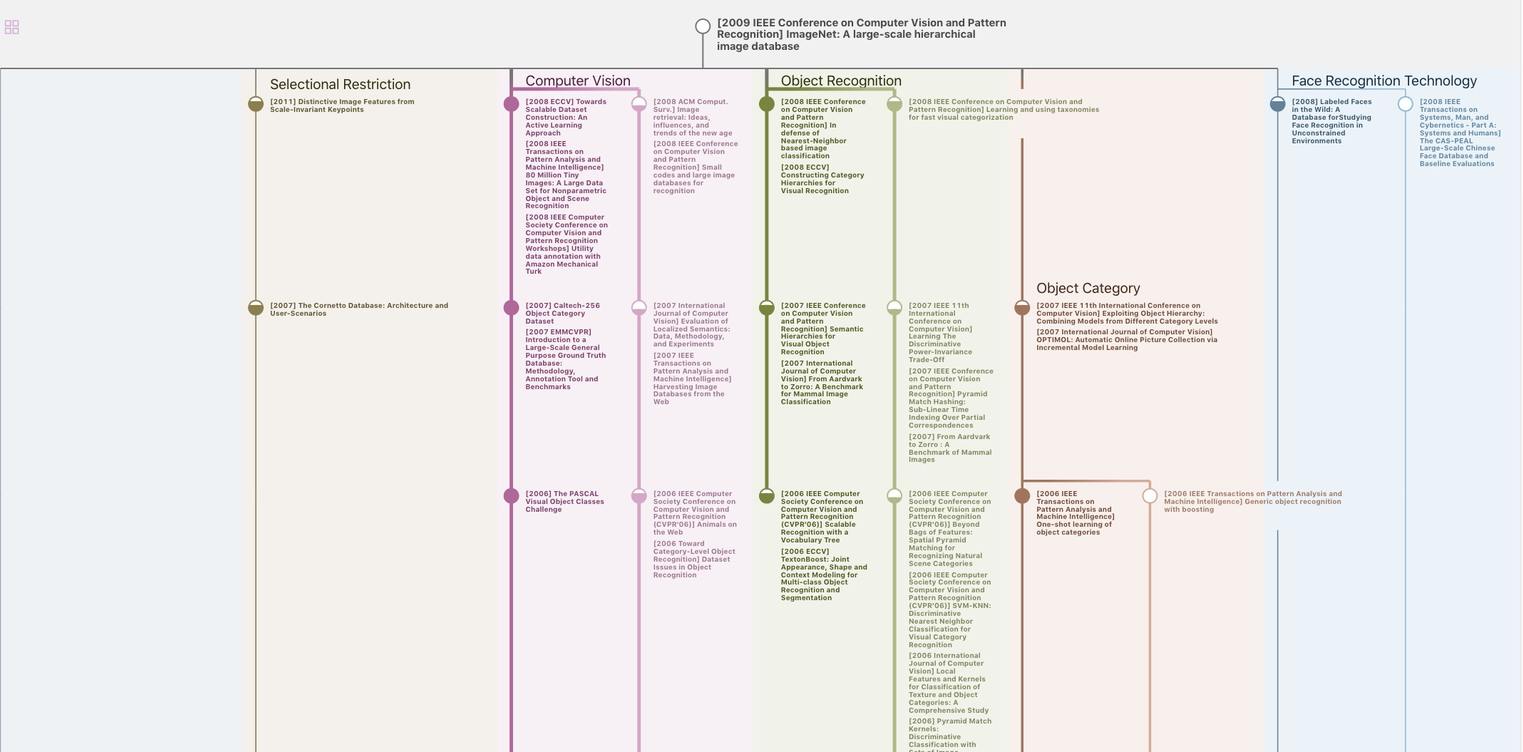
生成溯源树,研究论文发展脉络
Chat Paper
正在生成论文摘要