Detecting Autism Spectrum Disorder Using Topological Data Analysis
2020 IEEE INTERNATIONAL CONFERENCE ON ACOUSTICS, SPEECH, AND SIGNAL PROCESSING(2020)
摘要
Autism Spectrum Disorder (ASD) is a neurological developmental disorder affecting children at an early age. ASD diagnosis remains a challenge and is carried out using behavorial and developmental screening currently. Here we show the efficacy of using single trial EEG as a potential neural marker for ASD detection. We used EEG signals of a group of children participating in a visual cognitive task where images of emotional faces and trees were displayed. We used a feature extraction technique based on Topological Data Analysis (TDA) to classify autistic subjects from typically developing ones. The high accuracy ( 90%) of the pattern classifier validates the efficacy of using topological features in ASD detection. Our results also showed that ASD detection can be achieved as early as 120 ms post stimulus display and is independent of face/non-face images displayed.
更多查看译文
关键词
Topological Data Analysis, Persistent Homology, Persistent Entropy, Pattern Classifier, Autism Spectrum Disorder
AI 理解论文
溯源树
样例
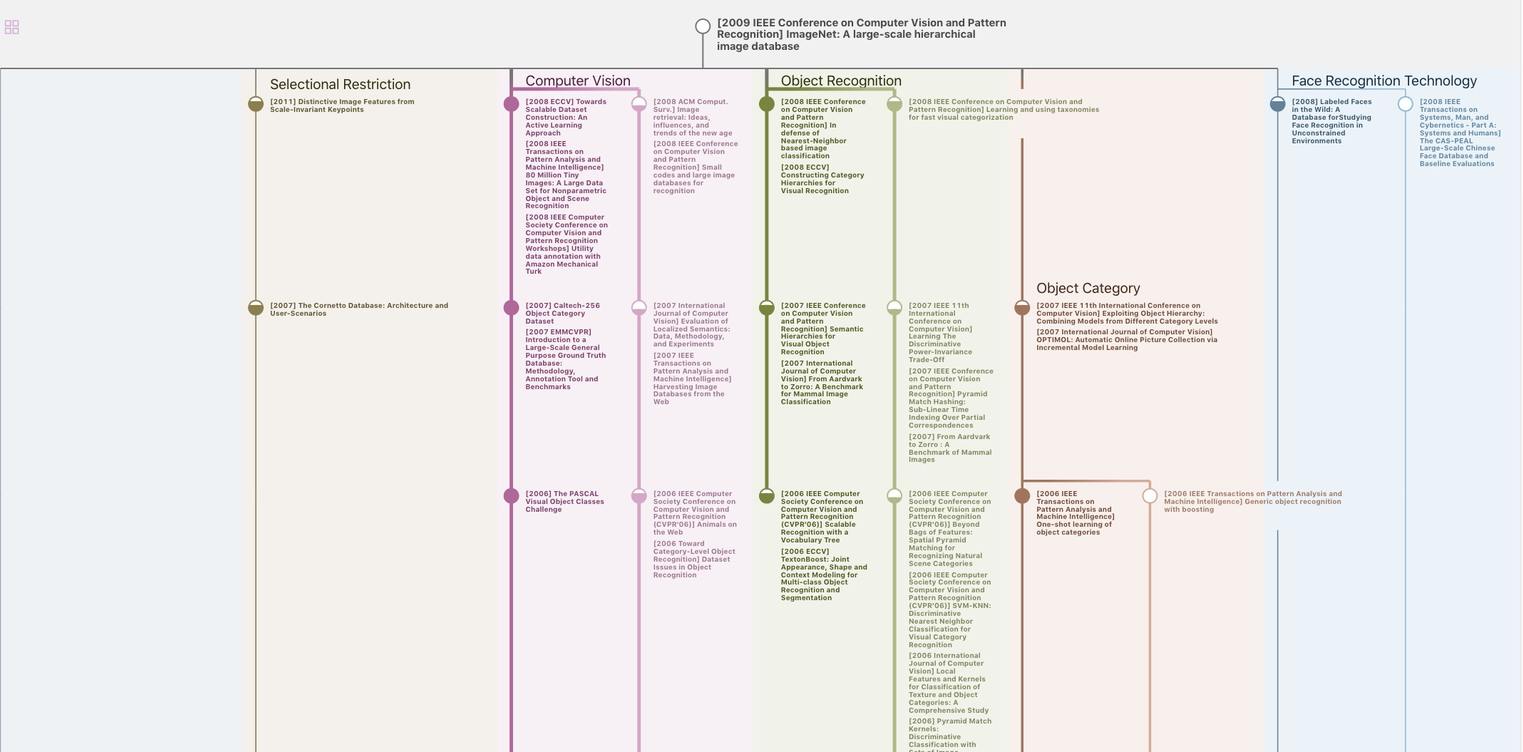
生成溯源树,研究论文发展脉络
Chat Paper
正在生成论文摘要