Deriving Compact Feature Representations Via Annealed Contraction
2020 IEEE INTERNATIONAL CONFERENCE ON ACOUSTICS, SPEECH, AND SIGNAL PROCESSING(2020)
摘要
It is common practice to use pretrained image recognition models to compute feature representations for visual data. The size of the feature representations can have a noticeable impact on the complexity of the models that use these representations, and by extension on their deployablity and scalability. Therefore it would be beneficial to have compact visual representations that carry as much information as their high-dimensional counterparts. To this end we propose a technique that shrinks a layer by an iterative process in which neurons are removed from the and network is fine tuned. Using this technique we are able to remove 99% of the neurons from the penultimate layer of AlexNet and VGG16, while suffering less than 5% drop in accuracy on CIFAR10, Caltech101 and Caltech256. We also show that our method can reduce the size of AlexNet by 95% while only suffering a 4% reduction in accuracy on Caltech101.
更多查看译文
关键词
model compression, compact representations, distillation
AI 理解论文
溯源树
样例
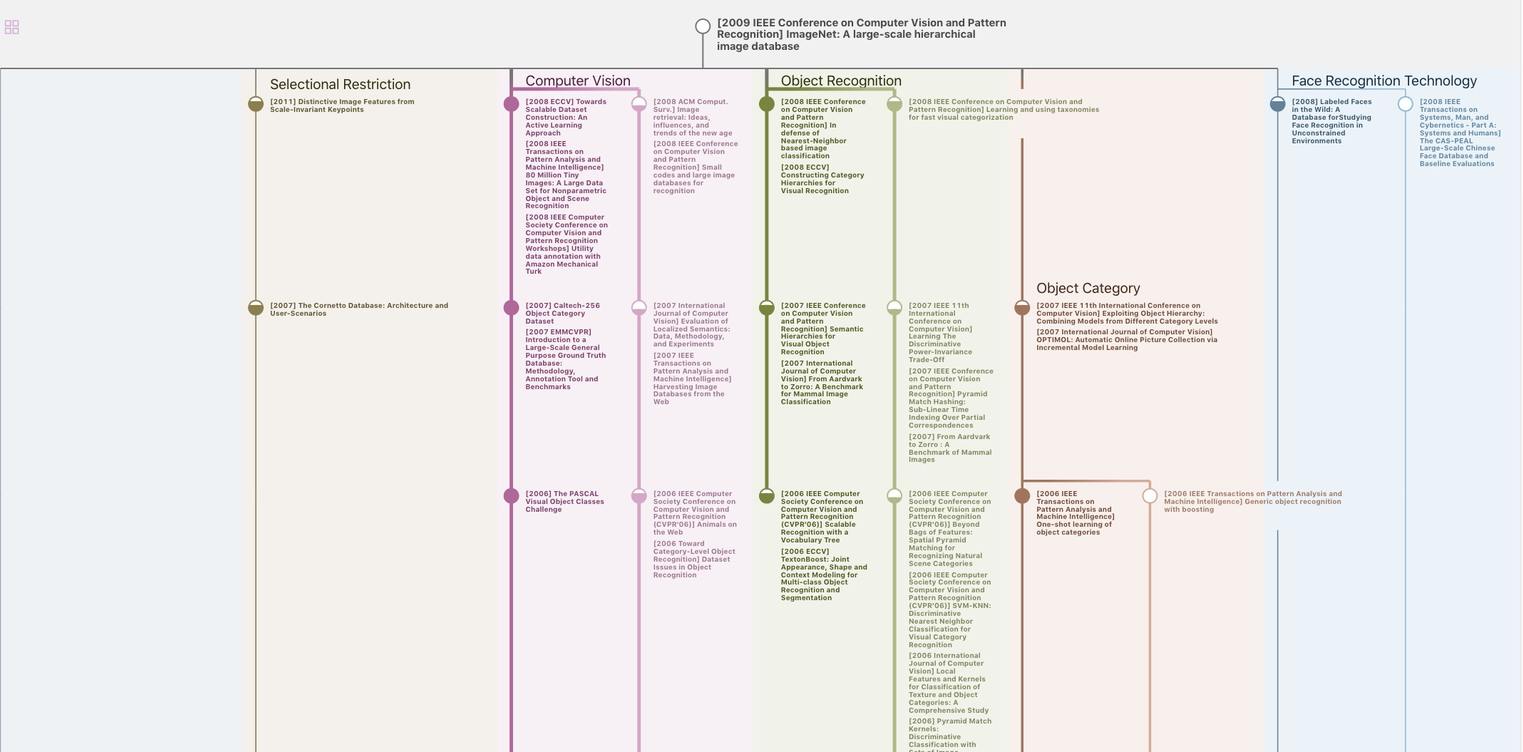
生成溯源树,研究论文发展脉络
Chat Paper
正在生成论文摘要