Progressive Multi-Target Network Based Speech Enhancement With Snr-Preselection For Robust Speaker Diarization
2020 IEEE INTERNATIONAL CONFERENCE ON ACOUSTICS, SPEECH, AND SIGNAL PROCESSING(2020)
摘要
In this paper, we design a novel front-end processing system for speaker diarization under realistic conditions with challenging background noises. To cope with diversified environments, we first extend our perviously proposed progressive learning based speech enhancement model by adding multi-task learning in each intermediate layer. The corresponding progressive multi-target (PMT) in various layers includes both progressive ratio mask (PRM) and progressively enhanced log-power spectra (PELPS) with specified signal-to-noise-ratios (SNRs). Speech distortions are commonly introduced during the front-end processing, which often deteriorate the back-end performance. However, the proposed speech enhancement model can be regarded as a bagging of models with multiple learning objectives, which provides flexibility for selecting the most appropriate output for robust speaker diarzation. In addition, a global SNR estimation is performed using the results of deep neural network (DNN) based speech activity detection (SAD) to decide whether the audio should be enhanced. We evaluate the speaker diarzation performance on the second DIHARD dataset which includes several different realistic conditions. Compared with the original data, experiments demonstrate that the enhanced data processed by our proposed method can effectively avoid the performance loss of every single domain, and achieve consistent improvements in most domains.
更多查看译文
关键词
Speech enhancement, speaker diarization, speech activity detection, DIHARD data, SNR estimation
AI 理解论文
溯源树
样例
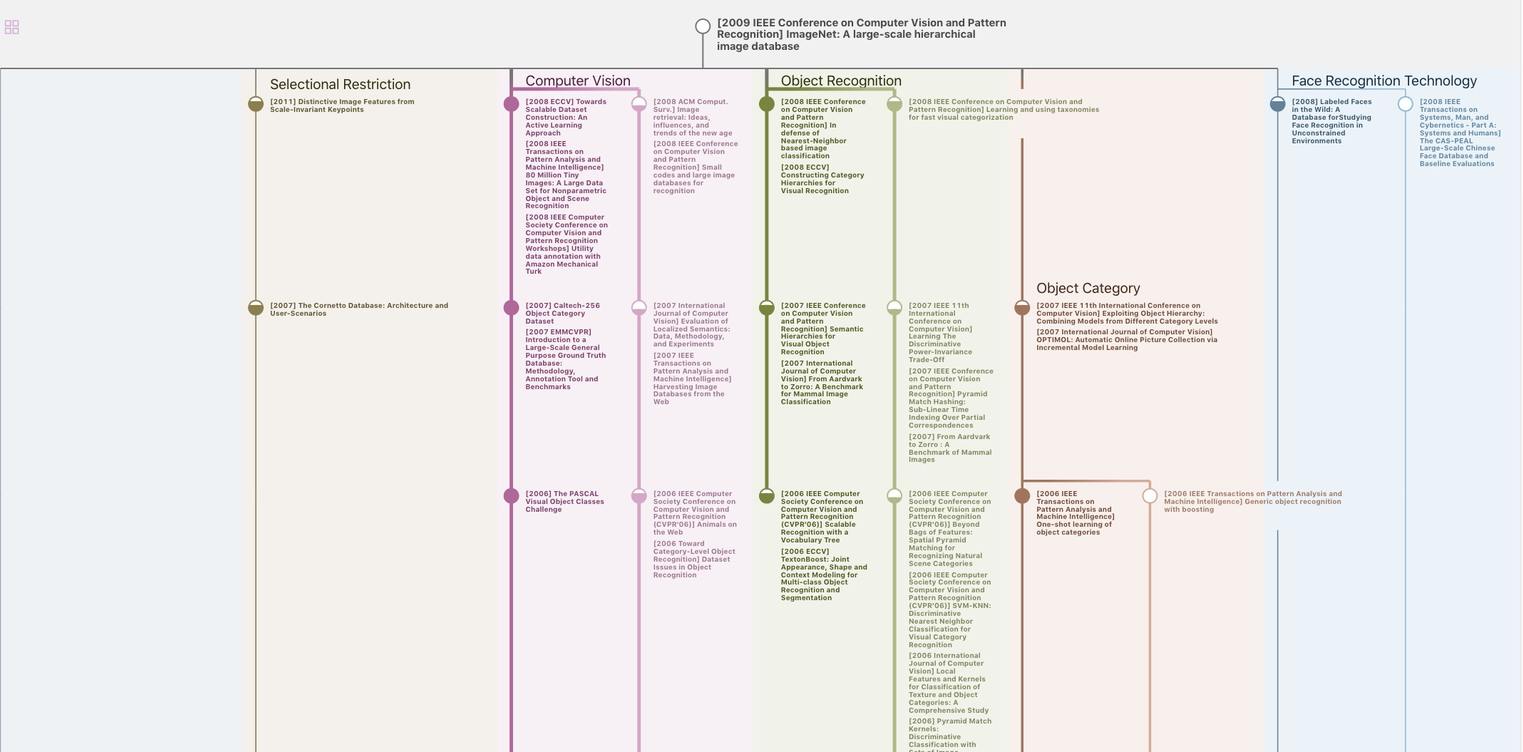
生成溯源树,研究论文发展脉络
Chat Paper
正在生成论文摘要