Evolving SARIMA Models Using cGA for Time Series Forecasting
2019 IEEE International Autumn Meeting on Power, Electronics and Computing (ROPEC)(2019)
摘要
This article presents a methodology to automate the production of forecasting models. The kind of models we produce is Seasonal ARIMA. The search for the best model structure is performed using the compact Genetic Algorithm, which uses a binary codification of the individuals. An important feature of compact Genetic Algorithms is the lack of an explicit representation of the population, which is replaced by an estimation of the probability distribution of each bit of the binary encoding of chromosomes in the population. The proposed methodology was tested with 7 time series from different application fields. The results were satisfactory; given a time series, the proposed methodology produces a SARIMA-based forecasting model, capable of capturing the time series main features. In all cases, the models were able to capture seasonality, and in the case of the air passengers data set, the resulting model captured the trend (i.e., non-stationarity) component of the data. As an extension of this work more extensive evolutionary processes will be executed (tuning their metaparameters), aiming to produce models that reduce forecasting errors.
更多查看译文
关键词
probability distribution,different application fields,SARIMA-based forecasting model,time series main features,evolving SARIMA models,time series forecasting,forecasting models,Seasonal ARIMA,model structure,compact Genetic Algorithm,binary codification,explicit representation
AI 理解论文
溯源树
样例
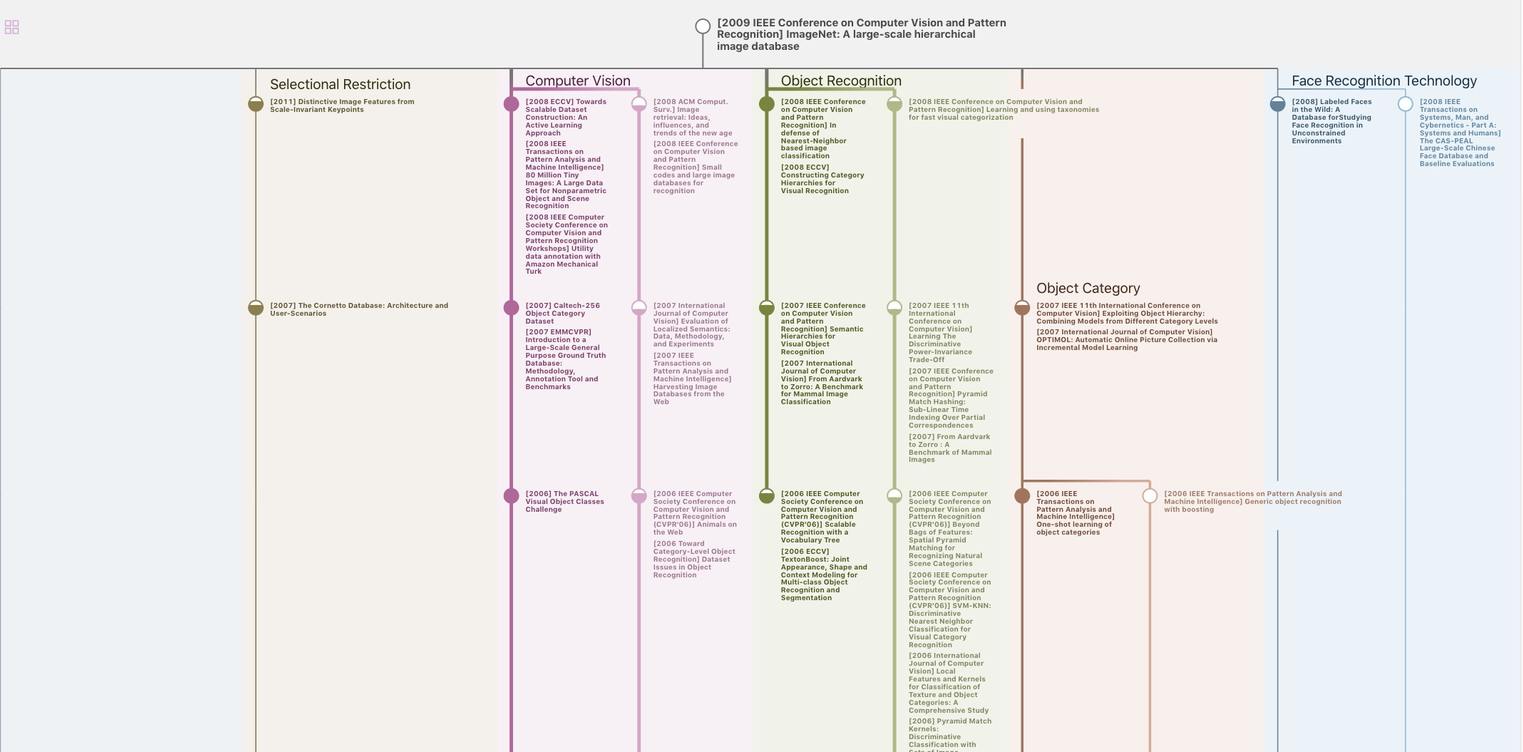
生成溯源树,研究论文发展脉络
Chat Paper
正在生成论文摘要