Joint Knowledge Graph and User Preference for Explainable Recommendation
ieee international conference computer and communications(2019)
摘要
Collaborative Filtering is recognized as an effective recommendation method, but it suffers from sparsity and cold start problems. To this end, this paper uses knowledge graph as a kind of auxiliary information to make more accurate recommendations, and fully considers the heterogeneity of entities and relations. A Joint Knowledge graph and user Preference model JKP is proposed, which combines user preferences and knowledge graph effectively for explainable recommendation. The model uses Multi-Layer Perceptron for knowledge graph embedding representations and item recommendation, and the task of knowledge graph embedding representation acts as a role to assist in recommendation tasks. At the same time, the model takes into account the preferences of the user while selecting the item. As for the different user preferences, the model adopts a soft mechanism to represent the user preference while interacting. The train process of JKP uses a joint method, in which the vector representation of the entity and the collaborative representation of the item in the recommendation algorithm can influence each other. The proposed model is validated on public dataset from real-world, and the results show the improvements not only in CTR tasks but also Top-N recommendations.
更多查看译文
关键词
knowledge graph,Multi-Layer Perceptron,multitask learning,joint training,explainable recommendation
AI 理解论文
溯源树
样例
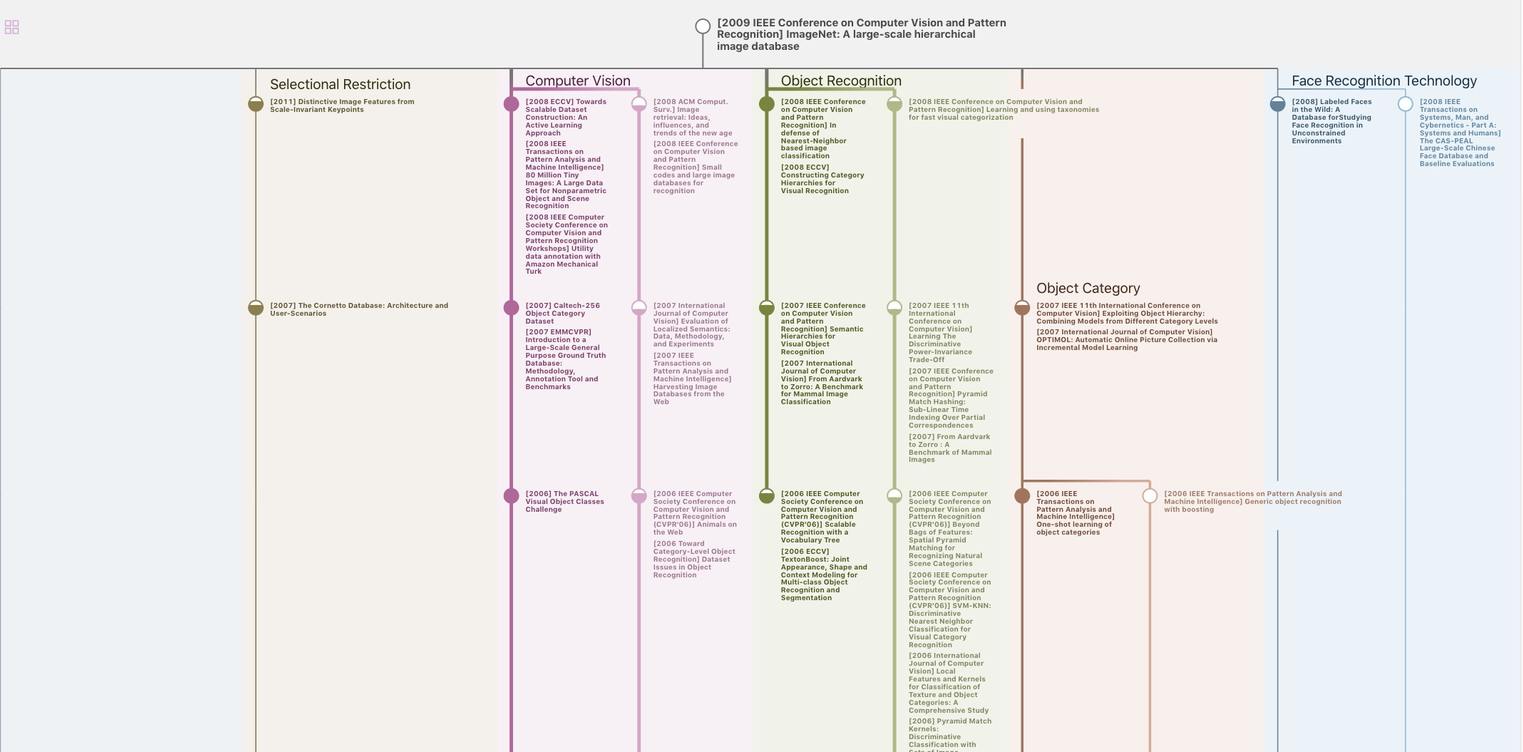
生成溯源树,研究论文发展脉络
Chat Paper
正在生成论文摘要