SPIDERnet: Attention Network For One-Shot Anomaly Detection In Sounds
ICASSP 2020 - 2020 IEEE International Conference on Acoustics, Speech and Signal Processing (ICASSP)(2020)
摘要
We propose a similarity function for one-shot anomaly detection in sounds (ADS) called SPecific anomaly IDentifiER network (SPIDERnet). In ADS systems, since overlooking an anomaly may result in serious incidents, we need to update such systems using an (often only one) overlooked anomalous sample. A previous study proposed the use of memory-based one-shot learning. A problem with this previous method is that it can detect only short anomalous sounds such as collision sounds because its similarity function is based on a naive mean-squared-error between the input and memorized spectrogram. To detect various anomalous sounds, SPIDERnet consists of (i) a neural network-based feature extractor for measuring similarity in embedded space and (ii) attention mechanisms for absorbing time-frequency stretching. Experimental results on two public datasets indicate that SPIDERnet outperforms conventional methods and robustly detects various anomalous sounds.
更多查看译文
关键词
Anomaly detection in sounds,acoustic condition monitoring,one-shot learning,and multi-head attention
AI 理解论文
溯源树
样例
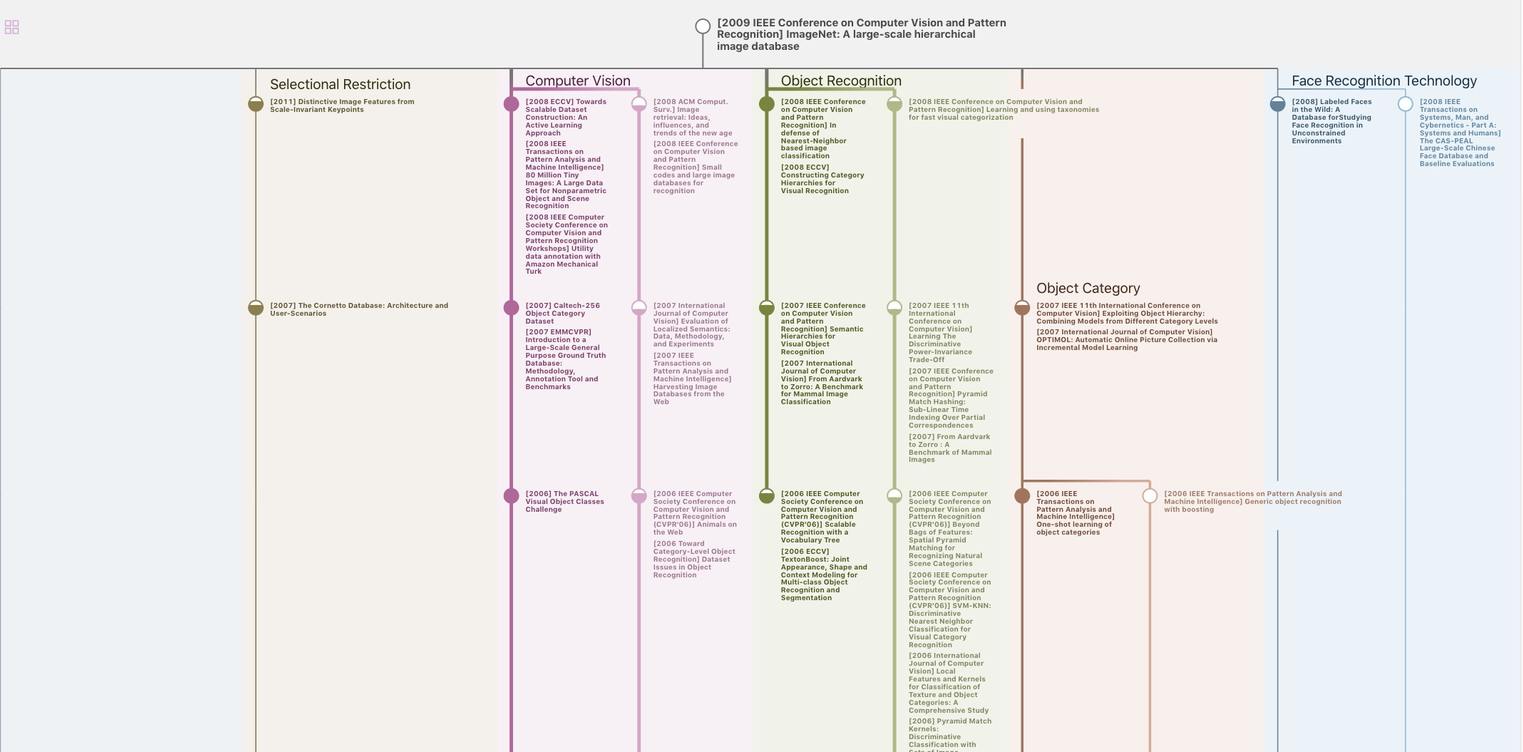
生成溯源树,研究论文发展脉络
Chat Paper
正在生成论文摘要