Pre-Training Audio Representations With Self-Supervision
IEEE SIGNAL PROCESSING LETTERS(2020)
摘要
We explore self-supervision as a way to learn general purpose audio representations. Specifically, we propose two self-supervised tasks: Audio2Vec, which aims at reconstructing a spectrogram slice from past and future slices and TemporalGap, which estimates the distance between two short audio segments extracted at random from the same audio clip. We evaluate how the representations learned via self-supervision transfer to different downstream tasks, either training a task-specific linear classifier on top of the pretrained embeddings, or fine-tuning a model end-to-end for each downstream task. Our results show that the representations learned with Audio2Vec transfer better than those learned by fully-supervised training on Audioset. In addition, by fine-tuning Audio2Vec representations it is possible to outperform fully-supervised models trained from scratch on each task, when limited data is available, thus improving label efficiency.
更多查看译文
关键词
Task analysis, Decoding, Training, Computer architecture, Spectrogram, Predictive models, Time-frequency analysis, Self-supervised learning, audio processing
AI 理解论文
溯源树
样例
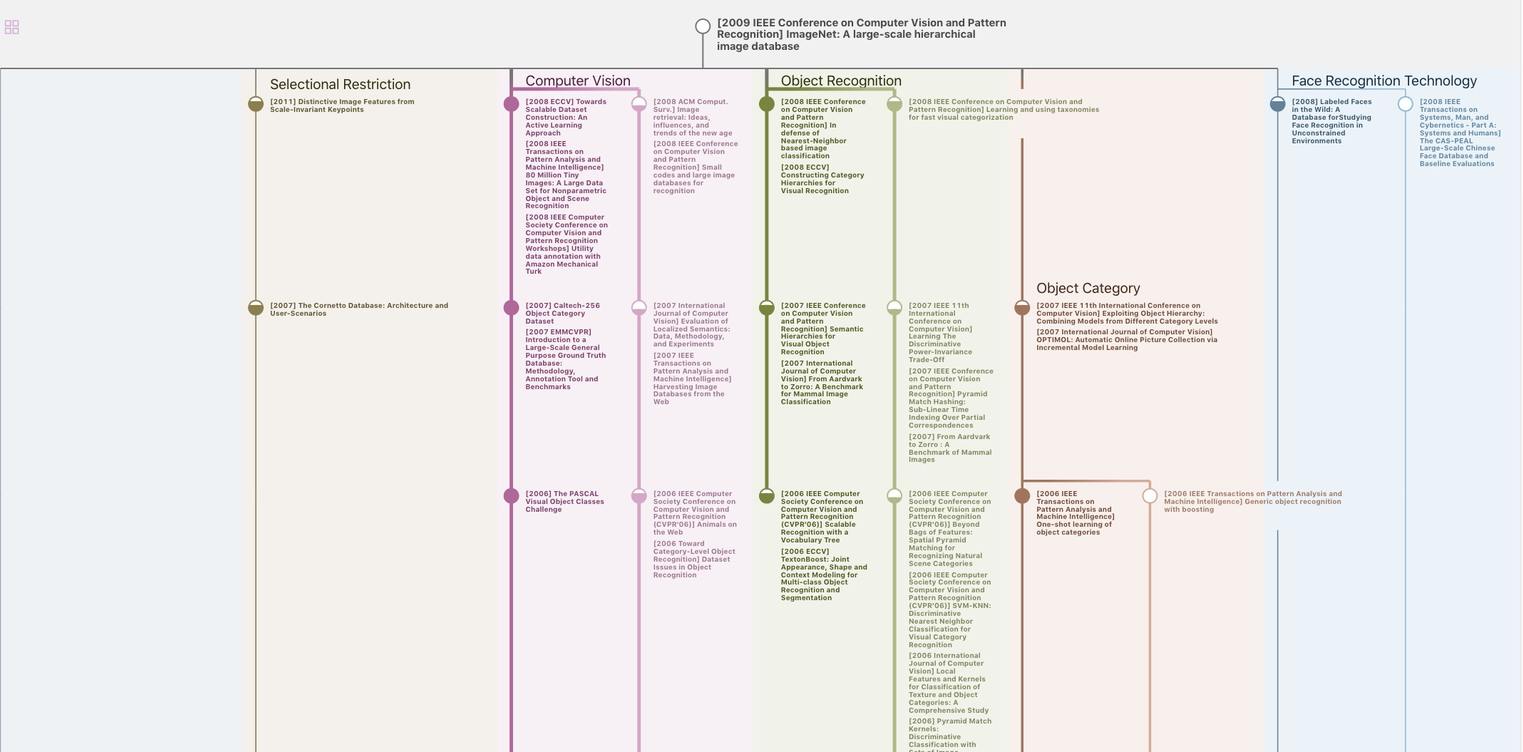
生成溯源树,研究论文发展脉络
Chat Paper
正在生成论文摘要