Spoken Document Retrieval Leveraging Bert-Based Modeling and Query Reformulation
2020 IEEE INTERNATIONAL CONFERENCE ON ACOUSTICS, SPEECH, AND SIGNAL PROCESSING(2020)
摘要
Spoken document retrieval (SDR) has long been deemed a fundamental and important step towards efficient organization of, and access to multimedia associated with spoken content. In this paper, we present a novel study of SDR leveraging the Bidirectional Encoder Representations from Transformers (BERT) model for query and document representations (embeddings), as well as for relevance scoring. BERT has produced extremely promising results for various tasks in natural language understanding, but relatively little research on it is devoted to text information retrieval (IR), let alone SDR. We further tackle one of the critical problems facing SDR, viz. a query is often too short to convey a user's information need, via the process of pseudo-relevance feedback (PRF), showing how information cues induced from PRF can be aptly incorporated into BERT for query expansion. In addition, such query reformulation through PRF also works in conjunction with additional augmentation of lexical features and confidence scores into the document embeddings learned from BERT. The merits of our approach are attested through extensive sets of experiments, which compare it with several classic and cutting-edge (deep learning-based) retrieval approaches.
更多查看译文
关键词
Spoken document retrieval, information retrieval, speech recognition, model augmentation, BERT, pseudo-relevance feedback, query reformulation
AI 理解论文
溯源树
样例
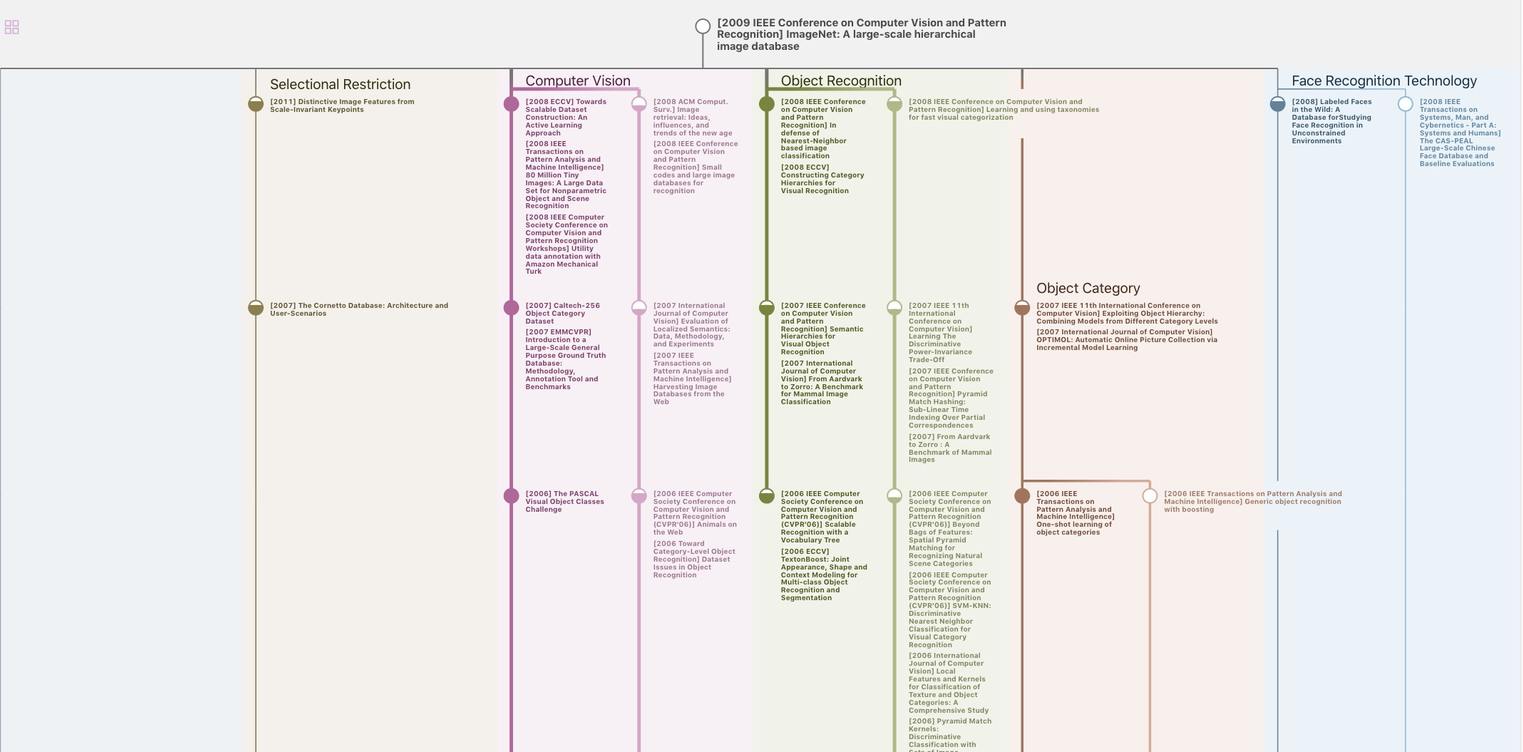
生成溯源树,研究论文发展脉络
Chat Paper
正在生成论文摘要