Decentralized Optimization With Non-Identical Sampling In Presence Of Stragglers
2020 IEEE INTERNATIONAL CONFERENCE ON ACOUSTICS, SPEECH, AND SIGNAL PROCESSING(2020)
摘要
We consider decentralized consensus optimization when workers sample data from non-identical distributions and perform variable amounts of work due to slow nodes known as stragglers. The problem of non-identical distributions and the problem of variable amount of work have been previously studied separately. In our work we analyse them together under a unified system model. We propose to combine worker outputs weighted by the amount of work completed by each. We prove convergence of the proposed method under perfect consensus, assuming straggler statistics are independent and identical across all workers for all iterations. Our numerical results show that under approximate consensus the proposed method outperforms the non-weighted scheme for both convex and non-convex objective functions.
更多查看译文
关键词
distributed computing, consensus optimization, non-identical sampling, federated learning
AI 理解论文
溯源树
样例
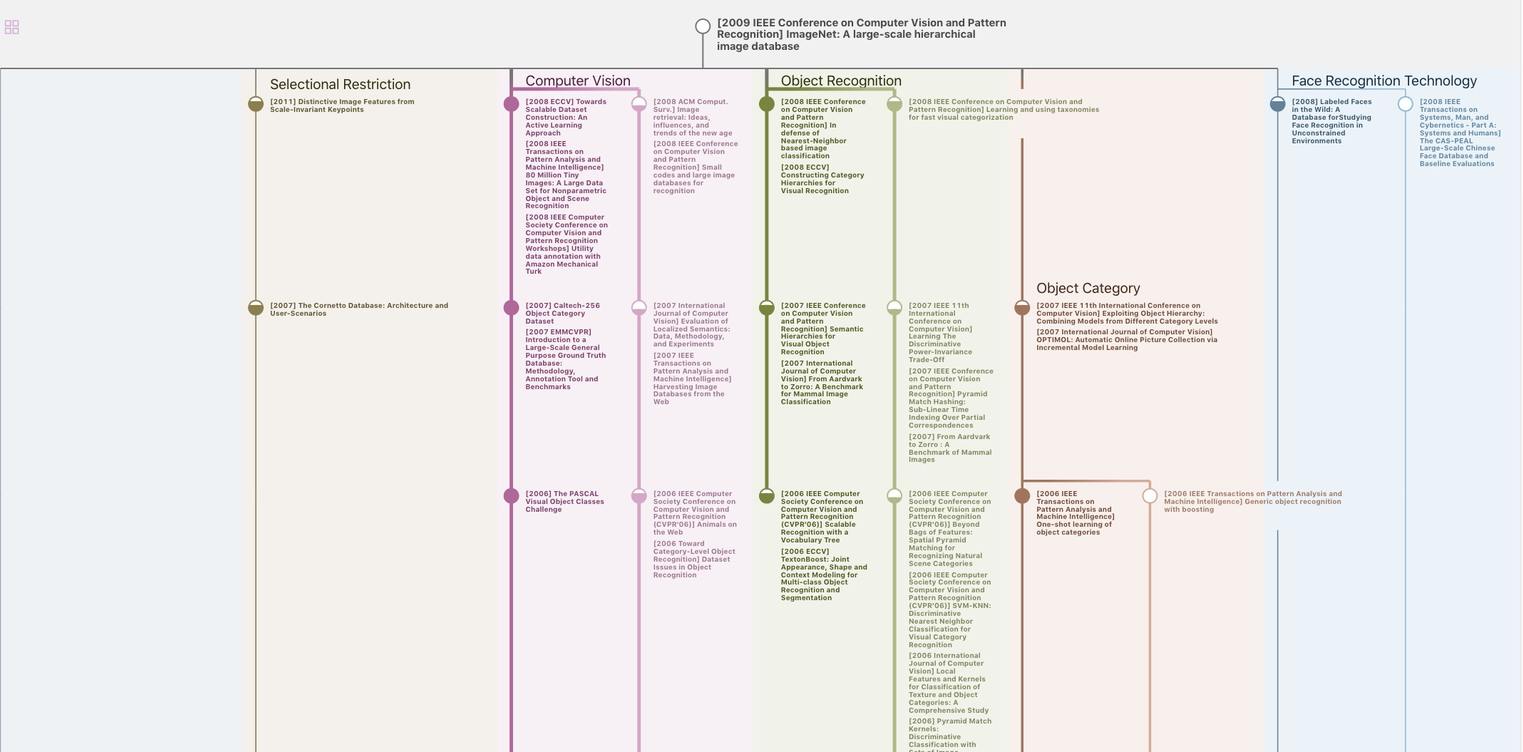
生成溯源树,研究论文发展脉络
Chat Paper
正在生成论文摘要