Spectrum Allocation in Wireless Networks for Crowd Labelling
ICASSP(2020)
摘要
The massive sensing data generated by Internet-of-Things will provide fuel for ubiquitous artificial intelligence (AI), while tremendous labels are required for AI model training via supervised learning. To tackle this challenge, a novel framework of wireless crowd labelling is proposed that downloads data to many imperfect mobile annotators for repetition labelling by exploiting multicasting in wireless networks. The integration of the rate-distortion theory and the principle of repetition labelling gives rise to a new tradeoff between radio-and-annotator resources under a constraint on labelling accuracy. Aiming at maximizing the labelling throughput, this work focuses on optimizing the joint annotator-andspectrum allocation (JASA). To develop an efficient solution approach, an optimal sequential annotator-clustering scheme is derived. Thereby, the optimal JASA policy can be found by an efficient tree search.
更多查看译文
关键词
Wireless Crowd Labelling, Multicast, Spectrum Allocation, Combinatorial Optimization
AI 理解论文
溯源树
样例
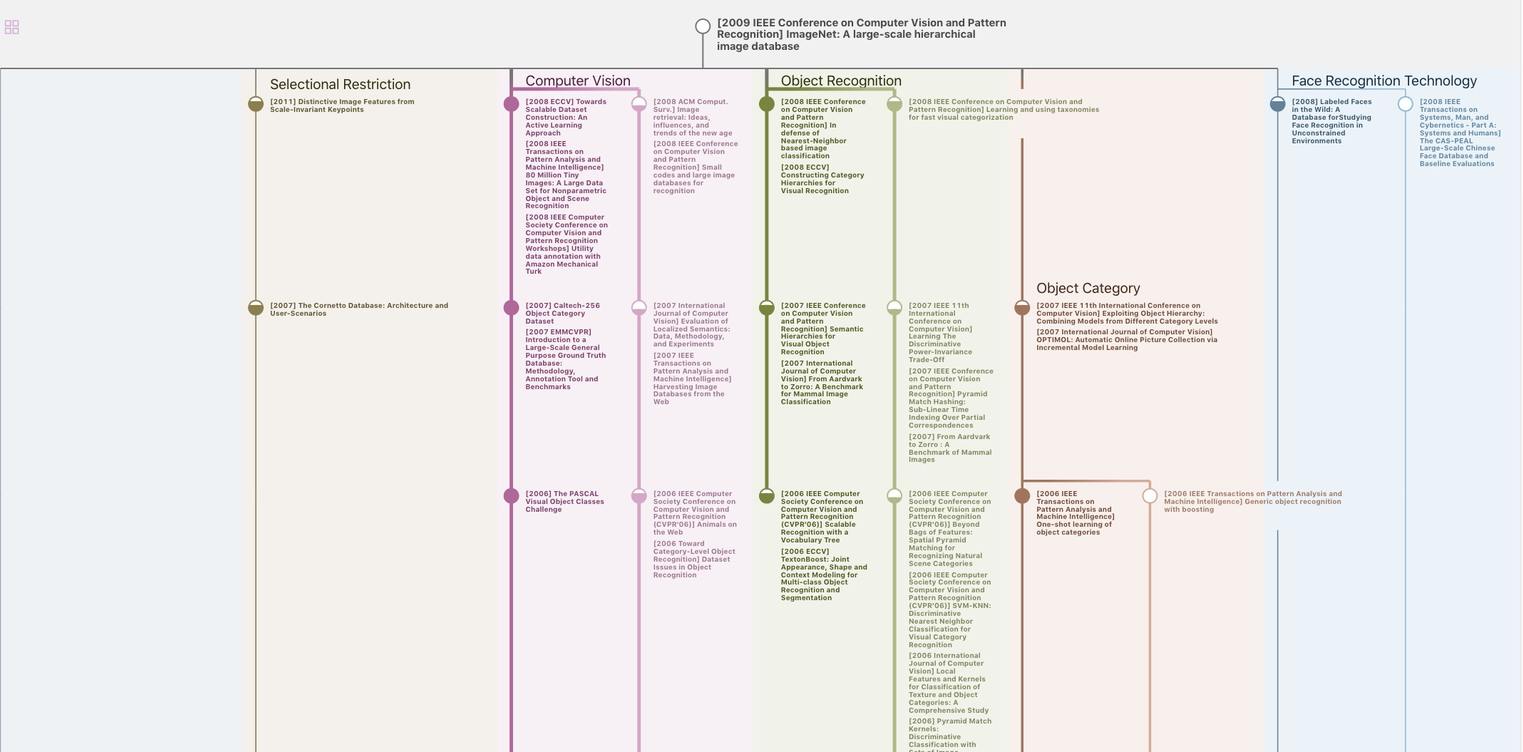
生成溯源树,研究论文发展脉络
Chat Paper
正在生成论文摘要