Wind Turbine Multi-label Fault Recognition Based on Combined Feature Selection and Neural Network
ieee conference energy internet and energy system integration(2019)
摘要
With the increasing status of renewable energy in energy system, efficient wind power generation has become one of the hot issues. As the layout and running environment of wind turbines are often complicated, high fault rate becomes a key factor influencing the improvement of power generation efficiency. In order to reduce operation, maintenance cost, and improve system reliability, we focus on early-warning wind turbine system fault type. This paper proposes a combined feature selection method and BP Neural Network model to realize the prediction of multi-label fault types of the wind turbine. This method combines the advantages of ML-ReliefF and PCA in feature selection to farthest minimize irrelevant and redundant features. The BP Neural Network is then constructed and trained to predict multiple fault types which will possibly occur. Based on the real SCADA system monitoring data, our experimental results show that feature selection method has significant effect on prediction, and the combined one of ML-ReliefF and PCA performs better than each single one of them. Based on it, the BP Neural Network multi-label fault prediction model can implement the fault early warning task with superior accuracy.
更多查看译文
关键词
Feature Selection,Neural Network,Fault Prediction,Pattern Recognition
AI 理解论文
溯源树
样例
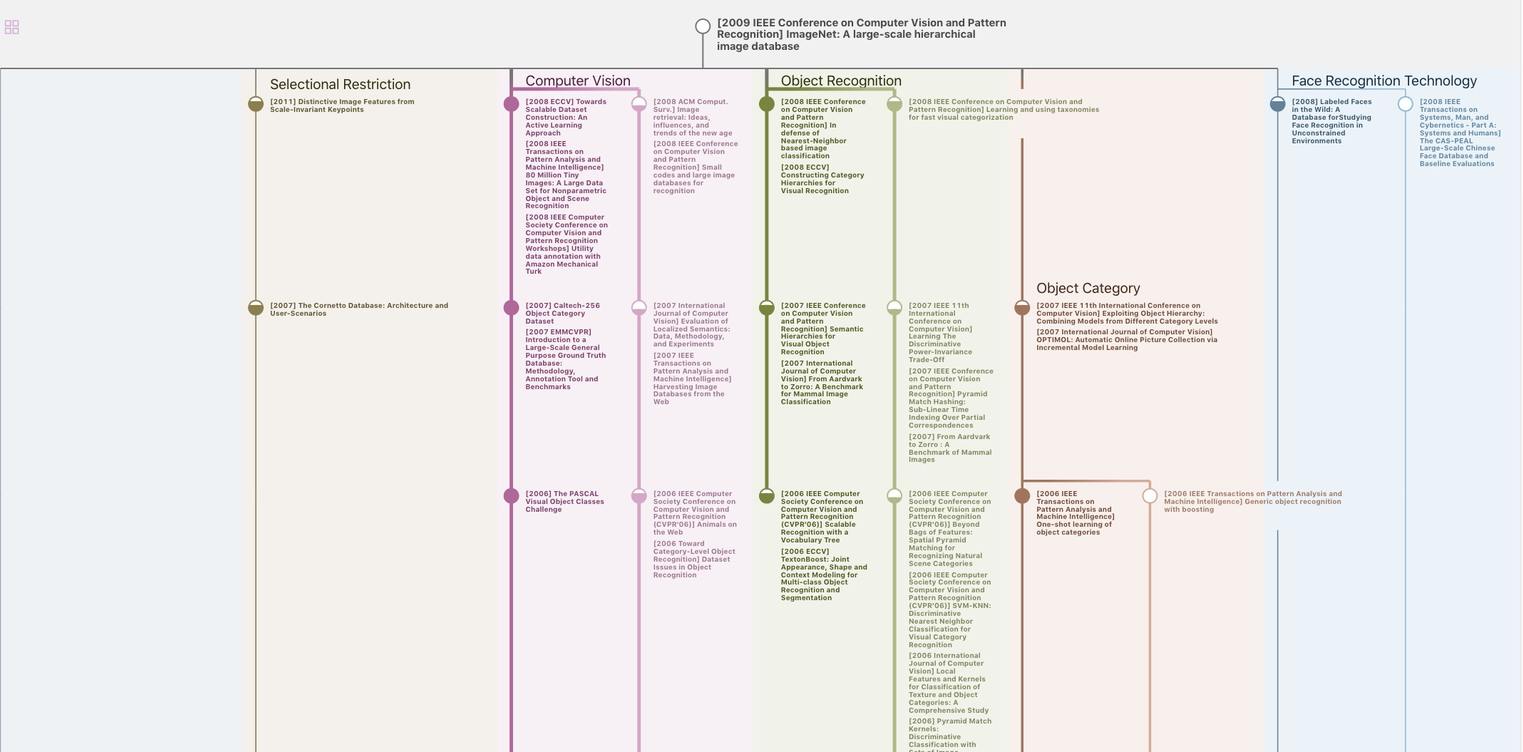
生成溯源树,研究论文发展脉络
Chat Paper
正在生成论文摘要