Multi-Task Center-Of-Pressure Metrics Estimation From Skeleton Using Graph Convolutional Network
2020 IEEE INTERNATIONAL CONFERENCE ON ACOUSTICS, SPEECH, AND SIGNAL PROCESSING(2020)
摘要
Center of pressure (COP) is an important measurement of postural and gait control in human biomechanical studies. A vision-based estimation of COP metrics offers a way to obtain these gold-standard metrics for the detection of balance and gait problems. In this paper, we propose an end-to-end framework to estimate the COP path length and the COP positions from the 3D skeleton, utilizing the spatial-temporal features learned by graph convolutional networks. We propose two single-task models for each metric and a multi-task approach jointly learning two metrics. To facilitate this line of research, we also release a novel 3D skeleton dataset containing a wide variety of action patterns with synchronized COP labels. The experiments on the dataset validate that our framework achieves state-of-the-art accuracies for both COP path length and COP position estimations, while the multi-task approach could yield more accurate and robust performance on COP path length estimation compared to the singletask model.
更多查看译文
关键词
center of pressure, balance control, graph convolutional network, multi-task learning
AI 理解论文
溯源树
样例
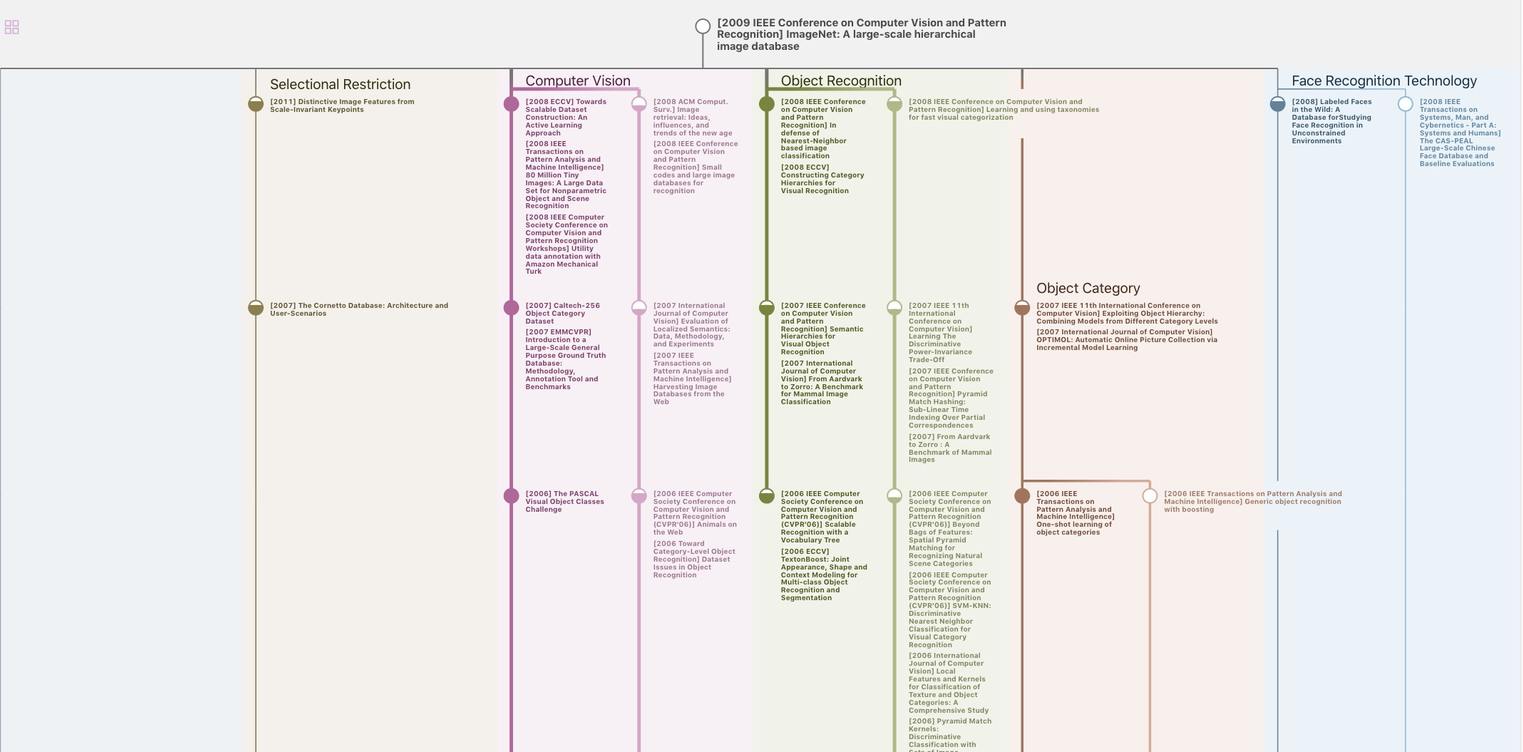
生成溯源树,研究论文发展脉络
Chat Paper
正在生成论文摘要