Growing and Pruning Selective Ensemble Regression for Nonlinear and Nonstationary Systems
IEEE ACCESS(2020)
摘要
For a selective ensemble regression (SER) scheme to be effective in online modeling of fast-arriving nonlinear and nonstationary data, it must not only be capable of maintaining a most up to date and diverse base model set but also be able to forget old knowledge no longer relevant. Based on these two important principles, in this paper, we propose a novel growing and pruning SER (GAP-SER) for time-varying nonlinear data. Specifically, during online operation, newly emerging process state is automatically identified and a local linear model is fitted to it. This adaptive growing strategy therefore maintains a most up to date and diverse local model set. The online prediction model is then constructed as a selective ensemble from the local linear model set based on a probability metric. Moreover, a pruning strategy is derived to remove 'unwanted' out of date local linear models in order to achieve low online computational complexity without sacrificing online modeling accuracy. A chaotic time series prediction and two real-world data sets are used to demonstrate the superior online modeling performance of the proposed GAP-SER over a range of benchmark schemes for nonlinear and nonstationary systems, in terms of online prediction accuracy and computational complexity.
更多查看译文
关键词
Adaptation models,Data models,Computational modeling,Predictive models,Brain modeling,Computational complexity,Measurement,Nonlinear and nonstationary data,local linear model,growing model,pruning model,selective ensemble
AI 理解论文
溯源树
样例
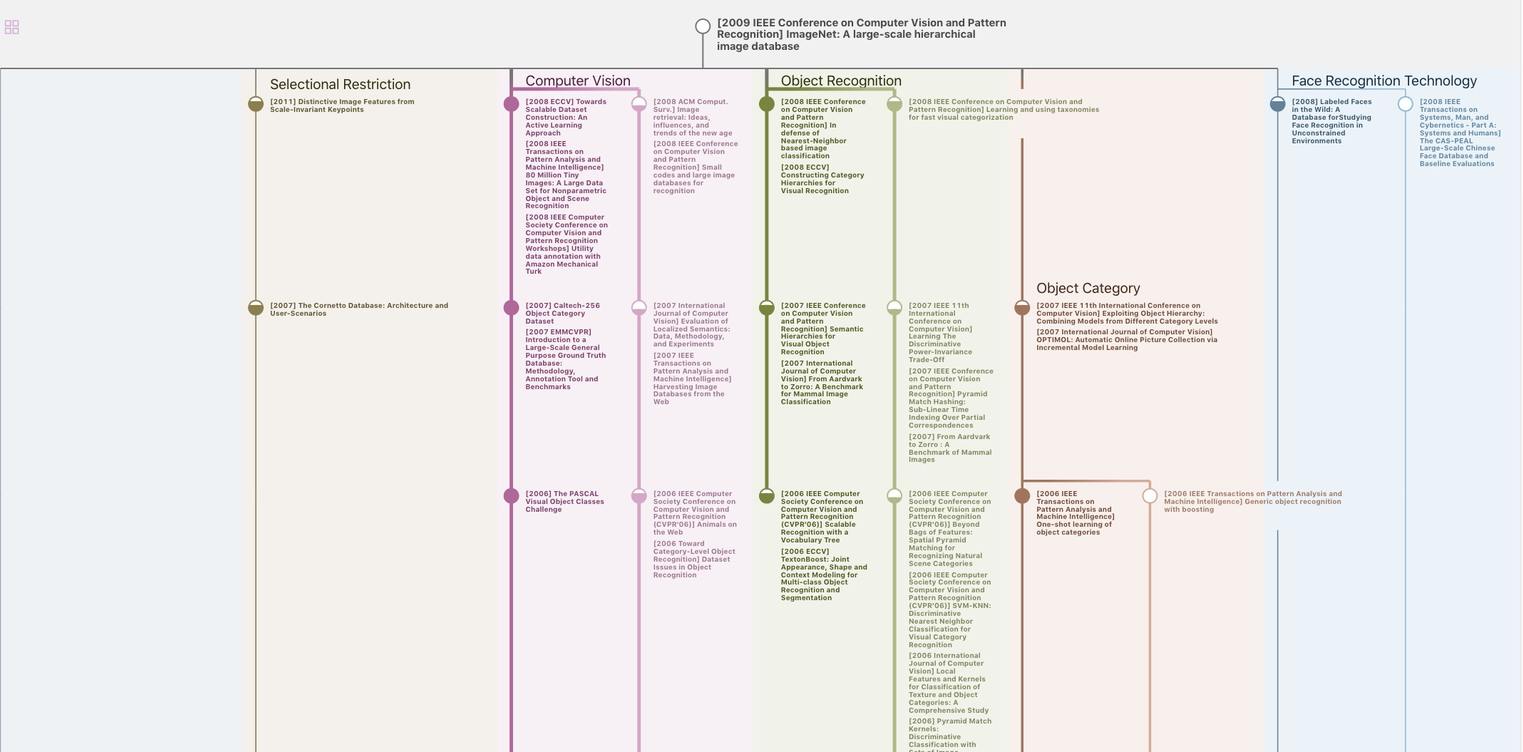
生成溯源树,研究论文发展脉络
Chat Paper
正在生成论文摘要