Spotting Key Members in Networks: Clustering-Embedded Eigenvector Centrality
IEEE systems journal(2020)
摘要
Identifying key members in a social network is critical to understand the underlying system behavior. Whereas there are several measures designed to discern the most central member, they fail to identify a central set of members and at the same time reveal the spheres of influence of the individuals in such central set. Here, we combine eigenvector centrality with clustering to design a mathematical programming formulation capable of detecting key members while preventing their spheres of influence from overlapping. Our computational experience reproduces these two features as different aspects of the same phenomenon. The optimal set of key members and their spheres of influence are identified in real-life networks and synthetic ones. For the former, community structures are consistent with existing knowledge about the instances. For the latter, network underlying organization is known a priori and it is perfectly uncovered. Experiments further reveal previously neglected nodes to be optimal key members. The size of the instances tested reach several hundreds of nodes and thousands of links.
更多查看译文
关键词
Mathematical model,Mathematical programming,Social networking (online),Sociology,Computational modeling,Organizations,Clustering,eigenvector centrality,mathematical optimization,social networks
AI 理解论文
溯源树
样例
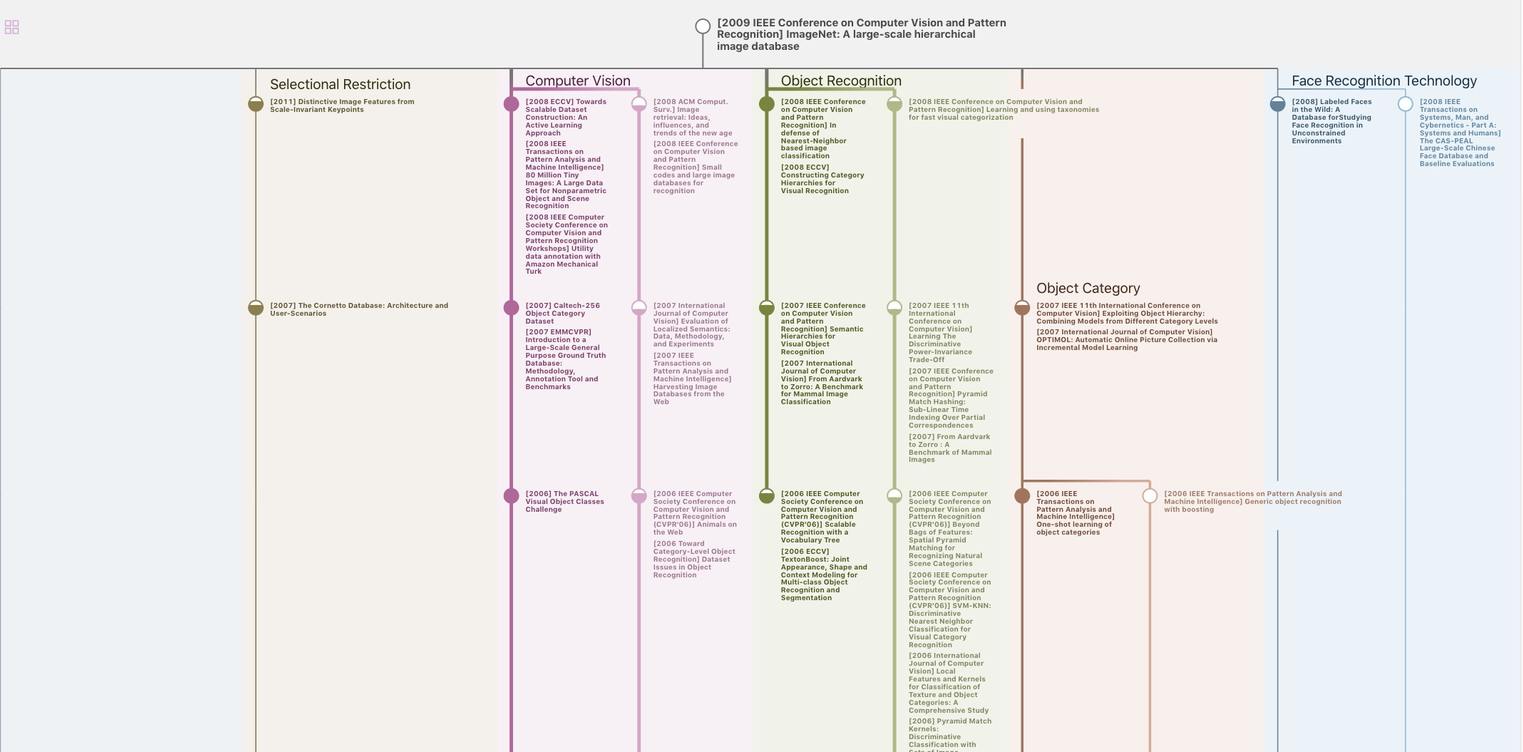
生成溯源树,研究论文发展脉络
Chat Paper
正在生成论文摘要