Joint Latent Class Analysis For Longitudinal Data: An Application On Adolescent Emotional Well-Being
COMMUNICATIONS FOR STATISTICAL APPLICATIONS AND METHODS(2020)
摘要
This study proposes generalized models of joint latent class analysis (JLCA) for longitudinal data in two approaches, a JLCA with latent profile (JLCPA) and a JLCA with latent transition (JLTA). Our models reflect cross-sectional as well as longitudinal dependence among multiple latent classes and track multiple class-sequences over time. For the identifiability and meaningful inference, EM algorithm produces maximum-likelihood estimates under local independence assumptions. As an empirical analysis, we apply our models to track the joint patterns of adolescent depression and anxiety among US adolescents and show that both JLCPA and JLTA identify three adolescent emotional well-being subgroups. In addition, JLCPA classifies two representative profiles for these emotional well-being subgroups across time, and these profiles have different tendencies according to the parent-adolescent-relationship subgroups.
更多查看译文
关键词
joint latent class profile analysis, joint latent transition analysis, EM algorithm, adolescent depression
AI 理解论文
溯源树
样例
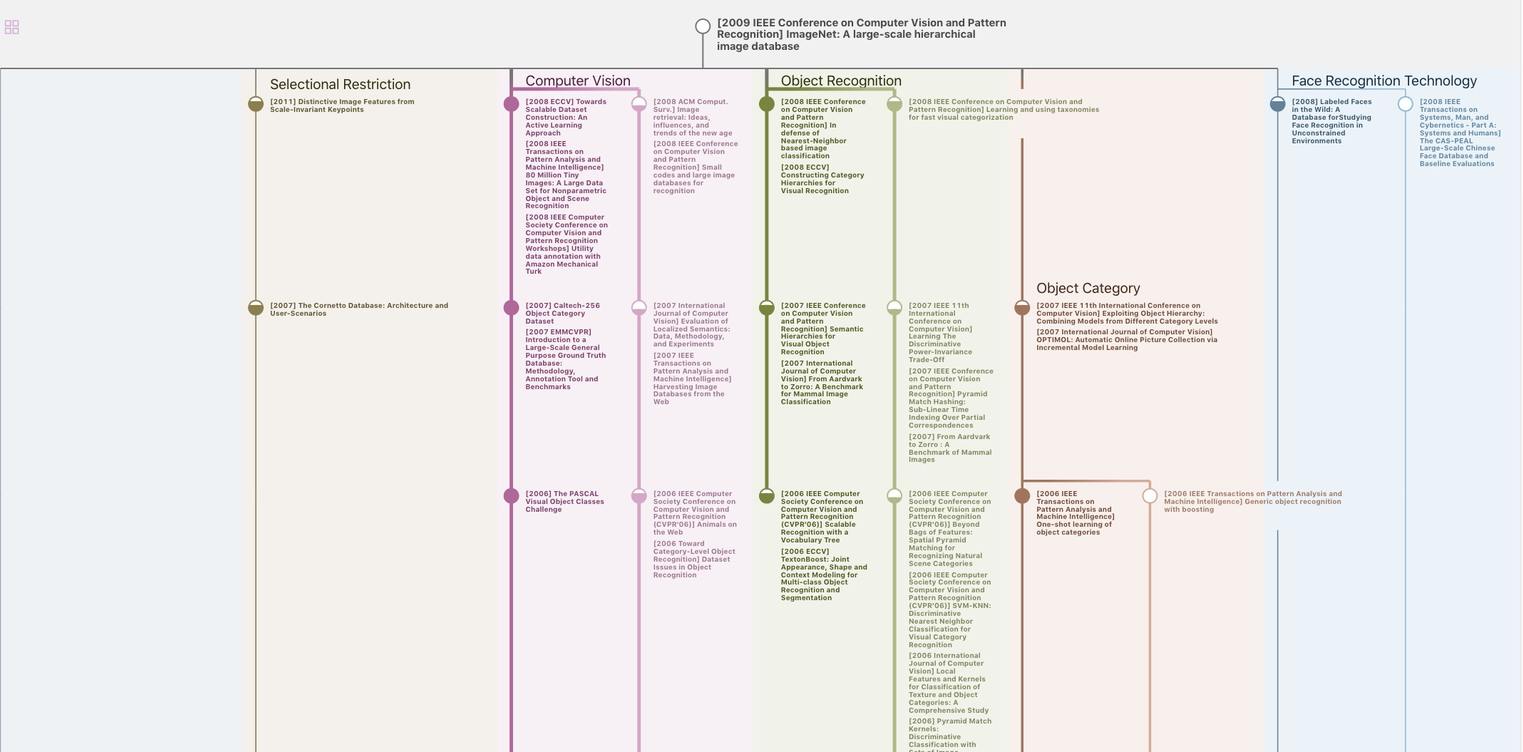
生成溯源树,研究论文发展脉络
Chat Paper
正在生成论文摘要