LncRNA-Disease Associations Prediction Based on Neural Network-Based Matrix Factorization
IEEE Access(2023)
摘要
Numerous experiments have demonstrated that long non-coding RNA(lncRNA) play an important role in various systems of the human body. LncRNAdeletions or mutations can cause human disease. The prediction of lncRNA-disease associations is conducive to the diagnosis and prevention of complex diseases. As we all know, it is a time-consuming and expensive process to predict lncRNA-disease associations via biological experiments. However, the computation methods can effectively discover lncRNA-disease associations with less human and material resources. In this paper, we propose a neural network-based matrix factorization model to predict lncRNA-disease associations, which is called NeuMFLDA. NeuMFLDA first converts the one-hot encoding of disease or lncRNA into word vector via the embedding layer. Then combined with the memorization of the conventional matrix factorization and the generalization of the multi-layer perceptron, the lncRNA-disease associations can be predicted more accurately. In addition, as opposed to conventional pointwise loss function, a new pairwise loss function is proposed to update our model parameters. Our new loss function optimizes the model from the perspective of ranking priority, which is more in line with the solution to the lncRNA-disease associations prediction task. Experiments show that NeuMFLDA reaches average AUCs of 0.904 +/- 0 :003 and 0.918 +/- 0 :002 in the framework of 5-fold cross validation and Leave-one-out cross validation, which is superior to three the-state-of-art methods. In case studies, 9, 9 and 8 out of top-10 candidate lncRNAs are verified by recently published literatures for hepatocelluar carcinoma, kidney cancer and ovarian cancer, respectively. In short, NeuMFLDA is an effective tool for predicting lncRNA-disease associations.
更多查看译文
关键词
LncRNA-disease associations,embedding,neural network,pairwise loss function
AI 理解论文
溯源树
样例
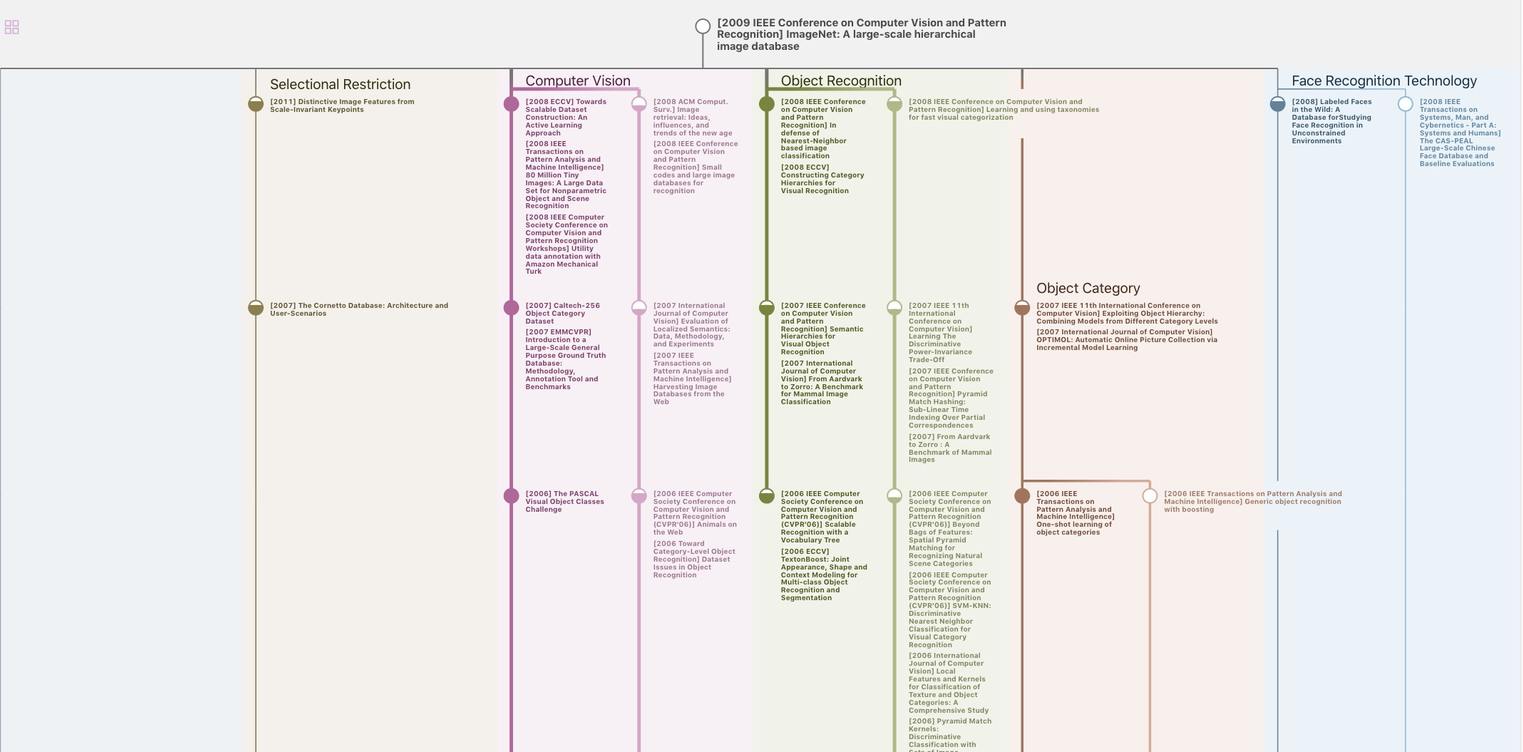
生成溯源树,研究论文发展脉络
Chat Paper
正在生成论文摘要