An Integrated Method for High-dimensional Imbalanced Assembly Quality Prediction Supported by Edge Computing
IEEE ACCESS(2020)
摘要
With the rapid expansion of Industrial Internet of Things, cloud computing and artificial intelligence, many intelligent information services have been developed in smart factories. One of the most important applications is helping factory managers predict the quality of assembled products. Traditional prediction methods of assembly quality mainly focus on building classification or regression models with high accuracy. However, less attention is paid to high-dimensional and imbalanced data, which is a special but common scenario at real-life assembly quality prediction. In this paper, we first use random forest to reduce dimension and analyze critical-to-quality characteristics. Then, a SMOTE-Adaboost method with jointly optimized hyperparameters is proposed for imbalanced data classification in assembly quality prediction. In addition, edge computing is introduced to improve the efficiency and flexibility of quality prediction. Finally, the practicality and effectiveness of the proposed method are verified by a case study of wheel bearing assembly line, and the experimental results show that the proposed method is superior to other classification methods in assembly quality prediction.
更多查看译文
关键词
Edge computing,Cloud computing,Predictive models,Quality assessment,Product design,Image edge detection,Assembly quality prediction,high-dimensional,imbalanced data classification,SMOTE-Adaboost,edge computing
AI 理解论文
溯源树
样例
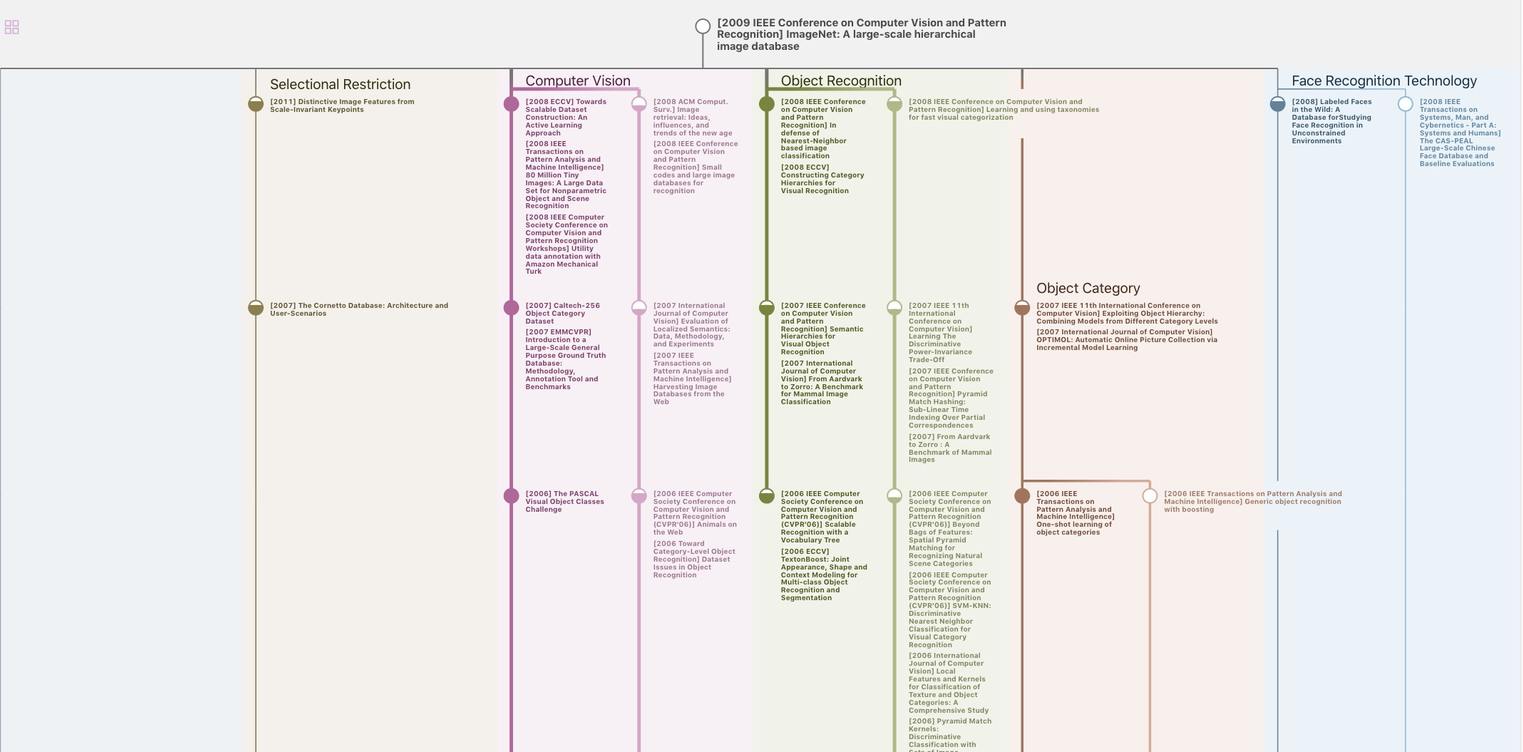
生成溯源树,研究论文发展脉络
Chat Paper
正在生成论文摘要