Rebalancing the car-sharing system with reinforcement learning
World Wide Web(2020)
摘要
With the sharing economy boom, there is a notable increase in the number of car-sharing corporations, which provided a variety of travel options and improved convenience and functionality. Owing to the similarity in the travel patterns of the urban population, car-sharing system often faces the problem of imbalance in the number of shared cars within the spatial distribution, especially during the rush hours. There are many challenges in redressing this imbalance, such as insufficient data and the large state space. In this study, we propose a new reward method called Double P (Picking & Parking) Bonus (DPB). We model the research problem as a Markov Decision Process (MDP) problem and introduce Deep Deterministic Policy Gradient, a state-of-the-art reinforcement learning framework, to find a solution. The results show that the rewarding mechanism embodied in the DPB method can indeed guide the users’ behaviors through price leverage, increase user stickiness, and cultivate user habits, thereby boosting the service provider’s long-term profit. In addition, taking the battery power of the shared car into consideration, we use the method of hierarchical reinforcement learning for station scheduling. This station scheduling method encourages the user to place the car that needs to be charged on the charging post within a certain site. It can ensure the effective use of charging pile resources, thereby rendering the efficient functioning of shared cars.
更多查看译文
关键词
Reinforcement learning,Car-sharing system,Scheduling
AI 理解论文
溯源树
样例
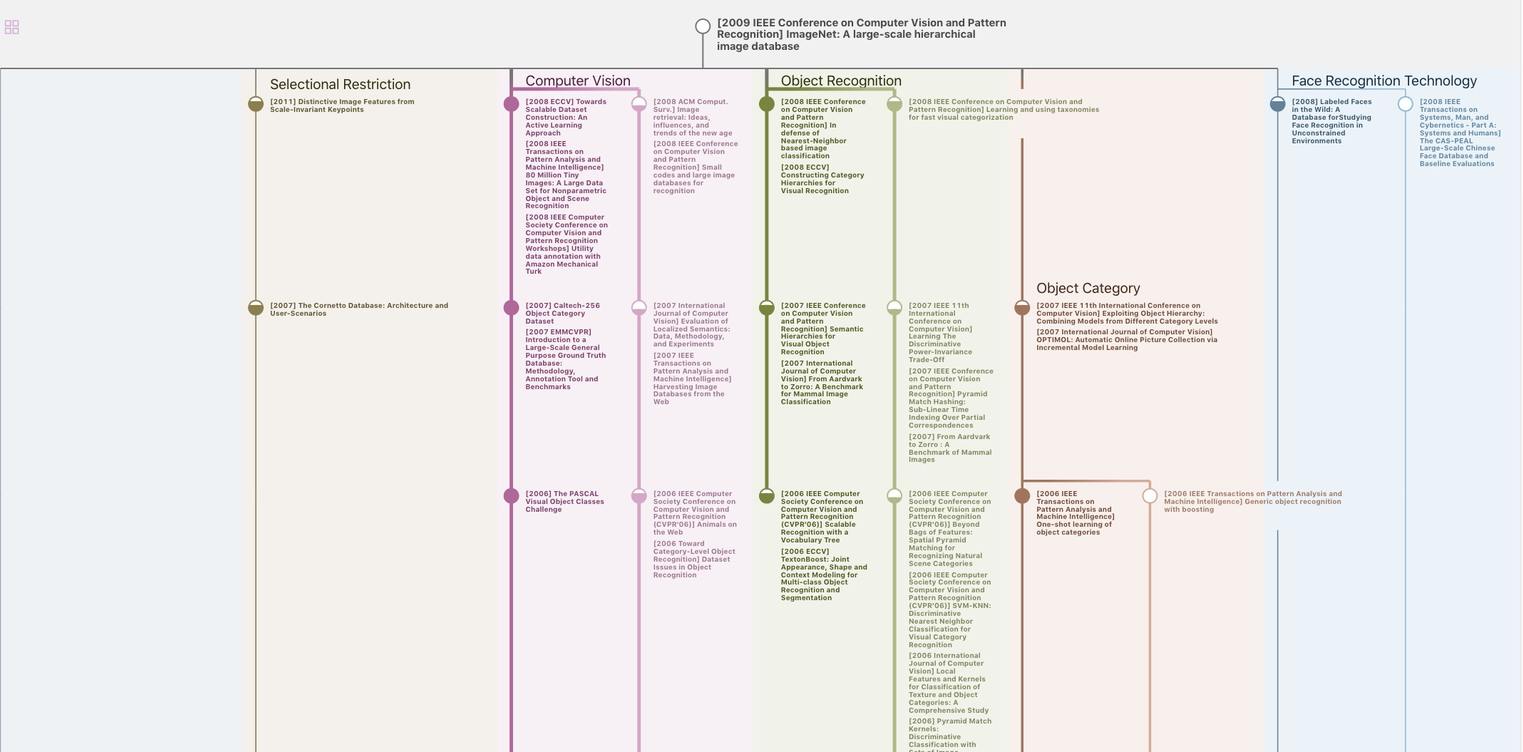
生成溯源树,研究论文发展脉络
Chat Paper
正在生成论文摘要