Spatial Interpolation of Gauge Measured Rainfall Using Compressed Sensing
ASIA-PACIFIC JOURNAL OF ATMOSPHERIC SCIENCES(2020)
摘要
In this work, we suggest new spatial precipitation interpolation schemes using compressed sensing (CS), which is a new framework for signal acquisition and smart sensor design. Using CS, the precipitation maps are recovered in high resolution by obtaining sparse coefficients of radial basis functions(RBFs). Two types of methods are designed according to the construction methods of CS matrix. In the first type, the CS matrix is derived as the product of an m × n ( n ≫ m ) weights matrix of inverse distance weighting (IDW) and an n × n radial basis function (RBF) matrix. The second type of CS matrix consists of an m × n RBF matrix that depends on a few observation vectors and a number of n unknown vectors. The advantage of the proposed CS methods is that it can be represented at a high resolution because it is interpolated based on a large number of bases (or degrees of freedom). This prevents the variance value from being much smaller than the actual value due to interpolation using a few observation scales. To test our CS interpolation schemes, interpolation results were compared with IDW, Ordinary Kriging (OK) and RBF interpolation methods for analytic test function and some actual rainfall data. In the case of an analytic test function, when the proposed method is compared at high resolution, the error from the true value is the smallest. In real rainfall data, comparison with real values is not possible at high resolutions, but the error with the observed data is the smallest in terms of ‘spatial variogram’. In addition, the proposed CS method generates hight resolution data from rainfall cases, showing promising results when identifying peaks.
更多查看译文
关键词
Compressed sensing, Inverse distance weights, Ordinary Kriging, Precipitation interpolation, Radial basis function
AI 理解论文
溯源树
样例
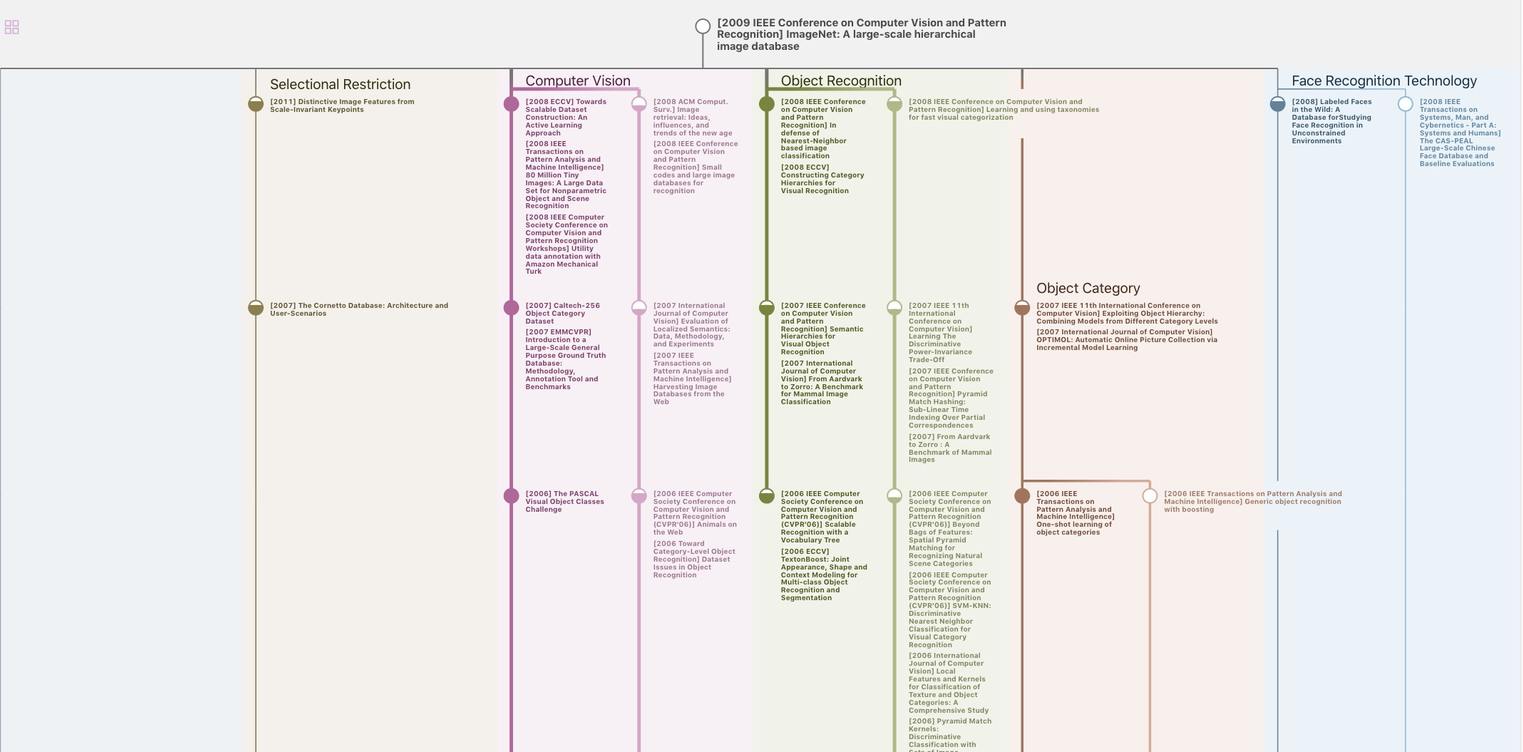
生成溯源树,研究论文发展脉络
Chat Paper
正在生成论文摘要