A priori evaluation of the Double-conditioned Conditional Source-term Estimation model for high-pressure heptane turbulent combustion using DNS data obtained with one-step chemistry
Combustion and Flame(2020)
摘要
An evaluation of the submodels constituting the Double-Conditioned Source-term Estimation (DCSE) method for utilization in Large Eddy Simulation (LES) is presented which makes use of a single realization of a turbulent high-pressure reactive-flow database obtained from Direct Numerical Simulation (DNS). A filtered and coarsened DNS (FCDNS) field is first created to mimic a real LES field, and the FCDNS values for thermodynamic variables (temperature, density and species mass fractions) are used as inputs to the DCSE model to predict the FCDNS reaction rates. It is found that there are significant errors in the predictions of the filtered reaction rate compared to the template. These errors are attributed to three important aspects of the model. First, the conditional-filtered values of the thermodynamic variables are found by an inversion of an integral equation, but this inversion requires the definition of spatial ensembles in which it must be performed; these ensembles are a subset of the entire spatial domain containing the LES volumes. A drastic reduction in the number of ensembles from the number of LES volumes (i.e. the largest possible number of such ensembles) deteriorates the model, whereas a further and smaller such reduction has a small effect. Second, the procedure for the integral inversion introduces problems related to the method selected to regularize the inversion. Third, by far the largest source of error was found to be due to the modelling of the joint probability density function of the conditioning variables: assuming a β-PDF for the marginal PDF of the reaction progress variable is shown to be a particularly poor choice, as is the assumption that the conditioning variables are statistically independent.
更多查看译文
关键词
High-pressure combustion,Modelling,DCSE,Inversion,PDF
AI 理解论文
溯源树
样例
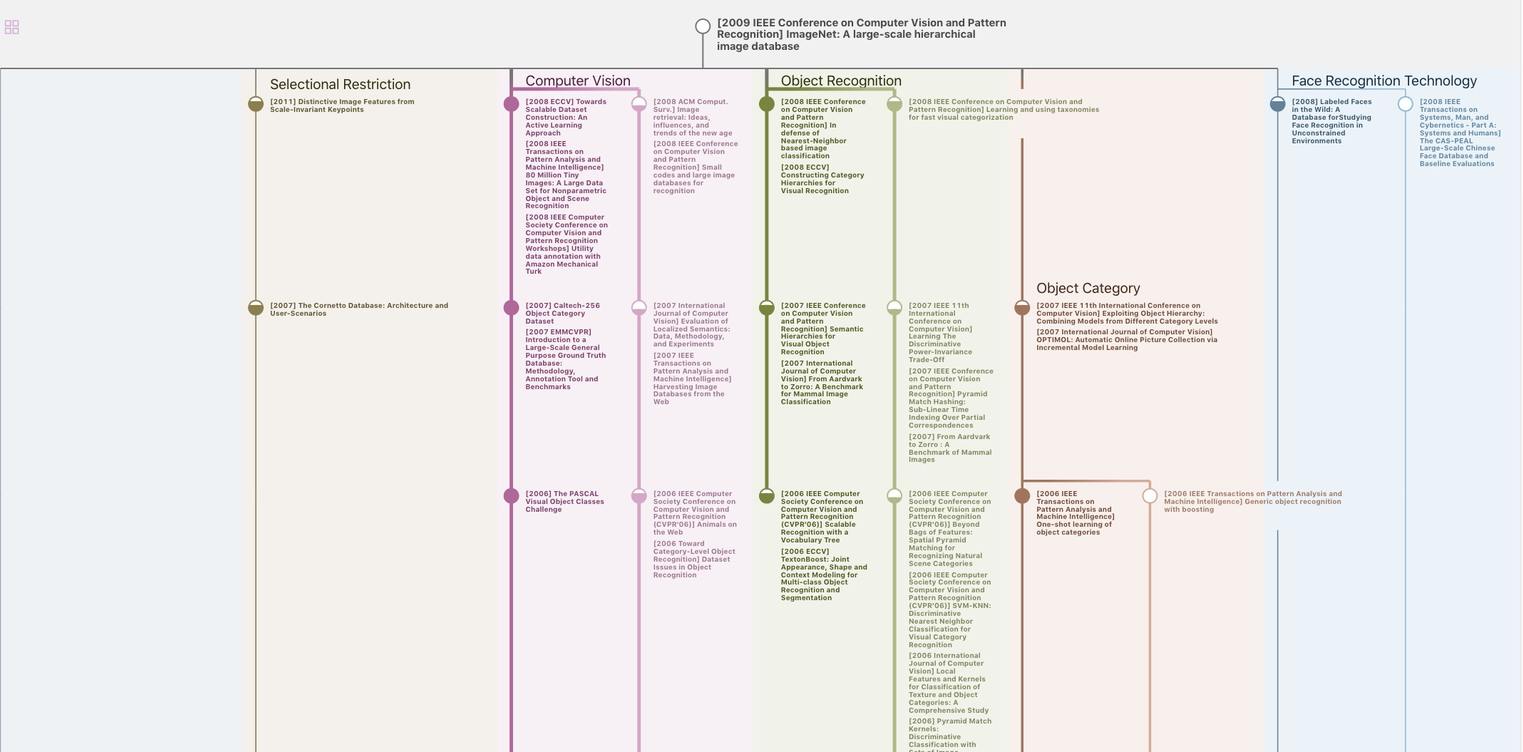
生成溯源树,研究论文发展脉络
Chat Paper
正在生成论文摘要