Learning double weights via data augmentation for robust sparse and collaborative representation-based classification
MULTIMEDIA TOOLS AND APPLICATIONS(2020)
摘要
Image classification is a hot technique applied in many multimedia systems, where both l 1 and l 2 regularizations have shown potential for robust sparse representation-based image classification. However, previous studies showed that l 1 or l 2 alone cannot ensure a robust result. The robustness of a classifier depends on the nature of the dataset most of the time. What is worse, data augmentation may make the dataset more complicated, which leads a sparse model become harder to optimize. In this paper, a novel sparse representation that learns double weights through data augmentation is proposed for robust image classification. The first weight combines the two coefficients solved by l 1 and l 2 regularizations to obtain a more discriminative representation, while the second weight integrates the residuals obtained from the original and virtual samples, to take full advantage of diversity created by data augmentation. The double-weight process builds a robust model that is able to deal with the augmented but variational datasets. Experiments on popular facial and object datasets demonstrate the promising performance of the proposed method. Learning double weights via sample virtualization is helpful to develop multimedia applications.
更多查看译文
关键词
Image classification,Sparse representation,Regularization,Augmentation
AI 理解论文
溯源树
样例
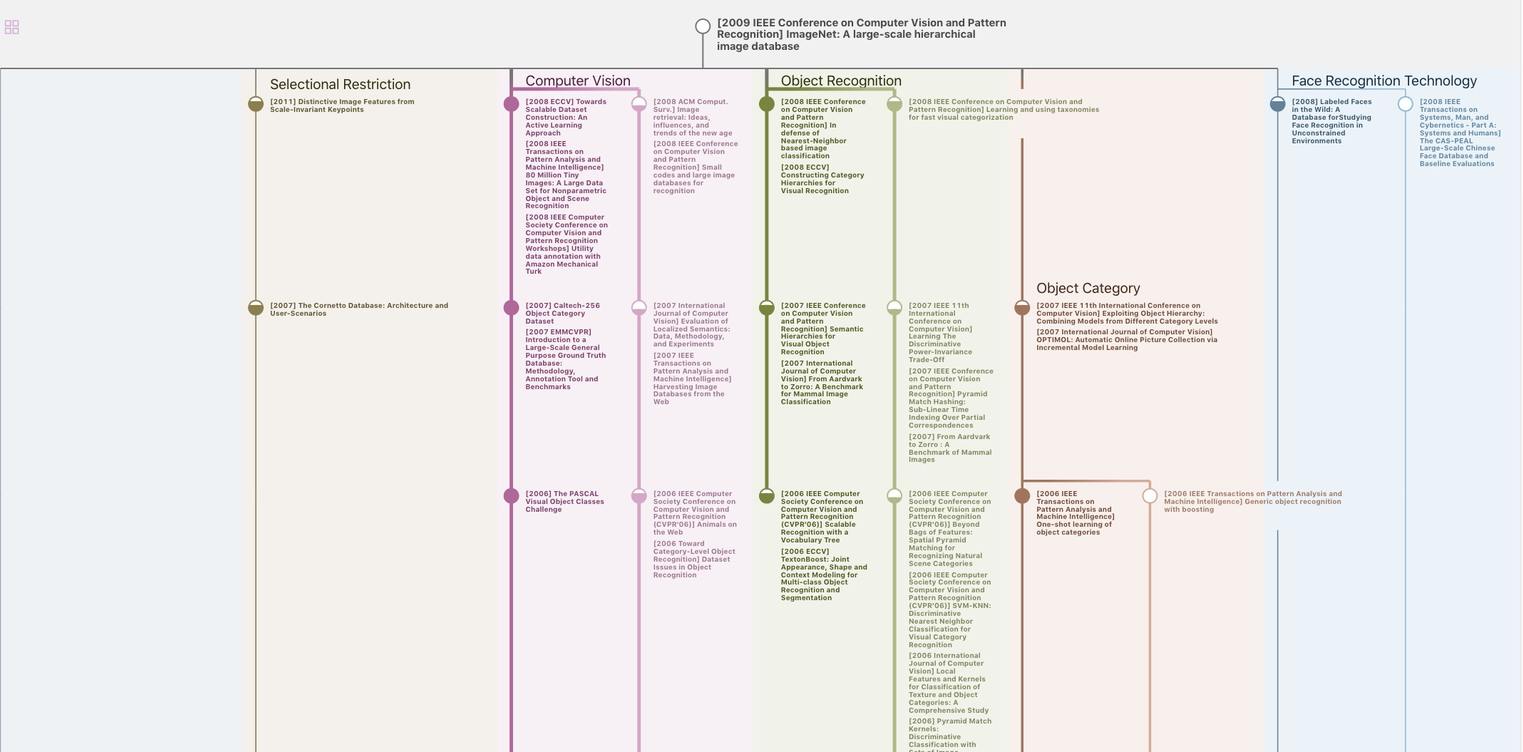
生成溯源树,研究论文发展脉络
Chat Paper
正在生成论文摘要