Cross Model Deep Learning Scheme for Automatic Modulation Classification
IEEE ACCESS(2020)
摘要
Deep Neural Networks (DNNs) have achieved remarkable accuracy improvements for automatic modulation classification. However, the employed networks often have millions of parameters and need very high computation, which makes it difficult to deploy these models on portable devices with limited resources. We propose a cross model deep learning scheme to build a lightweight deep network for accurate modulation classification. Firstly, a large Hybrid DNN (HDNN) that is composed of convolutional and recurrent layers is constructed and trained for automatic and accurate classification of signals. Then we build a smaller Layered Resnet Network (LRN) with shallow layers and few nodes. The HDNN and LRN are taken as a Teacher Model (TM) and a Student Model (SM) respectively. Finally, a knowledge distillation method is proposed to guide the learning of the SM, by formulating a teaching loss from the prediction of the TM to train the SM. The performances of the proposed HDNN and LRN are investigated on the public RadioML2016.10a and RadioML2016.10b data sets. The experimental results show that the trained HDNN presents state-of-the-art classification results and the LRN trained in this scheme takes only about a sixth of the HDNN's inference time and consumes only 472.3KB for storage, with a slight accuracy decrease compared with the large HDNN.
更多查看译文
关键词
Logic gates,Convolution,Kernel,Modulation,Training,Machine learning,Computational modeling,Automatic modulation classification,cross model deep learning,layered Resnet network
AI 理解论文
溯源树
样例
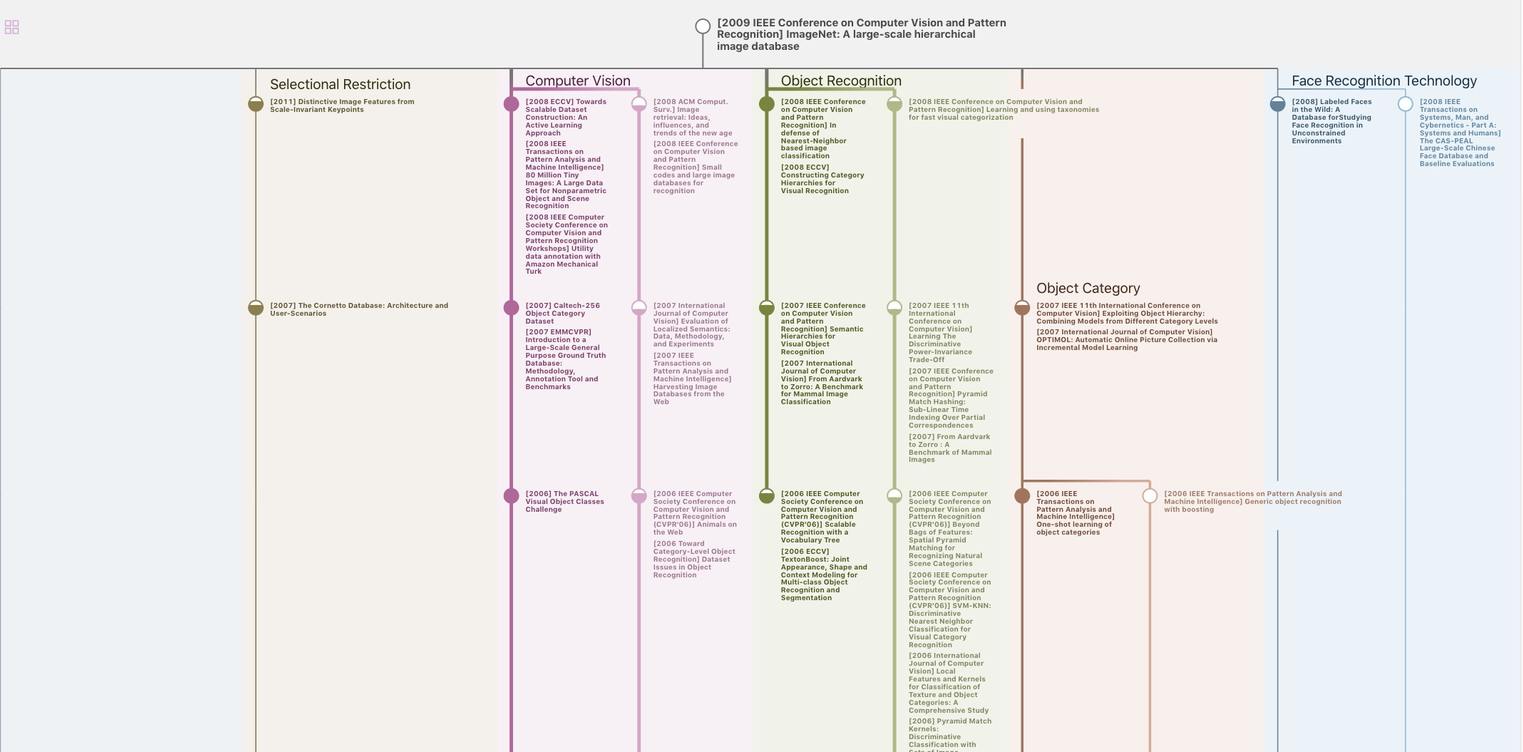
生成溯源树,研究论文发展脉络
Chat Paper
正在生成论文摘要