Development and internal validation of a clinical prediction model for non-recovery in older adults with back pain
OSTEOARTHRITIS AND CARTILAGE(2020)
摘要
Purpose: Back pain is the leading cause of disability globally and it is highly prevalent in older adults. In this population, spinal osteoarthritis (OA) may be a primary cause of back complaints. Nevertheless, evidence on prognostic factors in older adults with back pain in primary care is limited, and no clinical prediction models have been developed for this population. The aim of this study was to develop clinical prediction models for non-recovery in older adults presenting with a new episode of back pain. Methods: Data on adults ≥ 55 years included in the prospective BACE cohort study in general practice in The Netherlands was used. Three definitions of non-recovery were used combining follow-up data at 6- and 12-month follow-up: 1) back pain intensity ≥ 3/10 points on a 0-10 Numerical Rating Scale (NRS); 2) disability ≥ 4/24 points on the Roland-Morris Disability Questionnaire (RMDQ); 3) a dichotomized Global Perceived Effect (GPE) scale. The maximum number of potential predictors was calculated according to the approach proposed by Riley et al. Predictors were selected based on consistent prognostic factors in the back pain literature, prognostic factors found in individual studies in older adults with back pain, and factors deemed potentially clinically relevant. Multiple imputation was performed to impute missing baseline and outcome data. Multivariable logistic regression analysis with a backward selection procedure (p-value > 0.157 for removal) was used to estimate the prediction models. These analyses were repeated for the three previously defined outcome variables. Models’ performance was evaluated with explained variance (Nagelkerke’s R2), calibration (Hosmer-Lemeshow test), and discrimination [Area Under the Curve (AUC)] measures. Calibration and discrimination were considered adequate if the Hosmer-Lemeshow p-value was < 0.05 and the AUC was ≥ 0.75, respectively. To correct for over-optimism of the final models, these were internally validated in 250 bootstrapped samples. Results: 675 patients were included. Mean age was 66.4 (± 7.6) years, 59% were female, 86% had a lifetime history of back pain, and 77% reported a musculoskeletal comorbidity. Baseline pain intensity on the 0-10 NRS was equal to 5.2 (±2.7) and baseline disability on the 0-24 RMDQ was 9.8 (±5.8). A maximum of 14 potential predictors could be included in the models. For each outcome, a prediction model with adequate performance, calibration and discrimination was developed. The model for pain intensity included 8 predictors and displayed an optimism-adjusted AUC of 0.78, exhibiting successful internal validation. The disability model included 13 predictors, it displayed the best performance and was also internally validated (optimism-adjusted AUC = 0.85). The GPE model also displayed adequate internal validation (optimism-adjusted AUC = 0.81) and consisted of 12 predictors. Baseline age, back pain chronicity, pain intensity, disability, recent episodes of back pain, comorbid musculoskeletal pain, pain during rotation, and patient’s expectation to recover were consistent predictors for non-recovery. Morning stiffness longer than 30 minutes, kinesiophobia and pain catastrophizing were predictors in the disability and GPE models. Leg pain and depression were included only in the disability model, while sex was included only in the GPE model. Conclusions: This study presented the development and internal validation of three clinical prediction models for non-recovery in older adults with back pain. These models have potential to help primary care clinicians to determine which patients may not recover from a back pain episode. However, before being implemented in clinical practice, these models should be externally validated in a similar population, and an impact analysis should be conducted.
更多查看译文
关键词
back pain,clinical prediction model,non-recovery
AI 理解论文
溯源树
样例
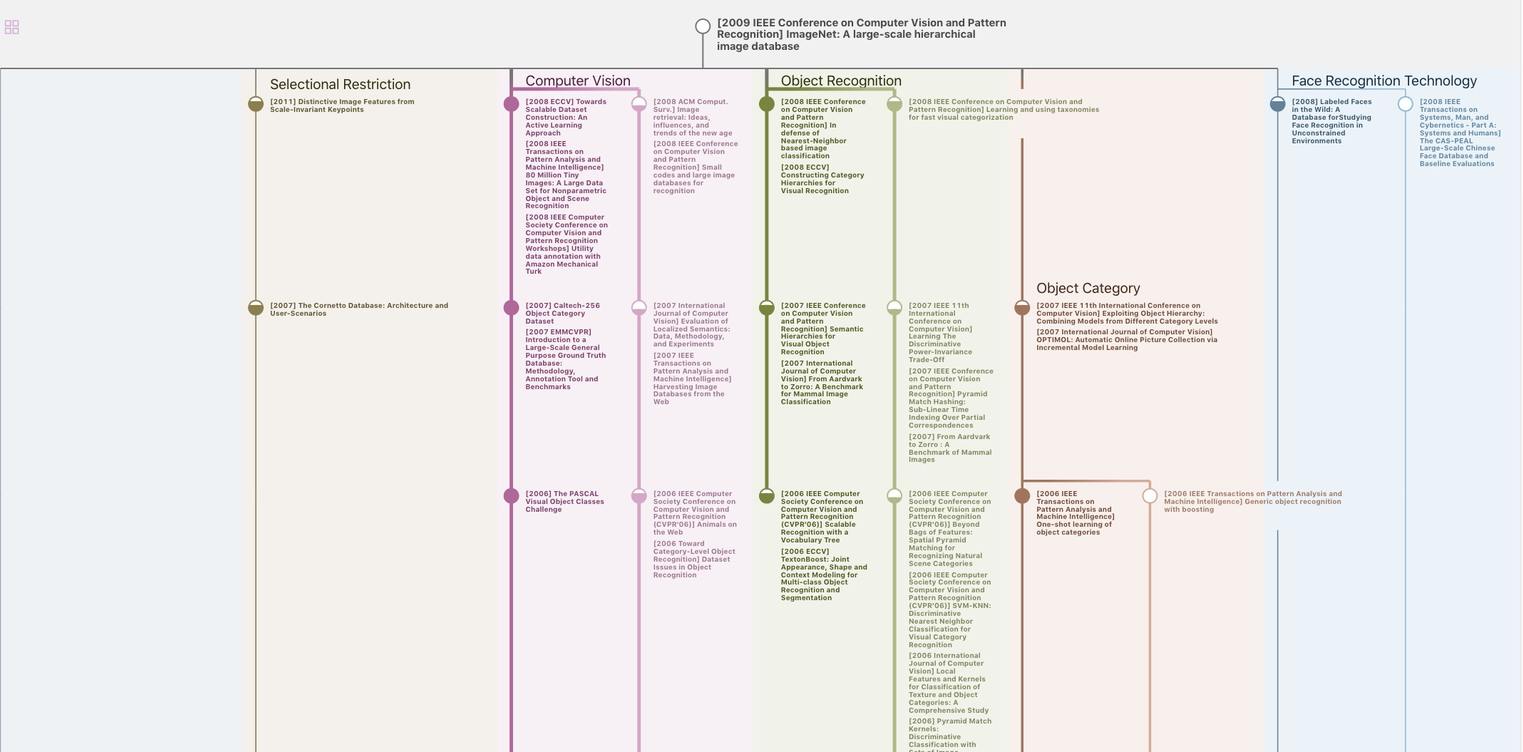
生成溯源树,研究论文发展脉络
Chat Paper
正在生成论文摘要