Deep mean shift clustering
user-5d54d98b530c705f51c2fe5a(2019)
摘要
We use Mean Shift clustering in the latent space of an auto-encoder to have a better representation of the data and a more structured latent space. Instead of just using the mode of the distribution calculated using kernel density estimates, we use trajectories of data points leading to the modes to better model the basin of attraction of each mode. This helps in better structuring of the latent space and results in a more inferential model. Since mean-shift can be modeled as an RNN-block [10] our method is end-to-end trainable. Tuning the bandwidth of mean-shift gives us the the flexibility of clustering the latent space on different hierarchical levels.
更多查看译文
AI 理解论文
溯源树
样例
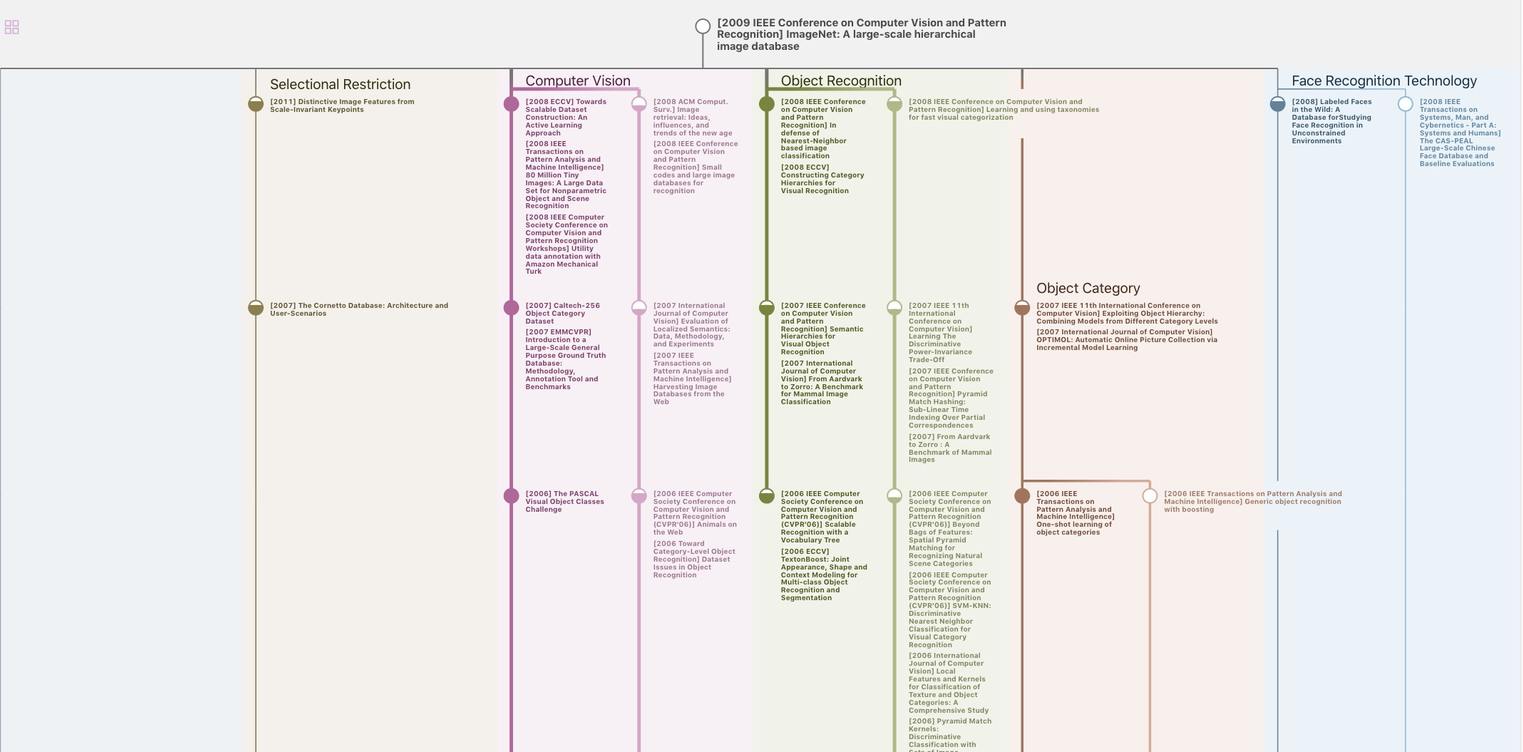
生成溯源树,研究论文发展脉络
Chat Paper
正在生成论文摘要