Learning Unsupervised Hierarchical Part Decomposition Of 3d Objects From A Single Rgb Image
2020 IEEE/CVF CONFERENCE ON COMPUTER VISION AND PATTERN RECOGNITION (CVPR)(2020)
摘要
Humans perceive the 3D world as a set of distinct objects that are characterized by various low-level (geometry, reflectance) and high-level (connectivity, adjacency, symmetry) properties. Recent methods based on convolutional neural networks (CNNs) demonstrated impressive progress in 3D reconstruction, even when using a single 2D image as input. However, the majority of these methods focuses on recovering the local 3D geometry of an object without considering its part-based decomposition or relations between parts. We address this challenging problem by proposing a novel formulation that allows to jointly recover the geometry of a 3D object as a set of primitives as well as their latent hierarchical structure without part-level supervision. Our model recovers the higher level structural decomposition of various objects in the form of a binary tree of primitives, where simple parts are represented with fewer primitives and more complex parts are modeled with more components. Our experiments on the ShapeNet and D-FAUST datasets demonstrate that considering the organization of parts indeed facilitates reasoning about 3D geometry.
更多查看译文
关键词
unsupervised hierarchical part decomposition,3d objects
AI 理解论文
溯源树
样例
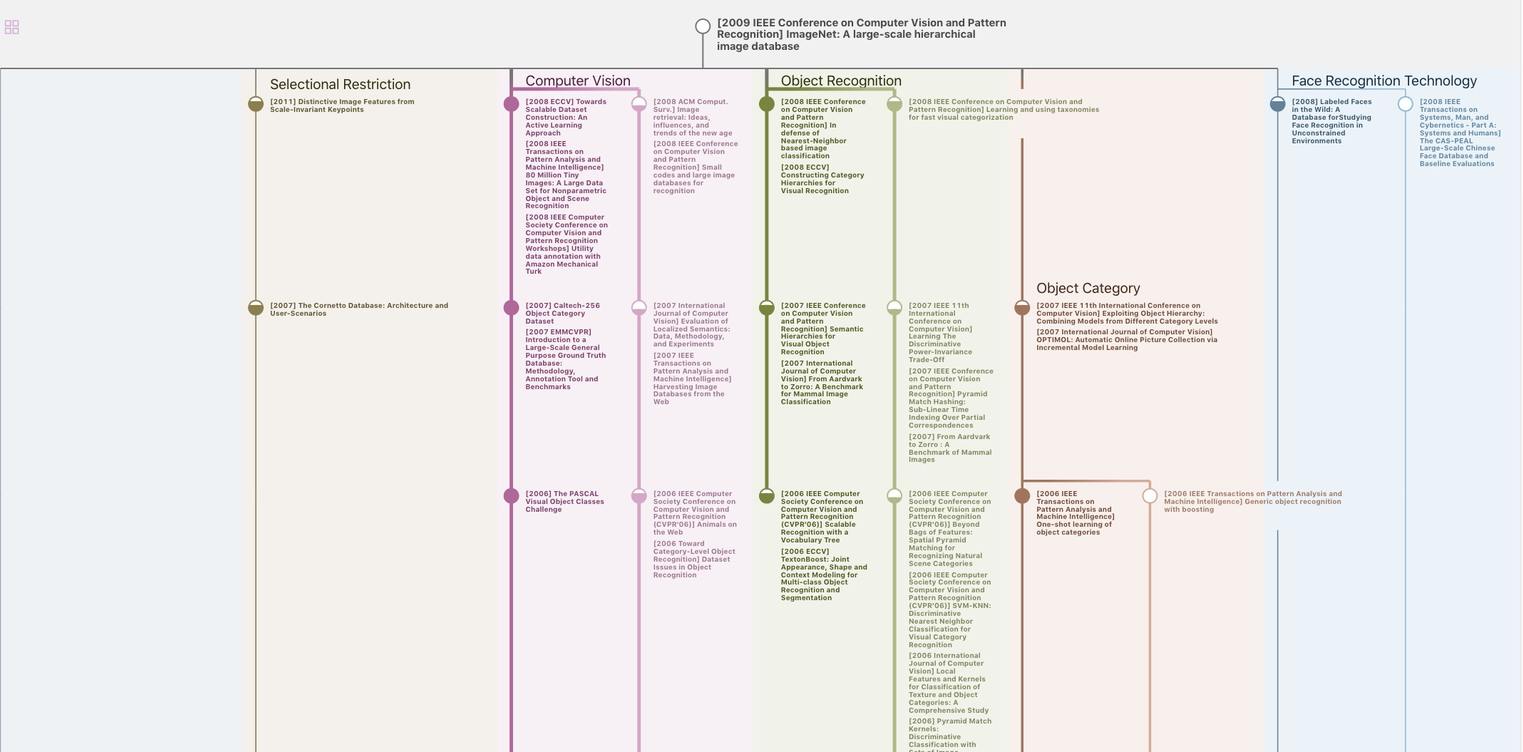
生成溯源树,研究论文发展脉络
Chat Paper
正在生成论文摘要