Dunit: Detection-Based Unsupervised Image-To-Image Translation
2020 IEEE/CVF CONFERENCE ON COMPUTER VISION AND PATTERN RECOGNITION (CVPR)(2020)
摘要
Image-to-image translation has made great strides in recent years, with current techniques being able to handle unpaired training images and to account for the multimodality of the translation problem. Despite this, most methods treat the image as a whole, which makes the results they produce for content-rich scenes less realistic. In this paper, we introduce a Detection-based Unsupervised Image-to-image Translation (DUNIT) approach that explicitly accounts for the object instances in the translation process. To this end, we extract separate representations for the global image and for the instances, which we then fuse into a common representation from which we generate the translated image. This allows us to preserve the detailed content of object instances, while still modeling the fact that we aim to produce an image of a single consistent scene. We introduce an instance consistency loss to maintain the coherence between the detections. Furthermore, by incorporating a detector into our architecture, we can still exploit object instances at test time. As evidenced by our experiments, this allows us to outperform the state-of-the-art unsupervised image-to-image translation methods. Furthermore, our approach can also be used as an unsupervised domain adaptation strategy for object detection, and it also achieves state-of-the-art performance on this task.
更多查看译文
关键词
global image,translated image,object instances,unpaired training images,translation problem,image-to-image translation approach,translation process,detection-based unsupervised image-to-image translation,DUNIT
AI 理解论文
溯源树
样例
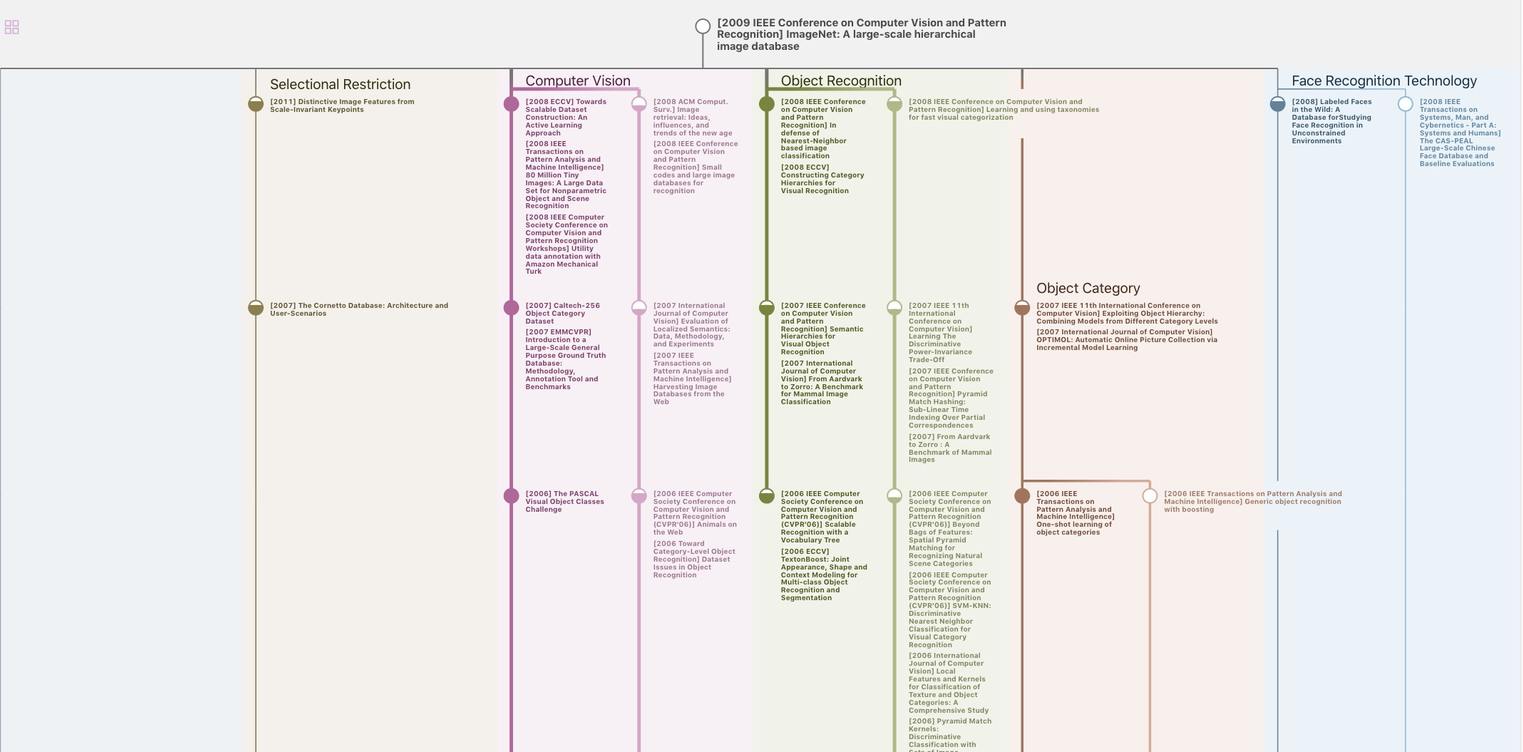
生成溯源树,研究论文发展脉络
Chat Paper
正在生成论文摘要