Transmomo: Invariance-Driven Unsupervised Video Motion Retargeting
2020 IEEE/CVF CONFERENCE ON COMPUTER VISION AND PATTERN RECOGNITION (CVPR)(2020)
摘要
We present a lightweight video motion retargeting approach TransMoMo that is capable of transferring motion of a person in a source video realistically to another video of a target person (Fig. I). Without using any paired data for supervision, the proposed method can be trained in an unsupervised manner by exploiting invariance properties of three orthogonal factors of variation including motion, structure, and view-angle. Specifically, with loss functions carefully derived based on invariance, we train an autoencoder to disentangle the latent representations of such factors given the source and target video clips. This allows us to selectively transfer motion extracted from the source video seamlessly to the target video in spite of structural and view-angle disparities between the source and the target. The relaxed assumption of paired data allows our method to be trained on a vast amount of videos needless of manual annotation of source-target pairing, leading to improved robustness against large structural variations and extreme motion in videos. We demonstrate the effectiveness of our method over the state-of-the-art methods such as NKN [39], EDN [7] and LCM [3]. Code, model and data are publicly available on our project page.(1)
更多查看译文
关键词
target video clips,paired data,source-target pairing,structural variations,extreme motion,invariance-driven unsupervised video motion retargeting,lightweight video motion retargeting approach TransMoMo,target person,orthogonal factors,loss functions,auto-encoder,latent representations,source video clips,structural view-angle disparities
AI 理解论文
溯源树
样例
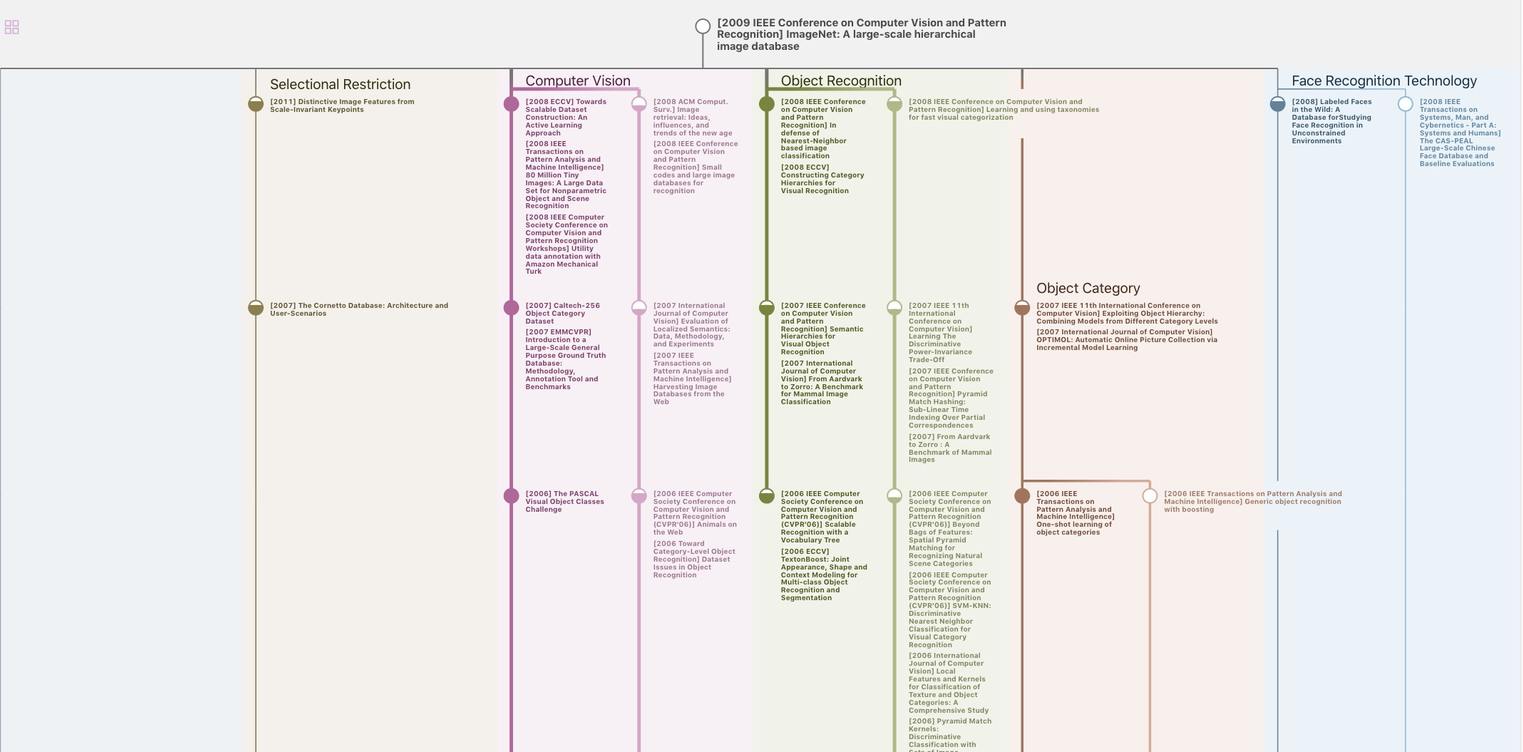
生成溯源树,研究论文发展脉络
Chat Paper
正在生成论文摘要