Decoupled Representation Learning For Skeleton-Based Gesture Recognition
2020 IEEE/CVF CONFERENCE ON COMPUTER VISION AND PATTERN RECOGNITION (CVPR)(2020)
摘要
Skeleton-based gesture recognition is very challenging, as the high-level information in gesture is expressed by a sequence of complexly composite motions. Previous works often learn all the motions with a single model. In this paper, we propose to decouple the gesture into hand posture variations and hand movements, which are then modeled separately. For the former, the skeleton sequence is embedded into a 3D hand posture evolution volume (HPEV) to represent fine-grained posture variations. For the latter, the shifts of hand center and fingertips are arranged as a 2D hand movement map (HMM) to capture holistic movements. To learn from the two inhomogeneous representations for gesture recognition, we propose an end-to-end two-stream network. The HPEV stream integrates both spatial layout and temporal evolution information of hand postures by a dedicated 3D CNN, while the HMM stream develops an efficient 21) CNN to extract hand movement features. Eventually, the predictions of the two streams are aggregated with high efficiency. Extensive experiments on SHREC' 17 Track, DHG-14/28 and FPHA datasets demonstrate that our method is competitive with the state-of-the-art.
更多查看译文
关键词
complexly composite motions,hand posture variations,hand movements,skeleton sequence,3D hand posture evolution volume,fine-grained posture variations,hand center,2D hand movement map,holistic movements,end-to-end two-stream network,temporal evolution information,hand postures,hand movement features,decoupled representation learning,skeleton-based gesture recognition,high-level information
AI 理解论文
溯源树
样例
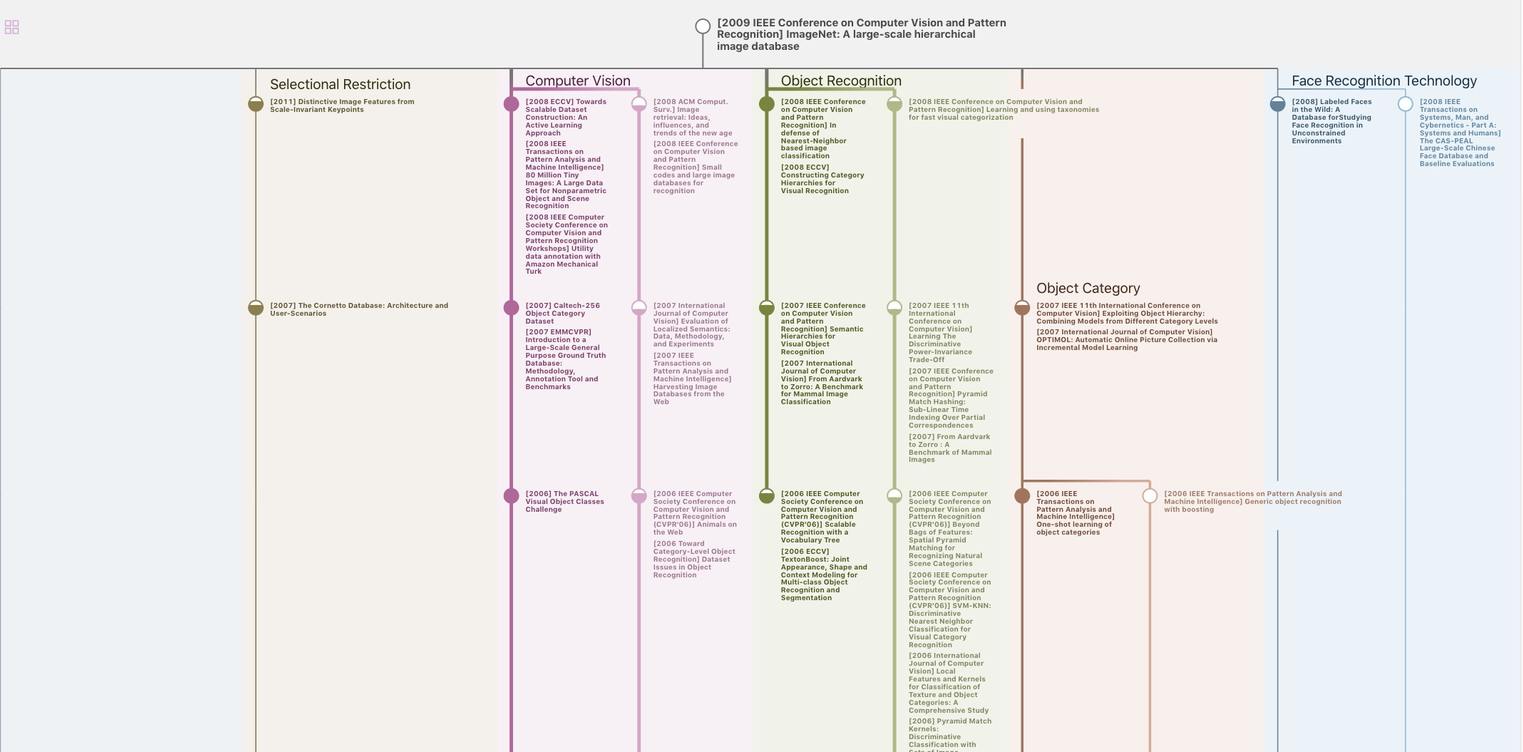
生成溯源树,研究论文发展脉络
Chat Paper
正在生成论文摘要