Relative Interior Rule in Block-Coordinate Descent
CVPR(2020)
摘要
It is well-known that for general convex optimization problems, block-coordinate descent can get stuck in poor local optima. Despite that, versions of this method known as convergent message passing are very successful to approximately solve the dual LP relaxation of the MAP inference problem in graphical models. In attempt to identify the reason why these methods often achieve good local minima, we argue that if in block-coordinate descent the set of minimizers over a variable block has multiple elements, one should choose an element from the relative interior of this set. We show that this rule is not worse than any other rule for choosing block-minimizers. Based on this observation, we develop a theoretical framework for block-coordinate descent applied to general convex problems. We illustrate this theory on convergent message-passing methods.
更多查看译文
关键词
block-coordinate descent,variable block,block-minimizers,general convex problems,convergent message-passing methods,relative interior rule,general convex optimization problems,dual LP relaxation,MAP inference problem,graphical models
AI 理解论文
溯源树
样例
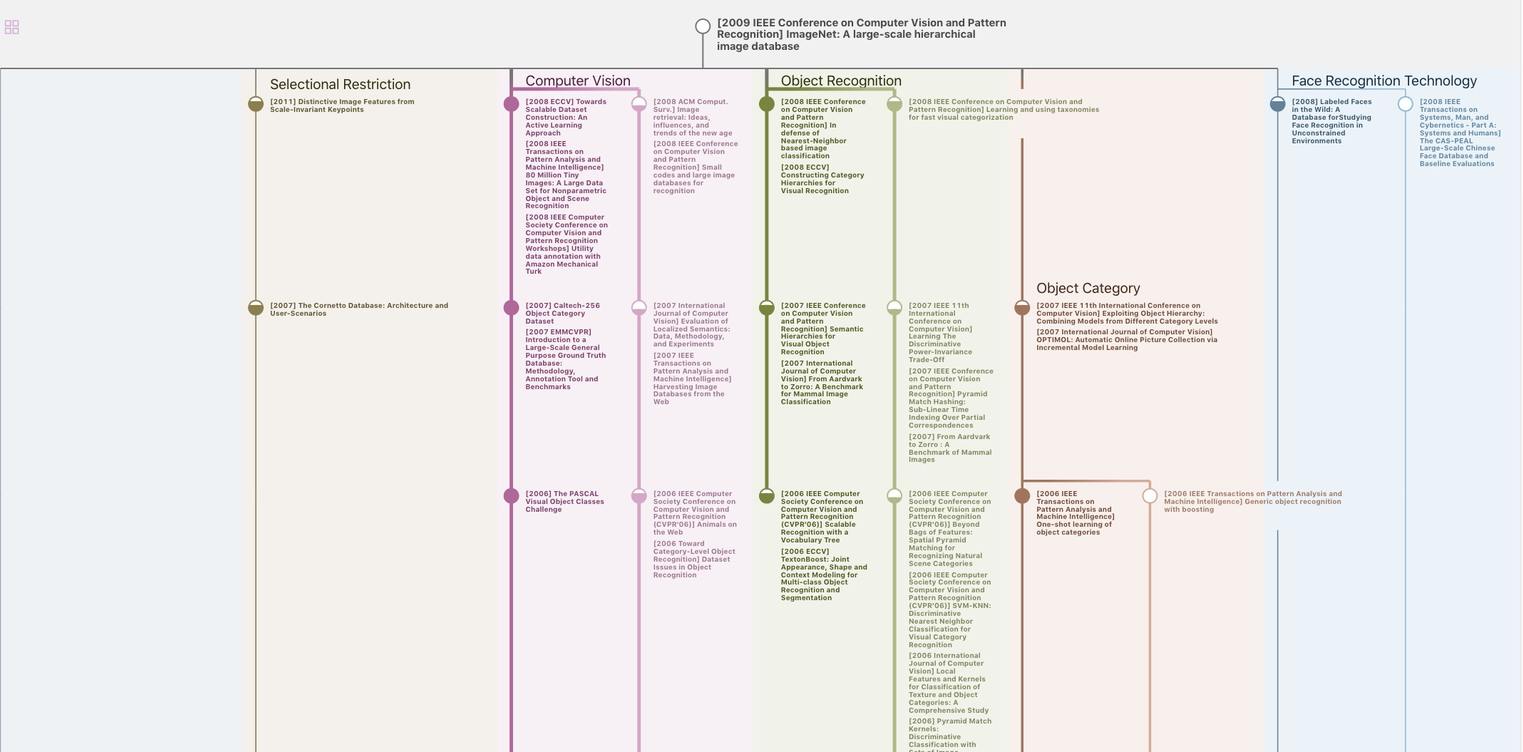
生成溯源树,研究论文发展脉络
Chat Paper
正在生成论文摘要