Exploring Data Aggregation in Policy Learning for Vision-Based Urban Autonomous Driving
CVPR(2020)
摘要
Data aggregation techniques can significantly improve vision-based policy learning within a training environment, e.g., learning to drive in a specific simulation condition. However, as on-policy data is sequentially sampled and added in an iterative manner, the policy can specialize and overfit to the training conditions. For real-world applications, it is useful for the learned policy to generalize to novel scenarios that differ from the training conditions. To improve policy learning while maintaining robustness when training end-to-end driving policies, we perform an extensive analysis of data aggregation techniques in the CARLA environment. We demonstrate how the majority of them have poor generalization performance, and develop a novel approach with empirically better generalization performance compared to existing techniques. Our two key ideas are (1) to sample critical states from the collected on-policy data based on the utility they provide to the learned policy in terms of driving behavior, and (2) to incorporate a replay buffer which progressively focuses on the high uncertainty regions of the policy\u0027s state distribution. We evaluate the proposed approach on the CARLA NoCrash benchmark, focusing on the most challenging driving scenarios with dense pedestrian and vehicle traffic. Our approach improves driving success rate by 16% over state-of-the-art, achieving 87% of the expert performance while also reducing the collision rate by an order of magnitude without the use of any additional modality, auxiliary tasks, architectural modifications or reward from the environment.
更多查看译文
关键词
vision-based urban autonomous driving,data aggregation techniques,vision-based policy learning,training environment,specific simulation condition,on-policy data,training conditions,learned policy,CARLA environment,empirically better generalization performance,sample critical states,dense pedestrian,vehicle traffic,driving success rate,collision rate,end-to-end driving policies,CARLA NoCrash benchmark,policy state distribution
AI 理解论文
溯源树
样例
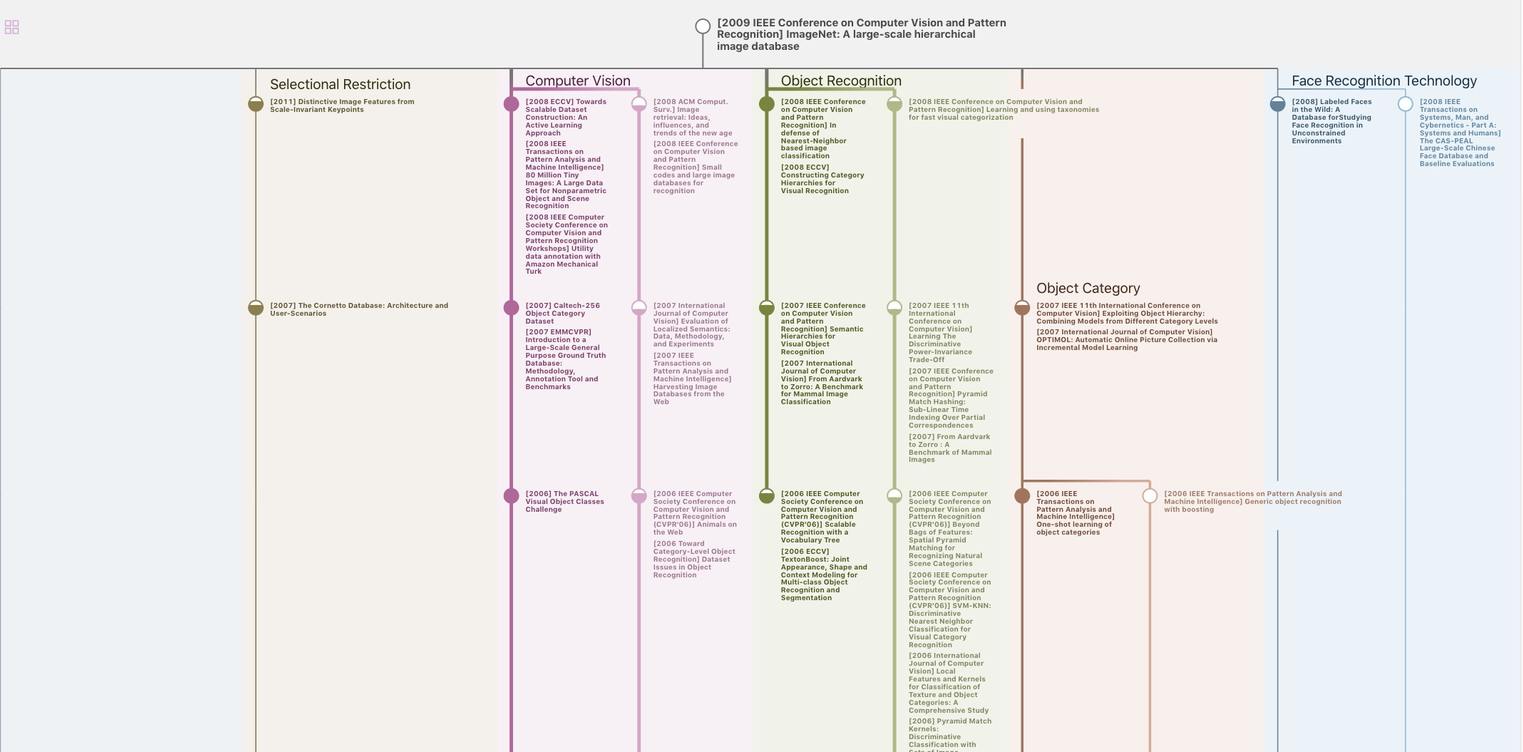
生成溯源树,研究论文发展脉络
Chat Paper
正在生成论文摘要