Imitative Non-Autoregressive Modeling for Trajectory Forecasting and Imputation
CVPR(2020)
摘要
Trajectory forecasting and imputation are pivotal steps towards understanding the movement of human and objects, which are quite challenging since the future trajectories and missing values in a temporal sequence are full of uncertainties, and the spatial-temporally contextual correlation is hard to model. Yet, the relevance between sequence prediction and imputation is disregarded by existing approaches. To this end, we propose a novel imitative non-autoregressive modeling method to simultaneously handle the trajectory prediction task and the missing value imputation task. Specifically, our framework adopts an imitation learning paradigm, which contains a recurrent conditional variational autoencoder (RC-VAE) as a demonstrator, and a non-autoregressive transformation model (NART) as a learner. By jointly optimizing the two models, RC-VAE can predict the future trajectory and capture the temporal relationship in the sequence to supervise the NART learner. As a result, NART learns from the demonstrator and imputes the missing value in a non autoregressive strategy. We conduct extensive experiments on three popular datasets, and the results show that our model achieves state-of-the-art performance across all the datasets.
更多查看译文
关键词
temporal relationship,NART learner,nonautoregressive strategy,trajectory forecasting,pivotal steps,human objects,temporal sequence,spatial-temporally contextual correlation,sequence prediction,trajectory prediction task,missing value imputation task,imitation learning paradigm,recurrent conditional variational autoencoder,RC-VAE,nonautoregressive transformation model,imitative nonautoregressive modeling
AI 理解论文
溯源树
样例
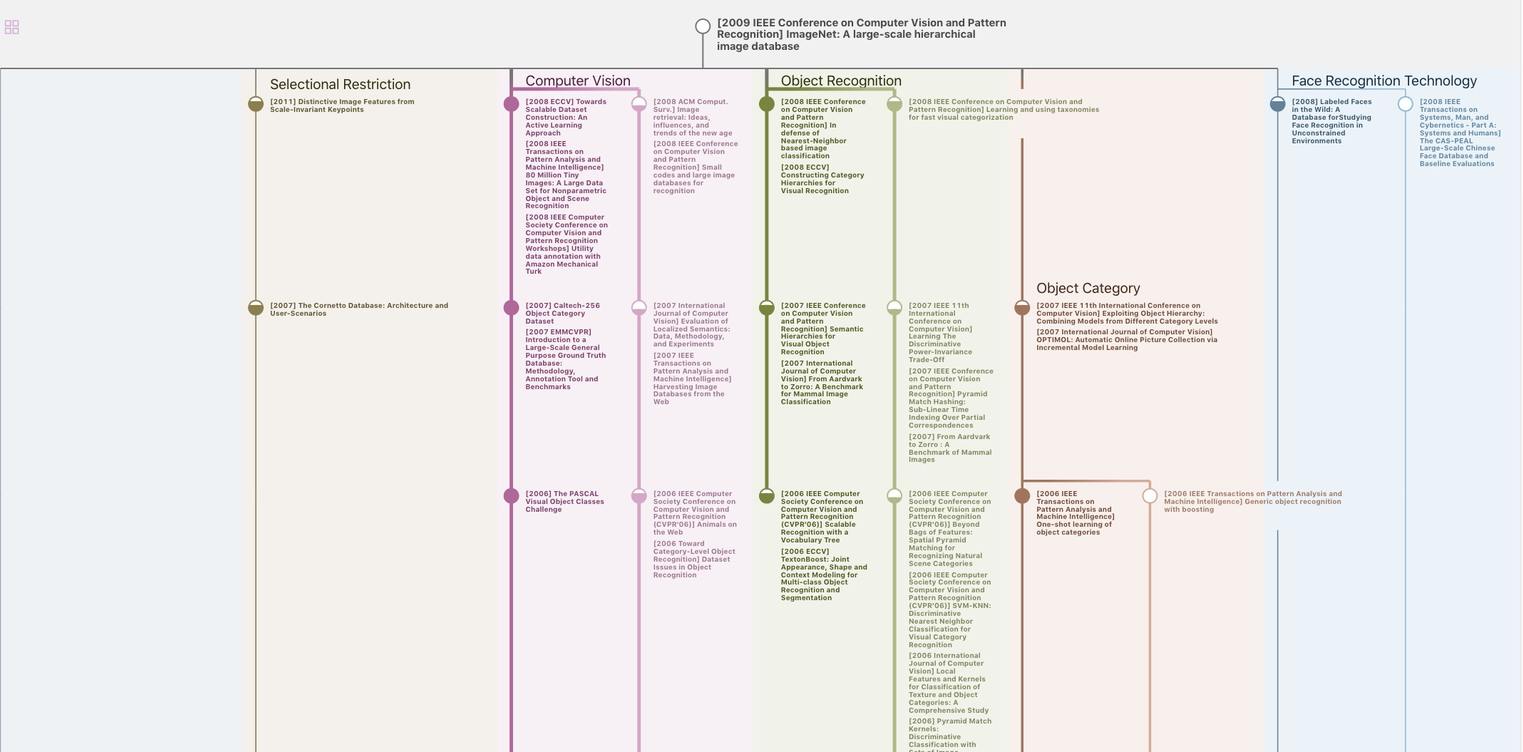
生成溯源树,研究论文发展脉络
Chat Paper
正在生成论文摘要