Reliability and Performance Assessment of Federated Learning on Clinical Benchmark Data
arxiv(2020)
摘要
As deep learning have been applied in a clinical context, privacy concerns have increased because of the collection and processing of a large amount of personal data. Recently, federated learning (FL) has been suggested to protect personal privacy because it does not centralize data during the training phase. In this study, we assessed the reliability and performance of FL on benchmark datasets including MNIST and MIMIC-III. In addition, we attempted to verify FL on datasets that simulated a realistic clinical data distribution. We implemented FL that uses a client and server architecture and tested client and server FL on modified MNIST and MIMIC-III datasets. FL delivered reliable performance on both imbalanced and extremely skewed distributions (i.e., the difference of the number of patients and the characteristics of patients in each hospital). Therefore, FL can be suitable to protect privacy when applied to medical data.
更多查看译文
关键词
federated learning,clinical benchmark data,performance assessment
AI 理解论文
溯源树
样例
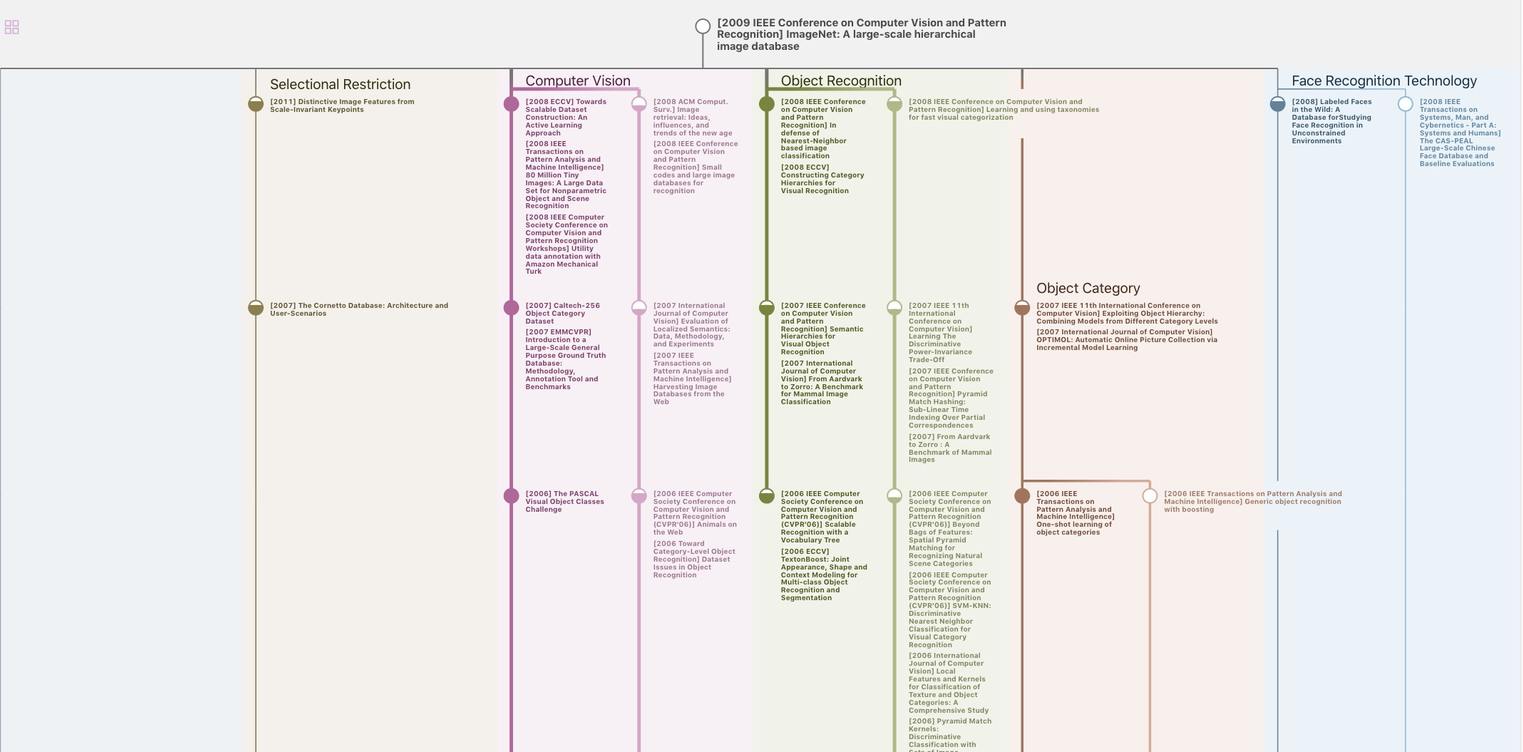
生成溯源树,研究论文发展脉络
Chat Paper
正在生成论文摘要