Reactive Sample Size for Heuristic Search in Simulation-based Optimization
arxiv(2020)
摘要
In simulation-based optimization, the optimal setting of the input parameters of the objective function can be determined by heuristic optimization techniques. However, when simulators model the stochasticity of real-world problems, their output is a random variable and multiple evaluations of the objective function are necessary to properly compare the expected performance of different parameter settings. This paper presents a novel reactive sample size algorithm based on parametric tests and indifference-zone selection, which can be used for improving the efficiency and robustness of heuristic optimization methods. The algorithm reactively decides, in an online manner, the sample size to be used for each comparison during the optimization according to observed statistical evidence. Tests employ benchmark functions extended with artificial levels of noise and a simulation-based optimization tool for hotel revenue management. Experimental results show that the reactive method can improve the efficiency and robustness of simulation-based optimization techniques.
更多查看译文
关键词
heuristic search,reactive sample size,optimization,simulation-based
AI 理解论文
溯源树
样例
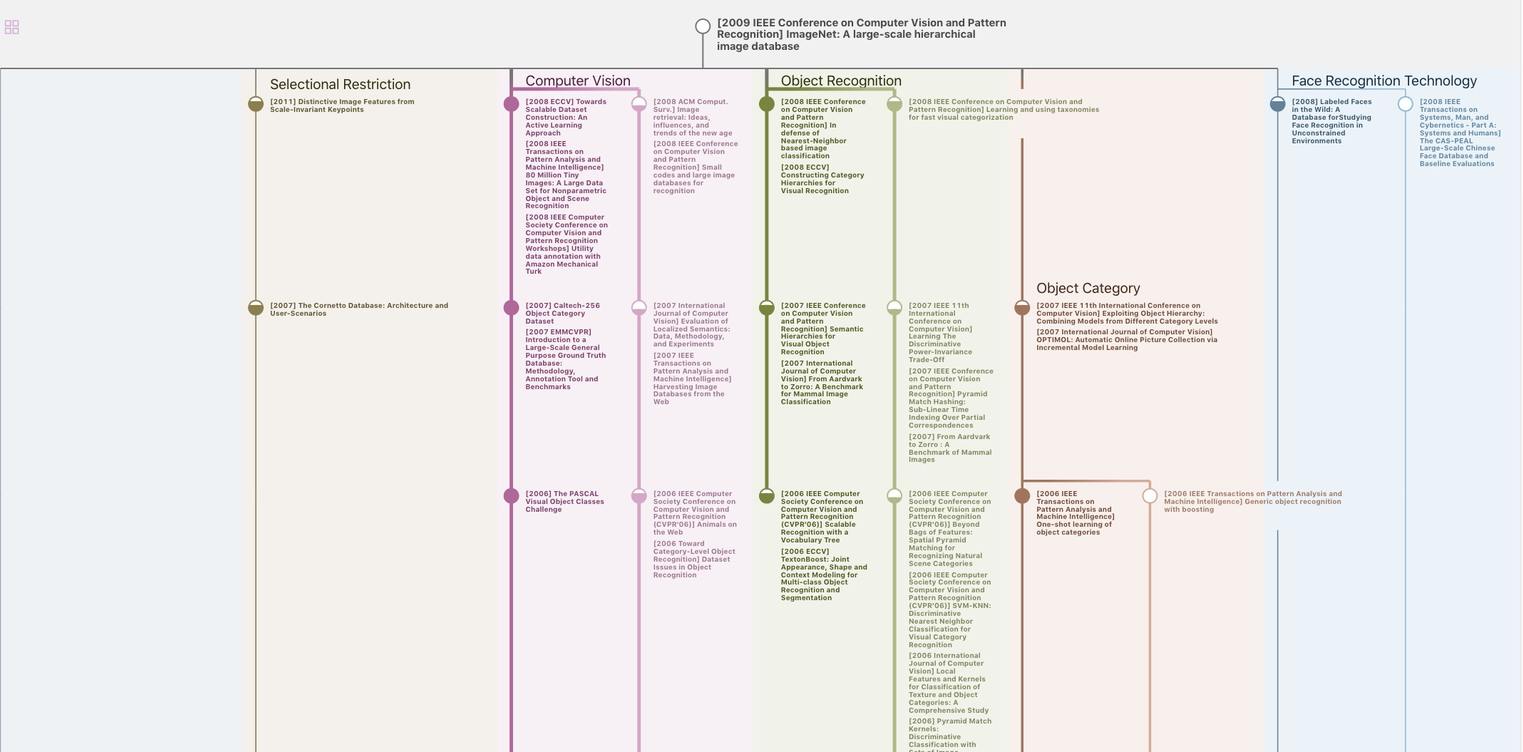
生成溯源树,研究论文发展脉络
Chat Paper
正在生成论文摘要