End-To-End Auditory Object Recognition Via Inception Nucleus
ICASSP 2020 - 2020 IEEE International Conference on Acoustics, Speech and Signal Processing (ICASSP)(2020)
摘要
Machine learning approaches to auditory object recognition are traditionally based on engineered features such as those derived from the spectrum or cepstrum. More recently, end- to-end classification systems in image and auditory recognition systems have been developed to learn features jointly with classification and result in improved classification accuracy. In this paper, we propose a novel end-to-end deep neural network to map the raw waveform inputs to sound class labels. Our network includes an "inception nucleus" that optimizes the size of convolutional filters on the fly that results in reducing engineering efforts dramatically. Classification results compared favorably against current state-of-the-art approaches, besting them by 10.4 percentage points on the Ur- bansound8k dataset. Analyses of learned representations revealed that filters in the earlier hidden layers learned wavelet like transforms to extract features that were informative for classification.
更多查看译文
关键词
End-to-End Learning,Auditory Object Recognition,Inception Nucleus,Deep Convolutional Neural Networks,Sound Event Classification
AI 理解论文
溯源树
样例
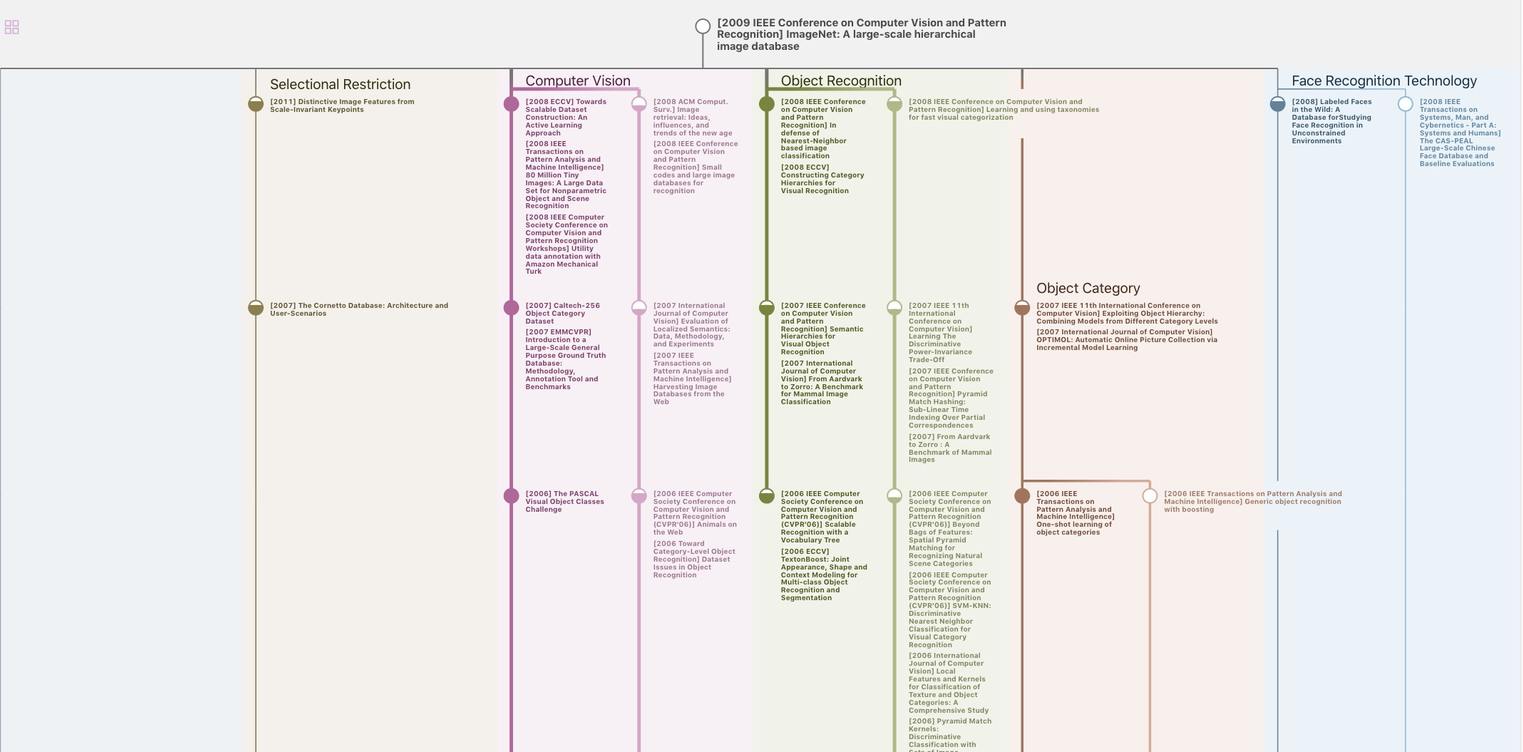
生成溯源树,研究论文发展脉络
Chat Paper
正在生成论文摘要