Exploring Optimal DNN Architecture for End-to-End Beamformers Based on Time-frequency References
arxiv(2020)
摘要
Acoustic beamformers have been widely used to enhance audio signals. Currently, the best methods are the deep neural network (DNN)-powered variants of the generalized eigenvalue and minimum-variance distortionless response beamformers and the DNN-based filter-estimation methods that are used to directly compute beamforming filters. Both approaches are effective; however, they have blind spots in their generalizability. Therefore, we propose a novel approach for combining these two methods into a single framework that attempts to exploit the best features of both. The resulting model, called the W-Net beamformer, includes two components; the first computes time-frequency references that the second uses to estimate beamforming filters. The results on data that include a wide variety of room and noise conditions, including static and mobile noise sources, show that the proposed beamformer outperforms other methods on all tested evaluation metrics, which signifies that the proposed architecture allows for effective computation of the beamforming filters.
更多查看译文
关键词
optimal dnn architecture,end-to-end,time-frequency
AI 理解论文
溯源树
样例
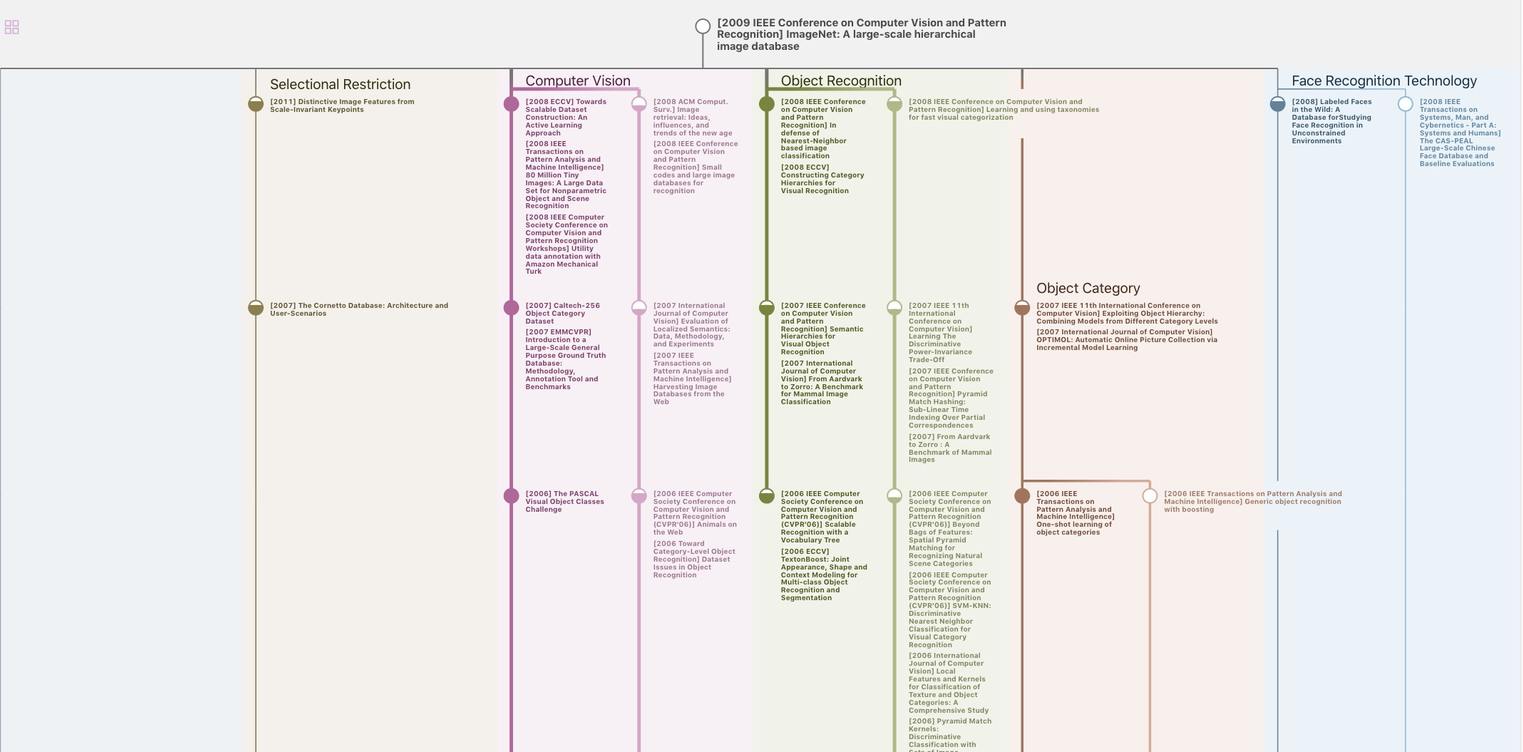
生成溯源树,研究论文发展脉络
Chat Paper
正在生成论文摘要