Revealing Mobility Regularities in Urban Rail Systems
Procedia computer science(2020)
Abstract
Studying mobility patterns in public transport systems is critical for multiple applications from strategic planning to operations control to information provision. Unveiling and understanding the underlying (unobserved) mechanism governing the generation of (observed) mobility patterns are challenging. Considering the recurrent human travel activities and system operations, typical mobility regularities (or variations) patterns of the system can be captured by travel demand. This paper proposes a new paradigm to investigate the regularity in macroscopic mobility patterns for urban rail applications using concepts from image processing and pattern recognition to identify distinct demand patterns. We analyse the within-day demand patterns and day-to-day comparisons by constructing the spatiotemporal eigen-demand images (faces). The case study showed that the entry demand of Hong Kong metro network over 6 months can be grouped into 5 distinct clusters. This new perspective of eigen-demand allows examining the internal essence of large amounts of mobility patterns over time and reveals a global daily demand pattern at the entire system.
MoreTranslated text
Key words
Pulic transport,eigen-demand,principal component analysis,clustering
AI Read Science
Must-Reading Tree
Example
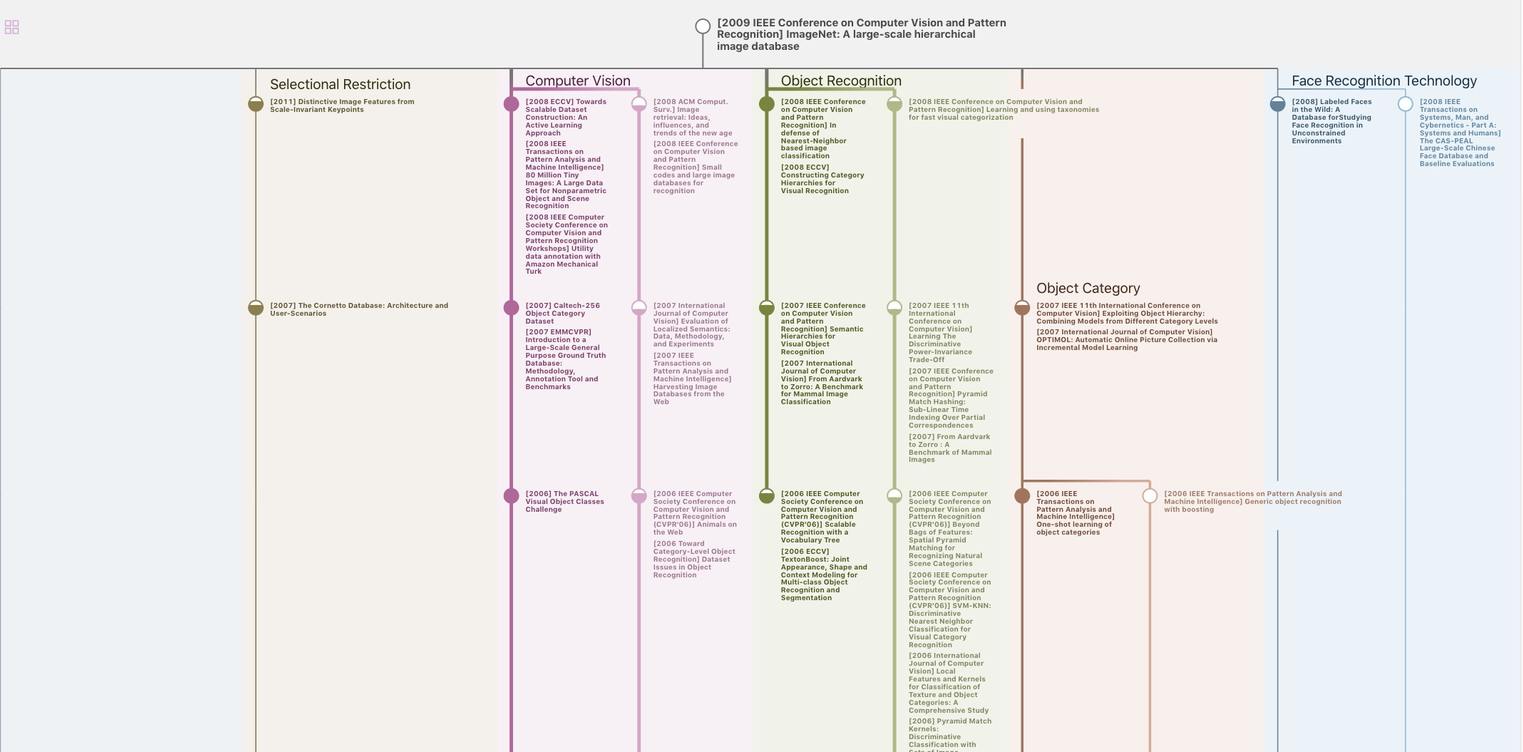
Generate MRT to find the research sequence of this paper
Chat Paper
Summary is being generated by the instructions you defined