Deep Learning Based Multi-Label Chest X-Ray Classification with Entropy Weighting Loss
2019 12th International Symposium on Computational Intelligence and Design (ISCID)(2019)
摘要
With large chest X-ray image datasets open-accessed, it is feasible to build diagnosis system based on deep learning methods. In this work, we formulated chest X-ray diagnosis as multi-label classification problem. However, multi-labels are treated independently in traditional methods and existing loss can easily lose important information for class with fewer cases. We proposed an entropy weighting loss to observe inter-label dependencies and make full use of class whose cases are fewer than others. From the global perspective of all pathological classes, digging relations between all classes, the proposed loss could improve model performance. We experimented with three basic deep learning models (VGG16, ResNet50, DenseNet121) at first, and decided to use DenseNet121 as a baseline model. We evaluated our approach and achieved better results, whose AUC score is 0.8430 on average, under the Chest X-ray14 and its patient-wise split. We demonstrate that our proposed loss can dig relations between different disease labels just as illness complications in reality, and we do not consider every label is independent.
更多查看译文
关键词
Chest X-ray,Multi-label learning,DenseNet,Cross entropy
AI 理解论文
溯源树
样例
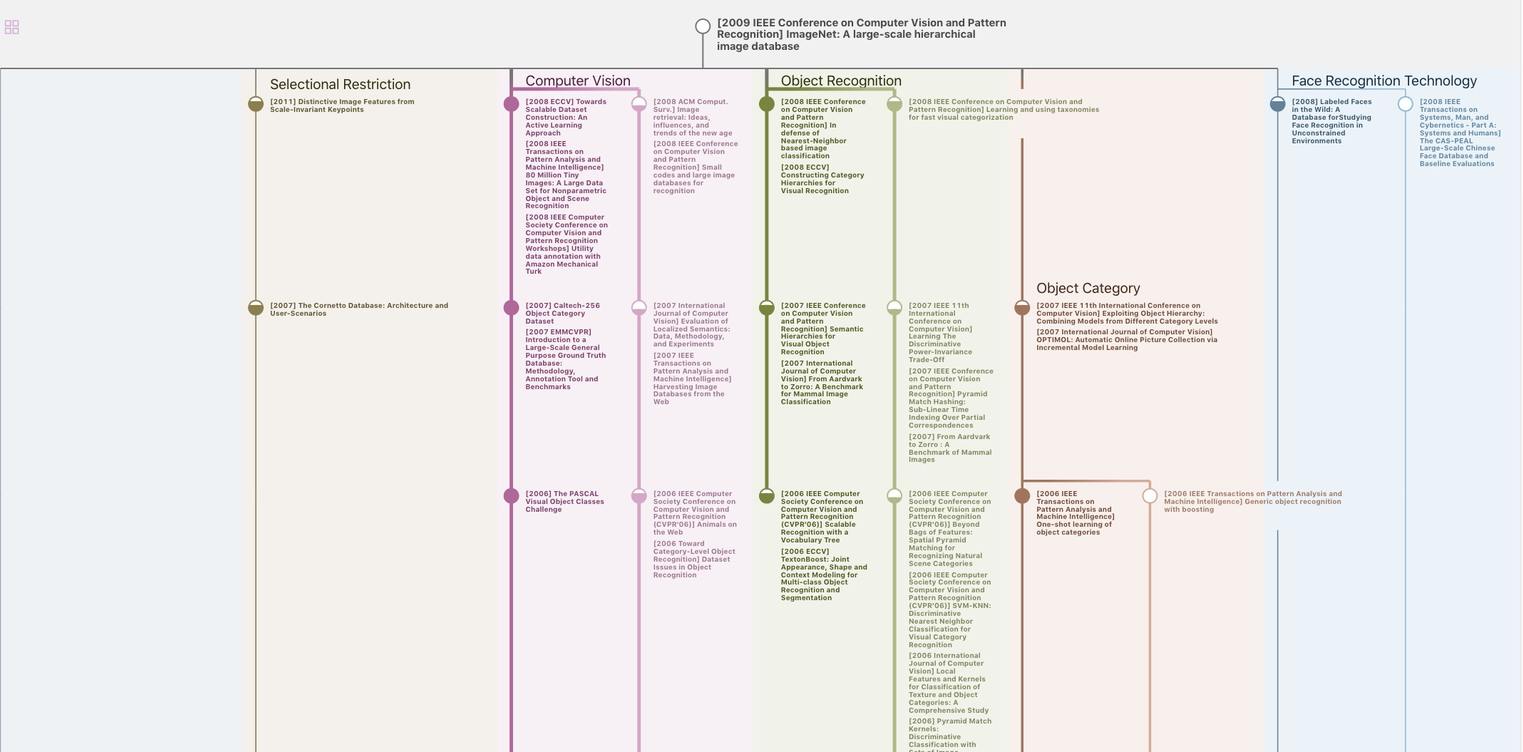
生成溯源树,研究论文发展脉络
Chat Paper
正在生成论文摘要