Spectral Cluster Maps Versus Spectral Clustering.
CISIM(2020)
摘要
The paper investigates several notions of graph Laplacians and graph kernels from the perspective of understanding the graph clustering via the graph embedding into an Euclidean space. We propose hereby a unified view of spectral graph clustering and kernel clustering methods. The various embedding techniques are evaluated from the point of view of clustering stability (with respect to k-means that is the algorithm underpinning the spectral and kernel methods). It is shown that the choice of a fixed number of dimensions may result in clustering instability due to eigenvalue ties. Furthermore, it is shown that kernel methods are less sensitive to the number of used dimensions due to downgrading the impact of less discriminative dimensions.
更多查看译文
关键词
Graph clustering, Spectral clustering, Spectral embedding, Kernel clustering
AI 理解论文
溯源树
样例
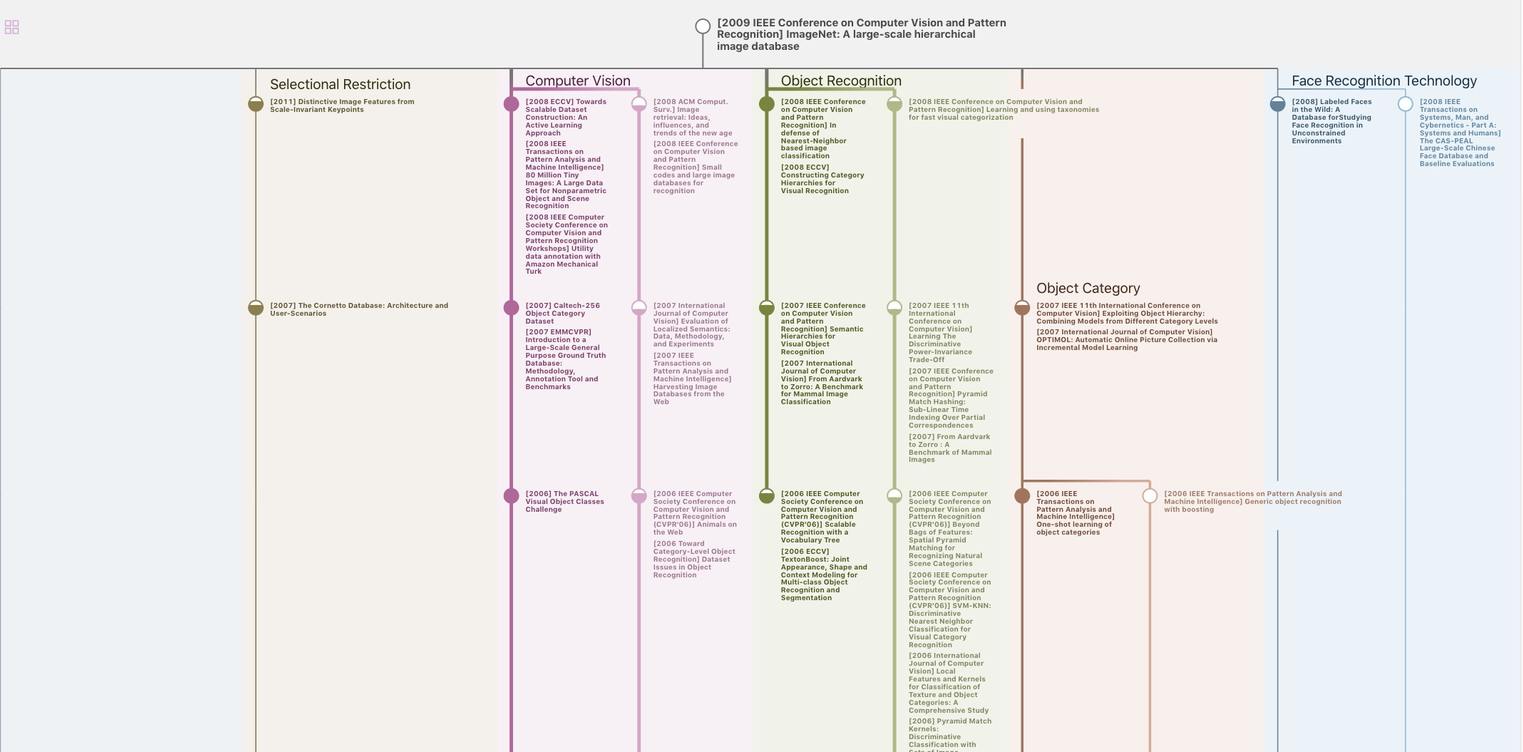
生成溯源树,研究论文发展脉络
Chat Paper
正在生成论文摘要