Non-taxonomic role extraction for biomedical concepts
ESSLLI Student Session(2013)
摘要
Ontologies play a major role in life sciences, enabling a number of applications, from new data integration to knowledge verification. Obtaining formalized knowledge from unstructured data is especially relevant for biomedical domain, since the amount of textual biomedical data has been growing exponentially. In this paper we present an approach of learning Description Logic (DL) roles from unstructured text. We model the task as a supervised learning of formal roles from the SNOMED Clinical Terms (SNOMED CT) ontology using the textual definitions from Medical Subject Headings (MeSH) thesaurus. The evaluation is focused on the three most populated roles in SNOMED CT: Associated Morphology, Finding Site and Causative Agent. Results demonstrate that our methodology may provide an Accuracy of up to 75% and can be extended to model an exhaustive set of roles for biomedical domain.
更多查看译文
AI 理解论文
溯源树
样例
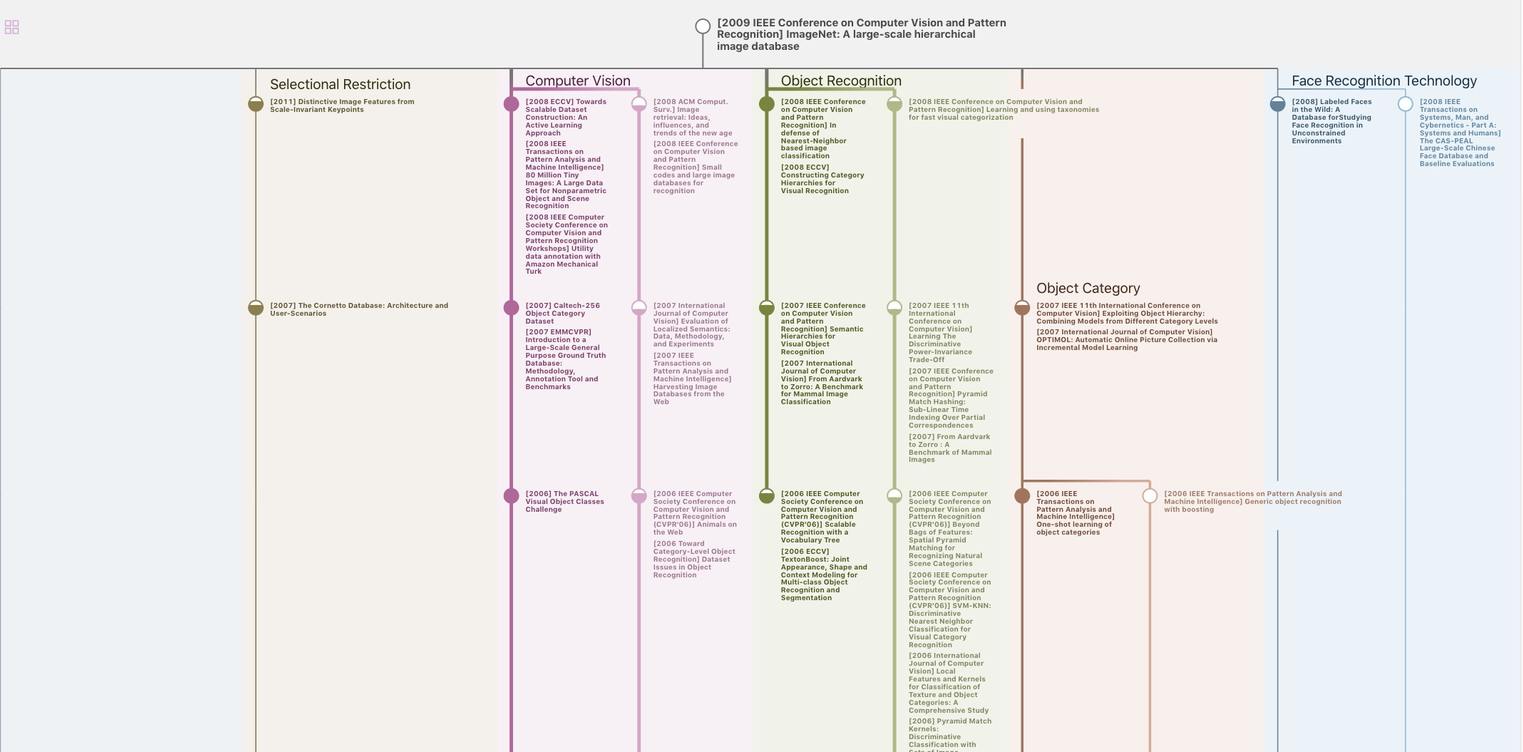
生成溯源树,研究论文发展脉络
Chat Paper
正在生成论文摘要