Improving KantanMT Training Efficiency with fast_align.
AMTA(2016)
摘要
In recent years, statistical machine translation (SMT) has been widely deployed in translators’\r\nworkflow with significant improvement of productivity. However, prior to invoking an SMT\r\nsystem to translate an unknown text, an SMT engine needs to be built. As such, building speed\r\nof the engine is essential for the translation workflow, i.e., the sooner an engine is built, the\r\nsooner it will be exploited.\r\nWith the increase of the computational capabilities of recent technology the building time for\r\nan SMT engine has decreased substantially. For example, cloud-based SMT providers, such as\r\nKantanMT, can built high-quality, ready-to-use, custom SMT engines in less than a couple of\r\ndays. To speed-up furthermore this process we look into optimizing the word alignment process\r\nthat takes place during building the SMT engine. Namely, we substitute the word alignment\r\ntool used by KantanMT pipeline – Giza++ – with a more efficient one, i.e., fast_align.\r\nIn this work we present the design and the implementation of the KantanMT pipeline that uses\r\nfast_align in place of Giza++. We also conduct a comparison between the two word\r\nalignment tools with industry data and report on our findings. Up to our knowledge, such\r\nextensive empirical evaluation of the two tools has not been done before.
更多查看译文
AI 理解论文
溯源树
样例
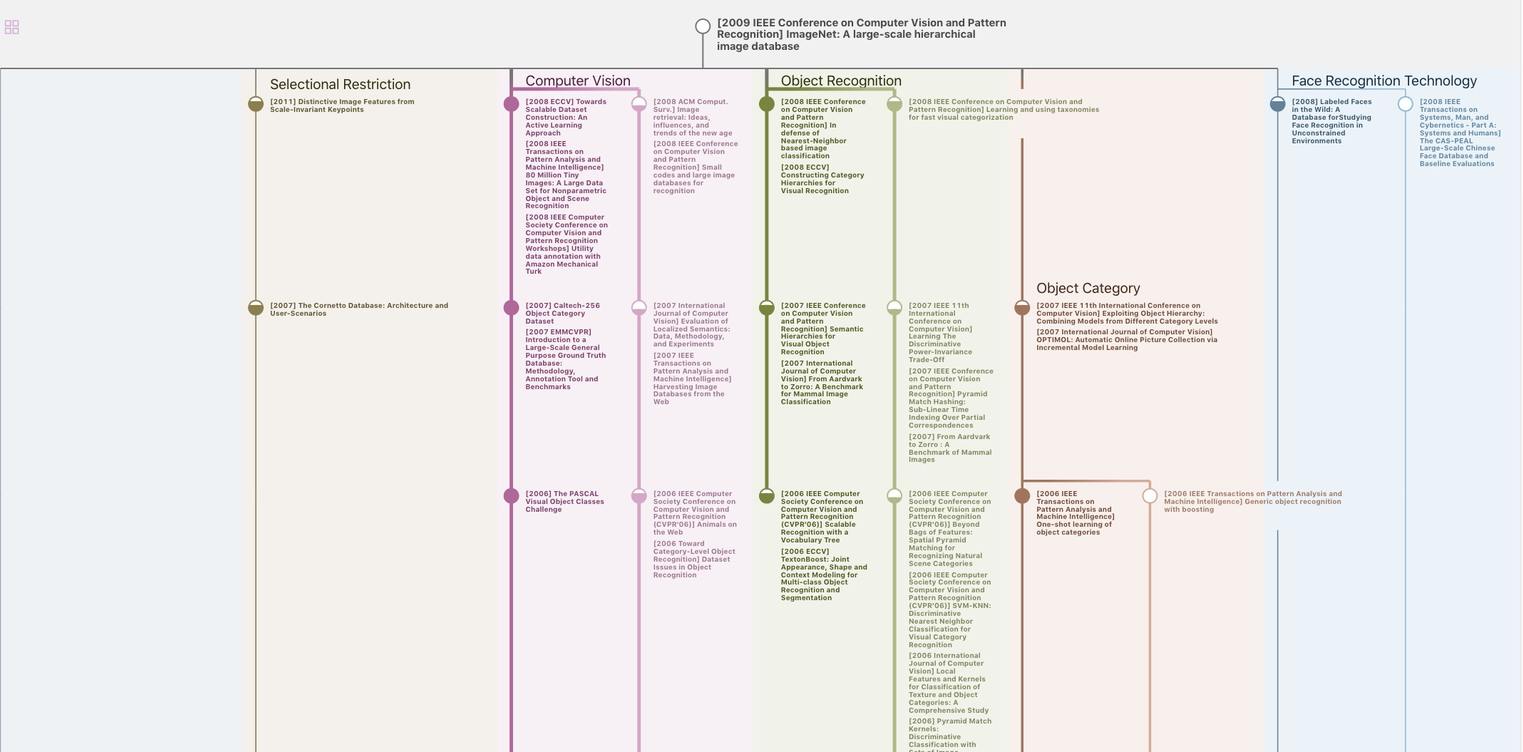
生成溯源树,研究论文发展脉络
Chat Paper
正在生成论文摘要