Who’s the Fairest of Them All? A Comparison of Methods for Classifying Tone and Causal Reasoning in Earnings-related Management Discourse
SSRN Electronic Journal(2020)
摘要
We compare the performance of machine learning algorithms and wordlists at replicating manual coding of sentence-level tone and attribution in earnings press releases. We train learning algorithms on a sample of manually annotated performance sentences and assess accuracy using a separate manually annotated holdout sample. Key findings are as follows. All methods detect negative sentences with lower accuracy than positive sentences. None of the approaches detect the presence of causal reasoning with high accuracy. Conditional on identifying a causal reasoning sentence manually, learning algorithms (but not wordlists) are able to distinguish between internal and external attributions. Absolute measurement errors exceed 20% for even the most reliable classification tasks such as tone and attribution type. No approach displays superior performance across all classification tasks and Naïve Bayes consistently underperforms other algorithms. Finally, even the best performing combination of classifiers struggles to detect self-attribution bias that is clearly evident with manual coding. We conclude that big data methods are not necessarily best for analyzing financial discourse, and that the value of manual coding should not be underestimated.
更多查看译文
AI 理解论文
溯源树
样例
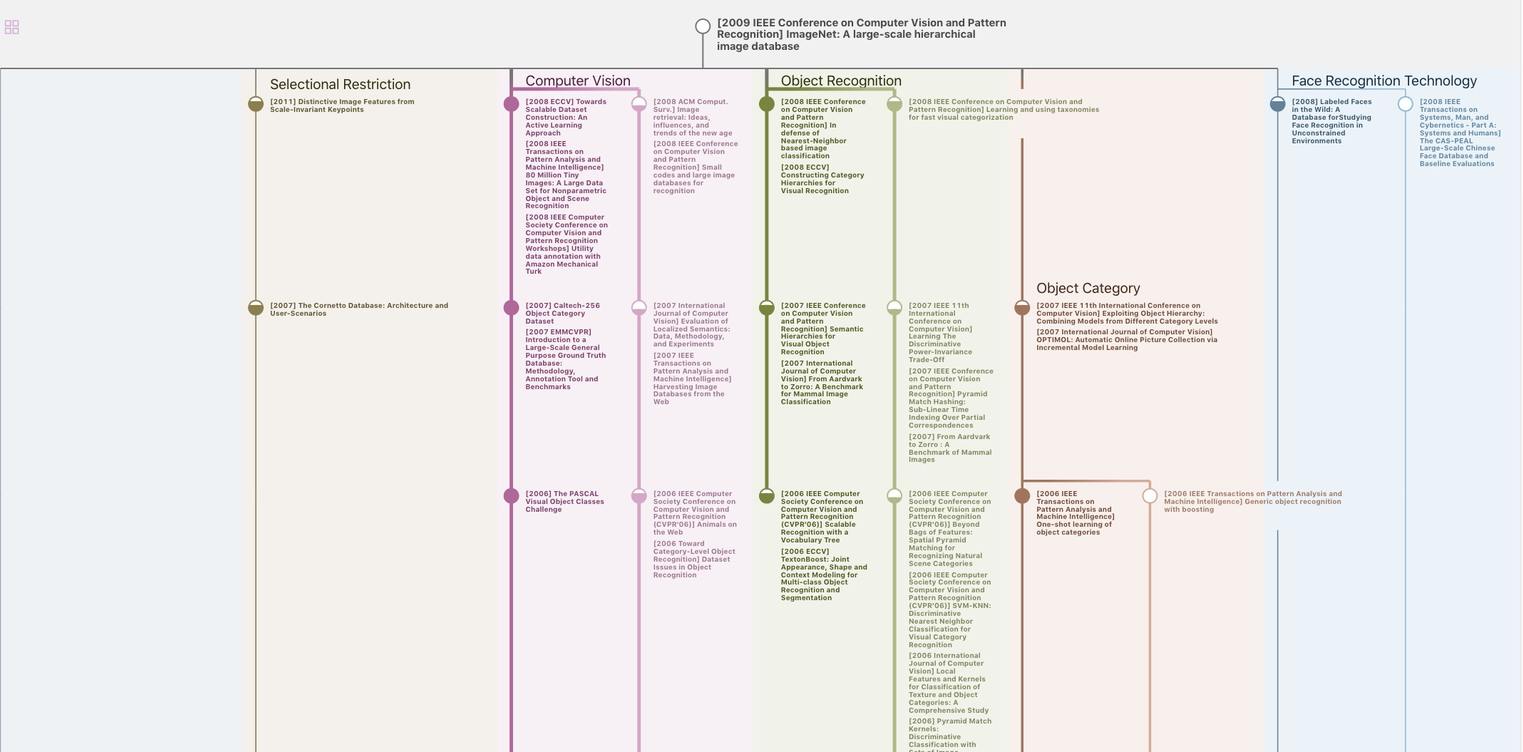
生成溯源树,研究论文发展脉络
Chat Paper
正在生成论文摘要