A Shift-Based Data Augmentation Strategy for Improving Saccade Landing Point Prediction
ETRA '20: 2020 Symposium on Eye Tracking Research and Applications Stuttgart Germany June, 2020(2020)
摘要
This paper demonstrates a technique for improving the performance of neural network-based models for saccade landing point estimation. Performance improvement is achieved by augmenting available training data with time-shifted replicates in order to improve prediction robustness to variations in saccade onset timing. The technique is validated for both long short-term memory (LSTM) and feed-forward neural network models for 5,893 saccades extracted from the recordings of 322 individuals during free-viewing of a movie trailer. The proposed augmentation strategy is demonstrated to improve the median accuracy of landing point estimates for LSTM models formulated using both the raw position and relative displacement of the gaze location.
更多查看译文
AI 理解论文
溯源树
样例
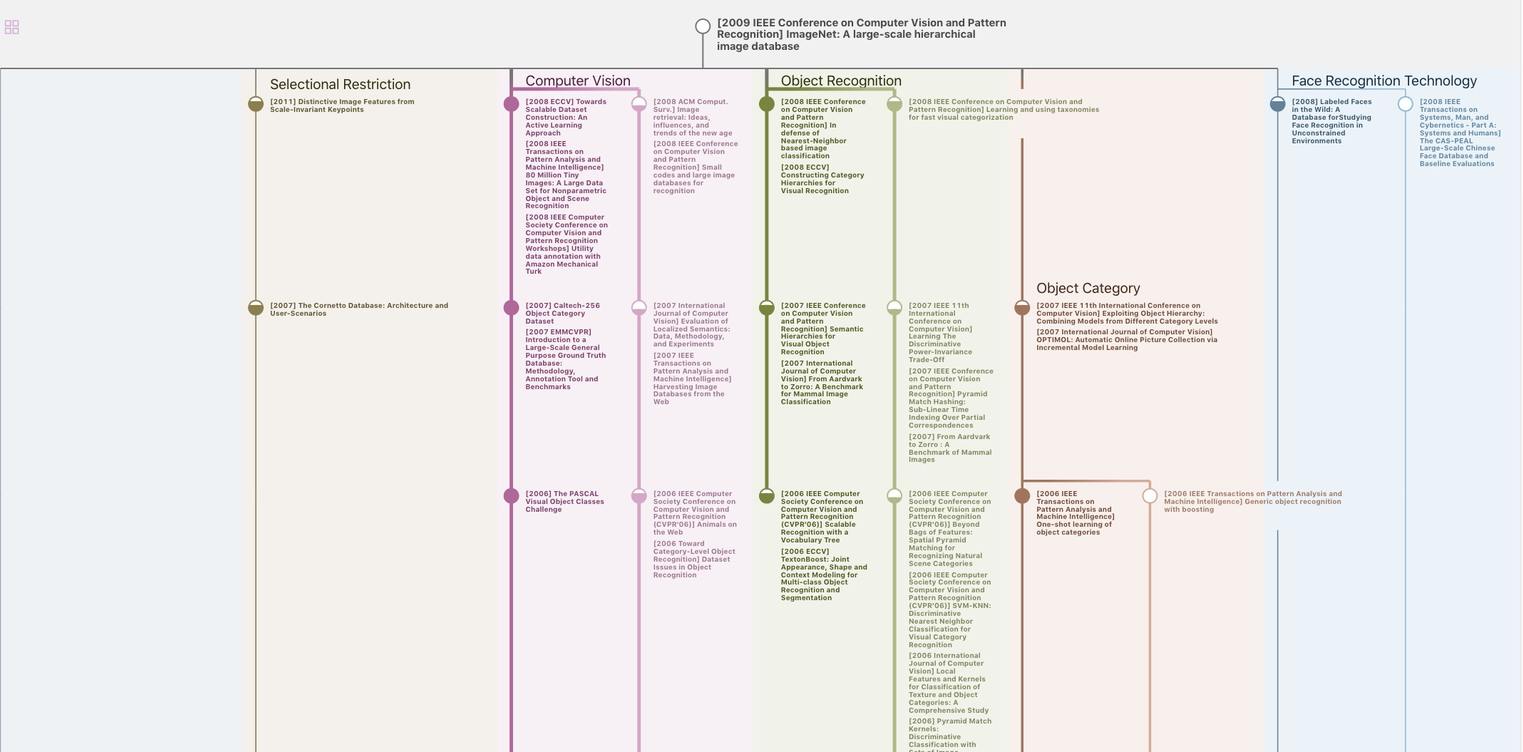
生成溯源树,研究论文发展脉络
Chat Paper
正在生成论文摘要