A Hierarchical Field-Level Inference Approach To Reconstruction From Sparse Lyman-Alpha Forest Data
arxiv(2020)
摘要
We address the problem of inferring the three-dimensional matter distribution from a sparse set of one-dimensional quasar absorption spectra of the Lyman-alpha forest. Using a Bayesian forward modelling approach, we focus on extending the dynamical model to a fully self-consistent hierarchical field-level prediction of redshift-space quasar absorption sightlines. Our field-level approach rests on a recently developed semiclassical analogue to Lagrangian perturbation theory (LPT), which improves over noise problems and interpolation requirements of LPT. It furthermore allows for a manifestly conservative mapping of the optical depth to redshift space. In addition, this new dynamical model naturally introduces a coarse-graining scale, which we exploited to accelerate the Markov chain Monte-Carlo (MCMC) sampler using simulated annealing. By gradually reducing the effective temperature of the forward model, we were able to allow it to first converge on large spatial scales before the sampler became sensitive to the increasingly larger space of smaller scales. We demonstrate the advantages, in terms of speed and noise properties, of this field-level approach over using LPT as a forward model, and, using mock data, we validated its performance to reconstruct three-dimensional primordial perturbations and matter distribution from sparse quasar sightlines.
更多查看译文
关键词
large-scale structure of Universe, dark matter, methods: statistical, methods: data analysis
AI 理解论文
溯源树
样例
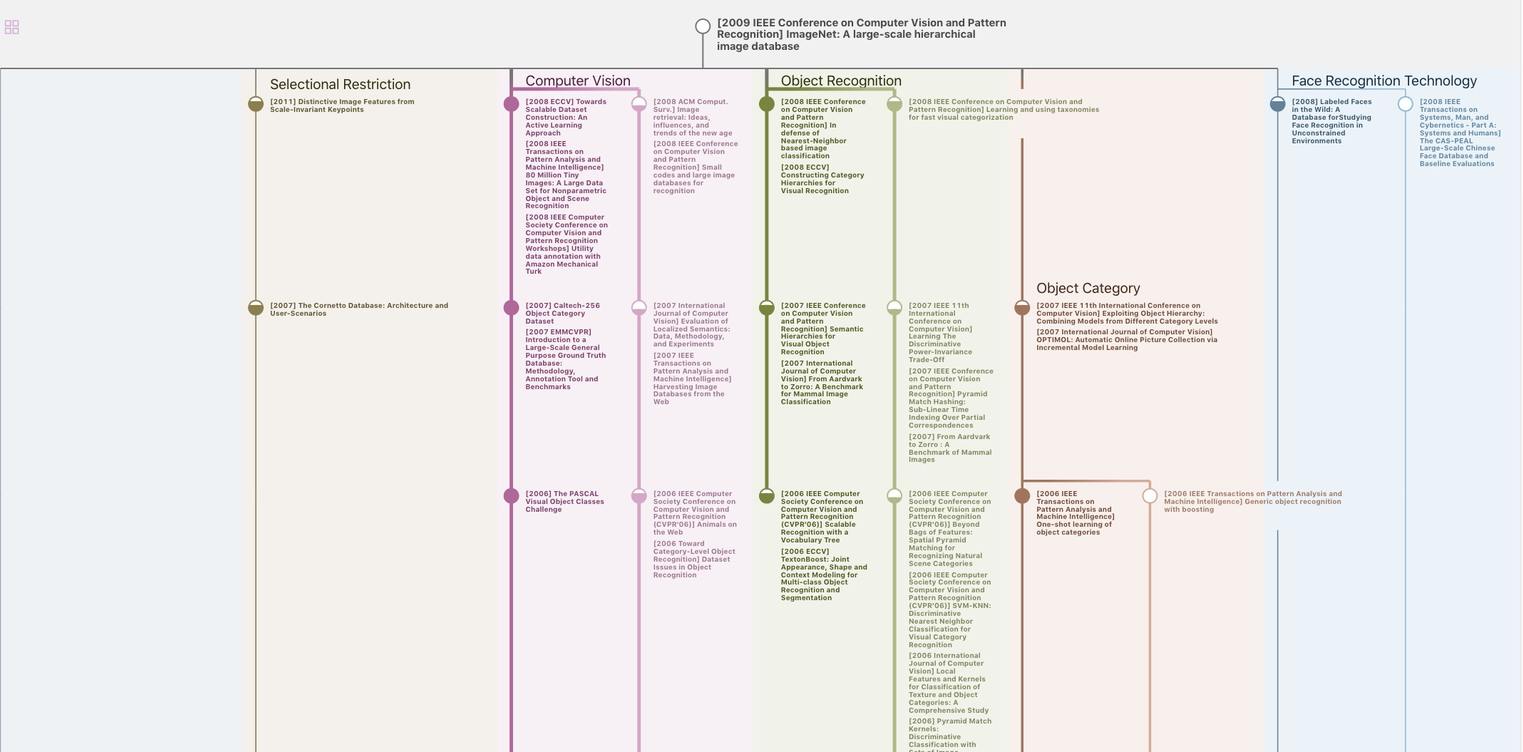
生成溯源树,研究论文发展脉络
Chat Paper
正在生成论文摘要