Predictive Modeling of Periodic Behavior for Human-Robot Symbiotic Walking
ICRA(2020)
摘要
We propose in this paper Periodic Interaction Primitives - a probabilistic framework that can be used to learn compact models of periodic behavior. Our approach extends existing formulations of Interaction Primitives to periodic movement regimes, i.e., walking. We show that this model is particularly well-suited for learning data-driven, customized models of human walking, which can then be used for generating predictions over future states or for inferring latent, biomechanical variables. We also demonstrate how the same framework can be used to learn controllers for a robotic prosthesis using an imitation learning approach. Results in experiments with human participants indicate that Periodic Interaction Primitives efficiently generate predictions and ankle angle control signals for a robotic prosthetic ankle, with MAE of 2.21 degrees in 0.0008s per inference. Performance degrades gracefully in the presence of noise or sensor fall outs. Compared to alternatives, this algorithm functions 20 times faster and performed 4.5 times more accurately on test subjects.
更多查看译文
关键词
human-robot symbiotic walking,probabilistic framework,periodic behavior,periodic movement regimes,customized models,human walking,latent variables,biomechanical variables,robotic prosthesis,imitation learning approach,human participants,ankle angle control signals,robotic prosthetic ankle,predictive modeling,Periodic Interaction Primitives
AI 理解论文
溯源树
样例
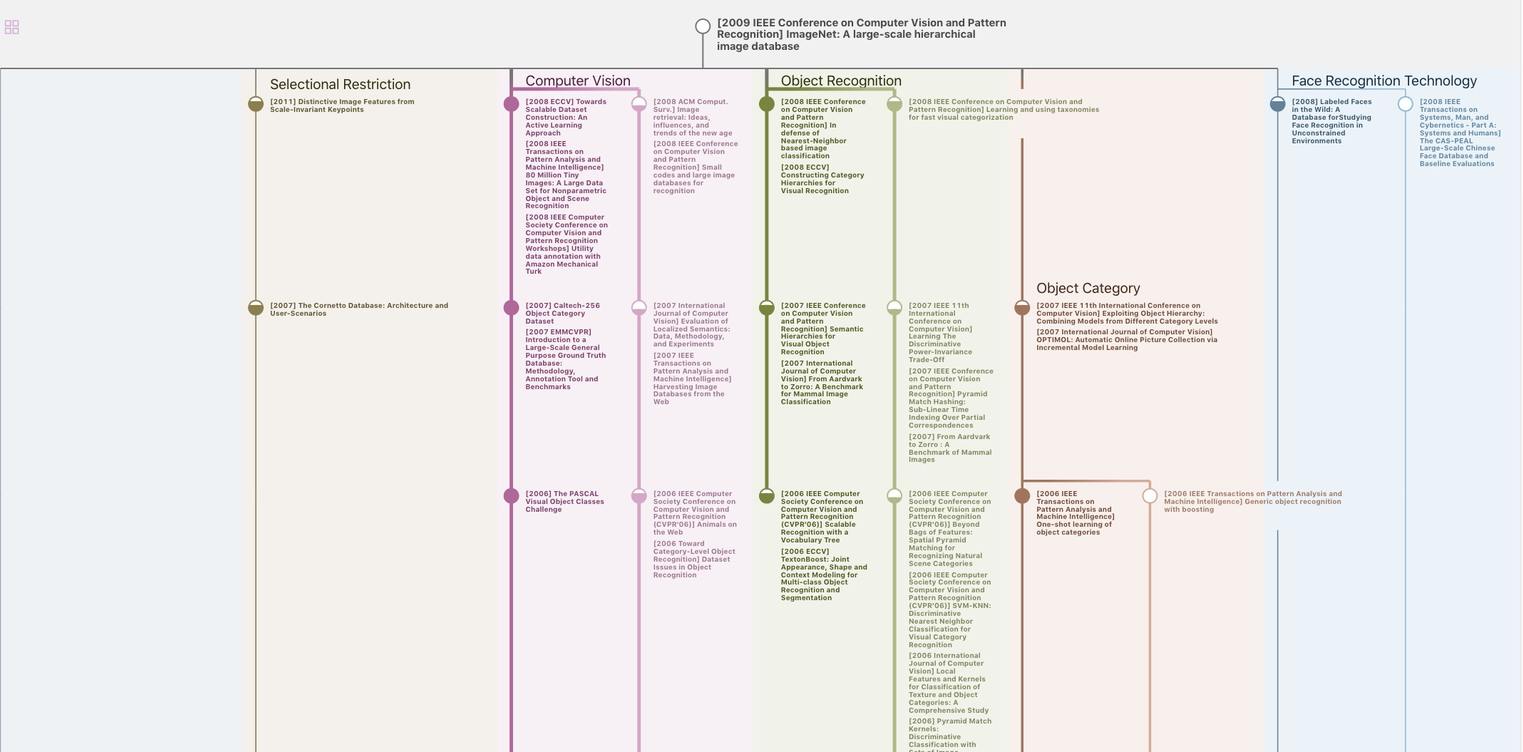
生成溯源树,研究论文发展脉络
Chat Paper
正在生成论文摘要