Nonmyopic and pseudo-nonmyopic approaches to optimal sequential design in the presence of covariates
arxiv(2023)
摘要
In sequential experiments, subjects become available for the study over a period of time, and covariates are often measured at the time of arrival. We consider the setting where sample size is fixed but covariate values are unknown until subjects enrol. Given a model for the outcome, a sequential optimal design approach can be used to allocate treatments to minimize the variance of the estimator of the treatment effect. We extend existing optimal design methodology so it can be used within a nonmyopic framework, where treatment allocation for the current subject depends not only on the treatments and covariates of the subjects already enrolled in the study, but also the impact of possible future treatment assignments within a specified horizon. The nonmyopic approach requires recursive formulae and suffers from the curse of dimensionality. We propose a pseudo-nonmyopic approach which has a similar aim to the nonmyopic approach, but does not involve recursion and instead relies on simulating trajectories of future possible decisions. Our simulation studies show that for the simple case of a logistic regression with a single binary covariate and a binary treatment, and a more realistic case with four binary covariates, binary treatment and treatment-covariate interactions, the nonmyopic and pseudo-nonmyopic approaches provide no competitive advantage over the myopic approach, both in terms of the size of the estimated treatment effect and also the efficiency of the designs. Results are robust to the size of the horizon used in the nonmyopic approach, and the number of simulated trajectories used in the pseudo-nonmyopic approach.
更多查看译文
关键词
Design of experiments,optimal design,dynamic programming,sequential design,coordinate exchange
AI 理解论文
溯源树
样例
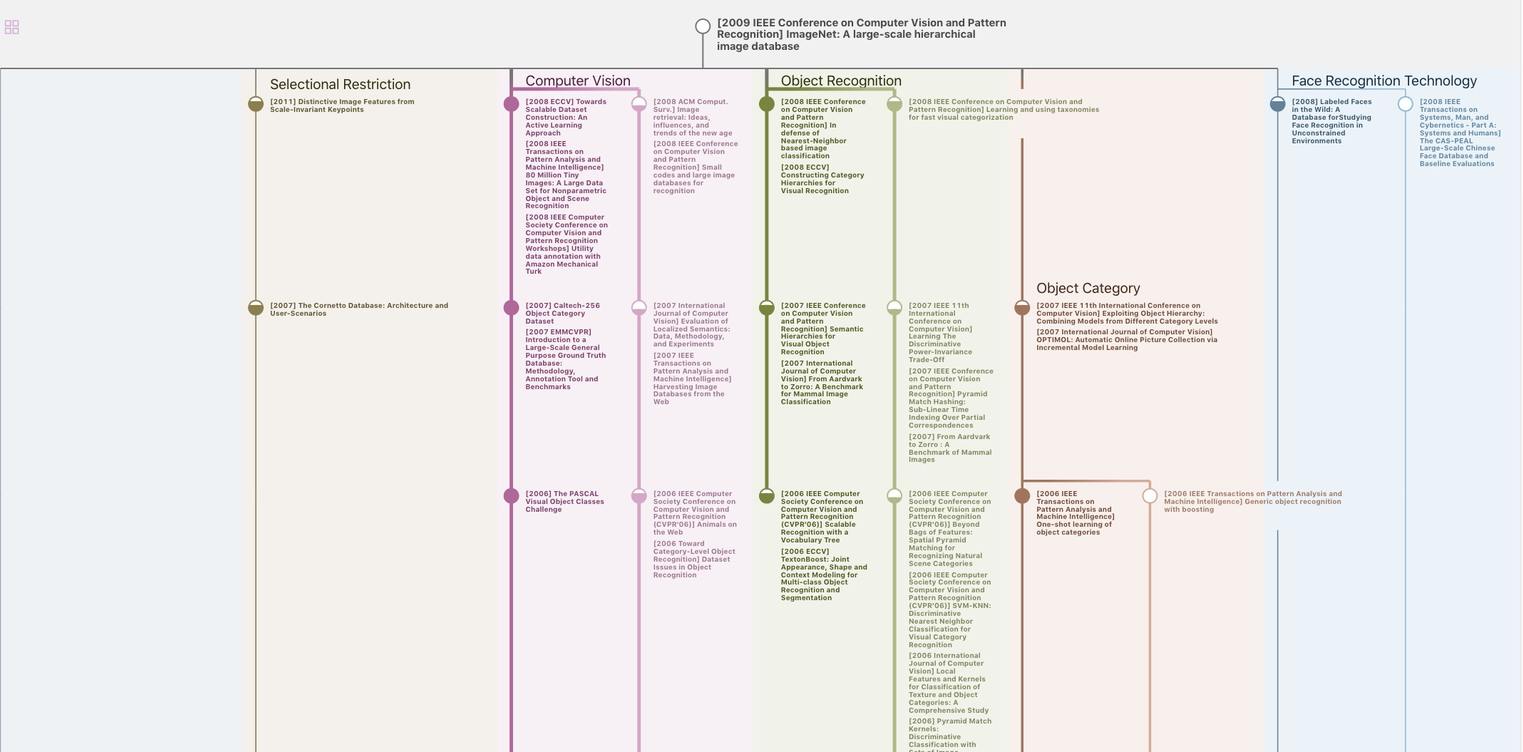
生成溯源树,研究论文发展脉络
Chat Paper
正在生成论文摘要