Uncertainty-Aware Blind Image Quality Assessment In The Laboratory And Wild
IEEE TRANSACTIONS ON IMAGE PROCESSING(2021)
摘要
Performance of blind image quality assessment (BIQA) models has been significantly boosted by end-to-end optimization of feature engineering and quality regression. Nevertheless, due to the distributional shift between images simulated in the laboratory and captured in the wild, models trained on databases with synthetic distortions remain particularly weak at handling realistic distortions (and vice versa). To confront the cross-distortion-scenario challenge, we develop a unified BIQA model and an approach of training it for both synthetic and realistic distortions. We first sample pairs of images from individual IQA databases, and compute a probability that the first image of each pair is of higher quality. We then employ the fidelity loss to optimize a deep neural network for BIQA over a large number of such image pairs. We also explicitly enforce a hinge constraint to regularize uncertainty estimation during optimization. Extensive experiments on six IQA databases show the promise of the learned method in blindly assessing image quality in the laboratory and wild. In addition, we demonstrate the universality of the proposed training strategy by using it to improve existing BIQA models.
更多查看译文
关键词
Databases, Distortion, Training, Uncertainty, Predictive models, Image quality, Optimization, Blind image quality assessment, learning-to-rank, uncertainty estimation, gMAD competition
AI 理解论文
溯源树
样例
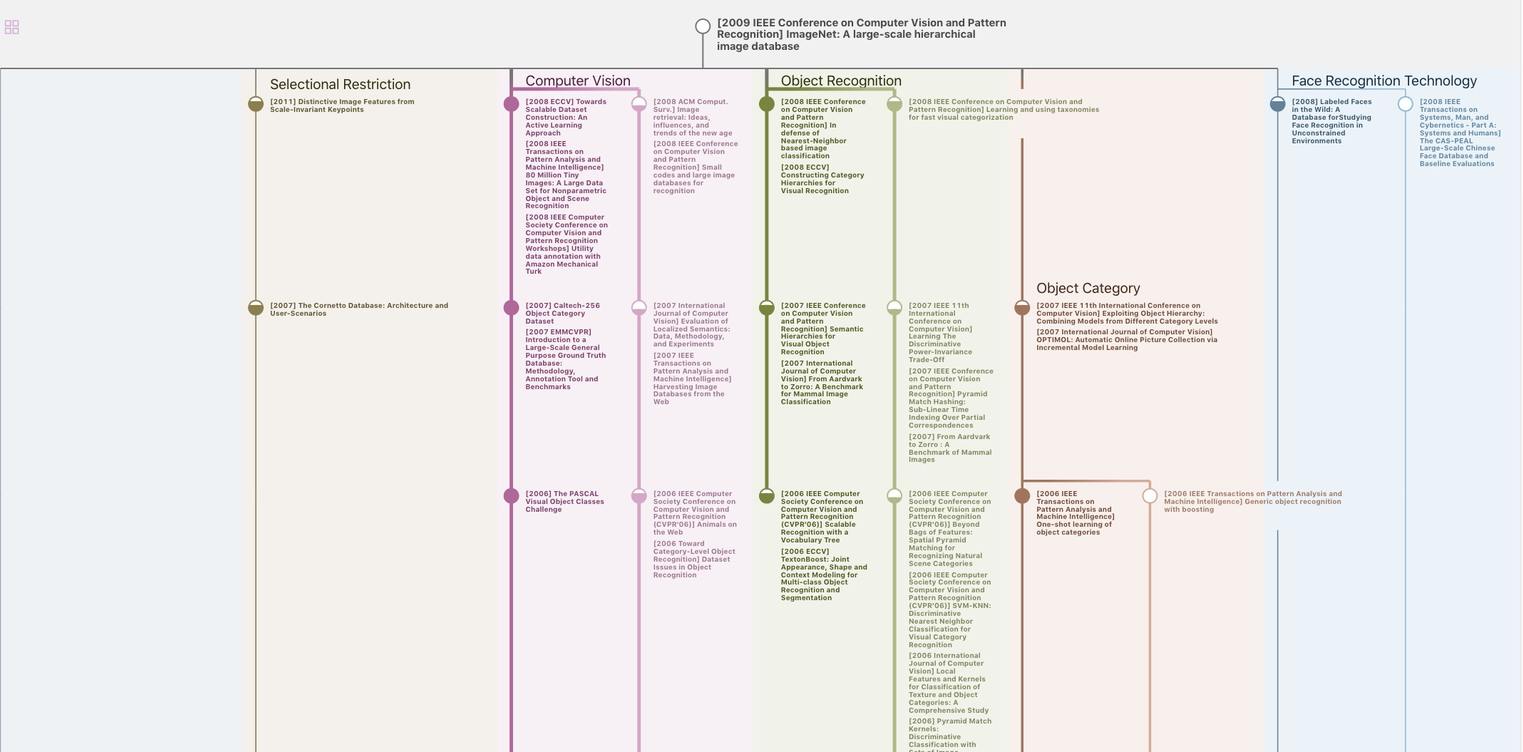
生成溯源树,研究论文发展脉络
Chat Paper
正在生成论文摘要